Probabilistic Flooding Performance Analysis Exploiting Graph Spectra Properties
IEEE/ACM Transactions on Networking(2023)
摘要
Probabilistic flooding is an efficient information dissemination policy capable of spreading information to the network nodes by sending information messages according to a fixed forwarding probability in a per-hop manner starting from an initiator node. It is a suitable approach, especially in topologies where the number of information messages sent under traditional approaches is significantly increased. The analysis presented in this paper considers graph spectra properties such as the largest eigenvalue
$\lambda _{1}$
of the adjacency matrix, and the eigenvector centrality. Both are analytically investigated and
$\frac {4}{\lambda _{1}}$
is derived as a lower bound of the forwarding probability that allows for global coverage, i.e., all network nodes receive the information message, under certain conditions also investigated here (e.g., the condition of the binomial approximation). It is shown that for any value of the forwarding probability equal to or larger than
$\frac {4}{\lambda _{1}}$
: (i) coverage is proportional to the initiator node’s eigenvector centrality; (ii) the probability a node receives the information message is proportional to the node’s eigenvector centrality; (iii) termination time decreases as the initiator node’s eigenvector centrality increases. If knowledge of
$\lambda _{1}$
is not available, then the average node degree
$\bar {d}$
can be used for ensuring global coverage. If knowledge of both
$\lambda _{1}$
and
$\bar {d}$
is not available, a dissemination policy is proposed that forwards messages to
$m$
(randomly selected) neighbor nodes. It is analytically shown that any value of
$m \geq 4$
allows for global coverage. Simulation results demonstrate the effectiveness of the considered analytical approach and the introduced policy.
更多查看译文
关键词
Information dissemination,coverage,termination time,probabilistic flooding,eigenvector centrality,largest eigenvalue,graph spectra properties,forwarding probability
AI 理解论文
溯源树
样例
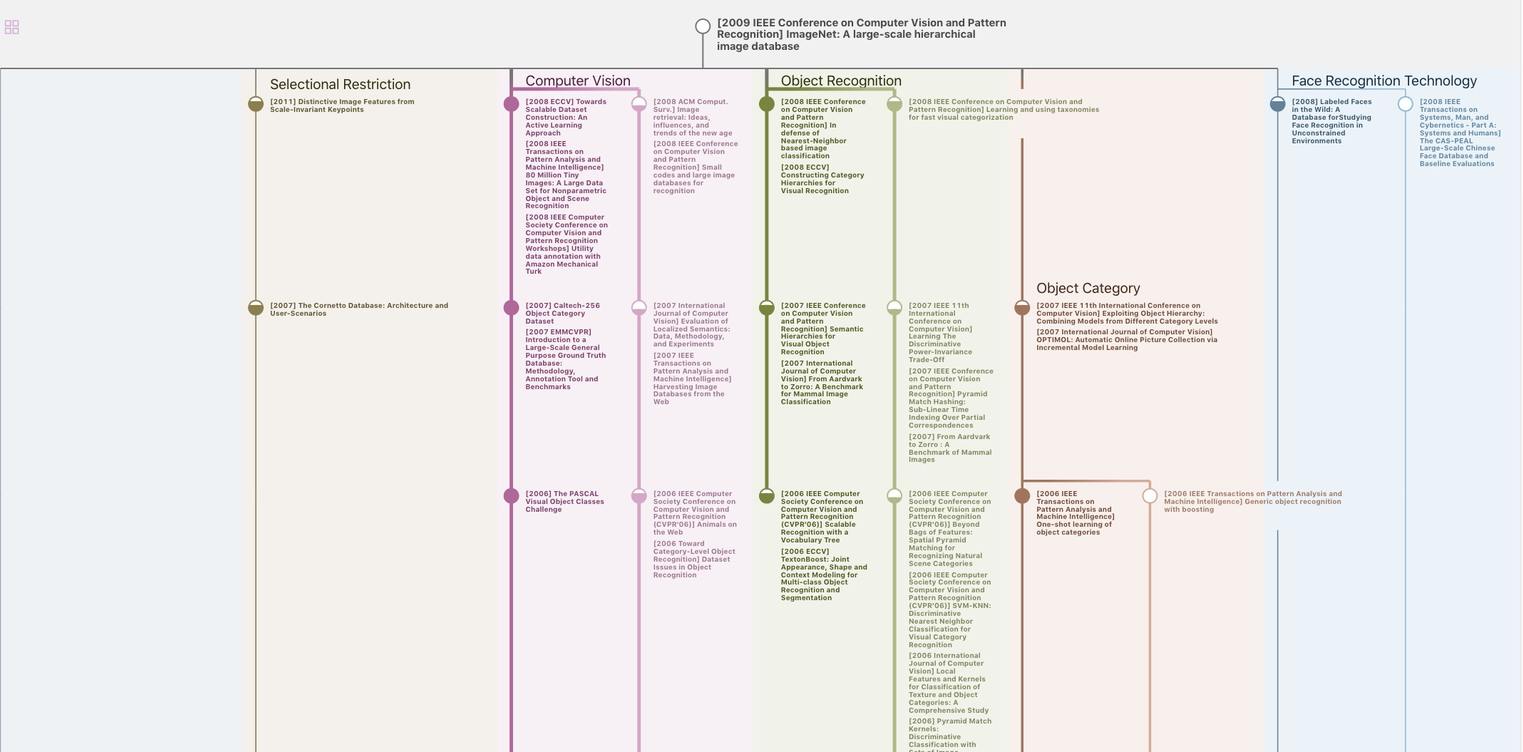
生成溯源树,研究论文发展脉络
Chat Paper
正在生成论文摘要