Navigating Diverse Salient Features for Vehicle Re-Identification
IEEE TRANSACTIONS ON INTELLIGENT TRANSPORTATION SYSTEMS(2022)
摘要
Mining sufficient discriminative information is vital for effective feature representation in vehicle re-identification. Traditional methods mainly focus on the most salient features and neglect whether the explored information is sufficient. This paper tackles the above limitation by proposing a novel Salience-Navigated Vehicle Re-identification Network (SVRN) which explores diverse salient features at multi-scales. For mining sufficient salient features, we design SVRN from two aspects: 1) network architecture: we propose a novel salience-navigated vehicle re-identification network, which mines diverse features under a cascaded suppress-and-explore mode. 2) feature space: cross-space constraint enables the diversity from feature space, which restrains the cross-space features by vehicle and image identifications (IDs). Extensive experiments demonstrate our method's effectiveness, and the overall results surpass all previous state-of-the-arts in three widely-used Vehicle ReID benchmarks (VeRi-776, VehicleID, and VERI-WILD), i.e., we achieve an 84.5% mAP on VeRi-776 benchmark that outperforms the second-best method by a large margin (3.5% mAP).
更多查看译文
关键词
Navigation, Task analysis, Image color analysis, Boosting, Feature extraction, Benchmark testing, Space vehicles, Vehicle re-identification, suppress-and-explore mode, grid-based salient navigation, cross-space constraints
AI 理解论文
溯源树
样例
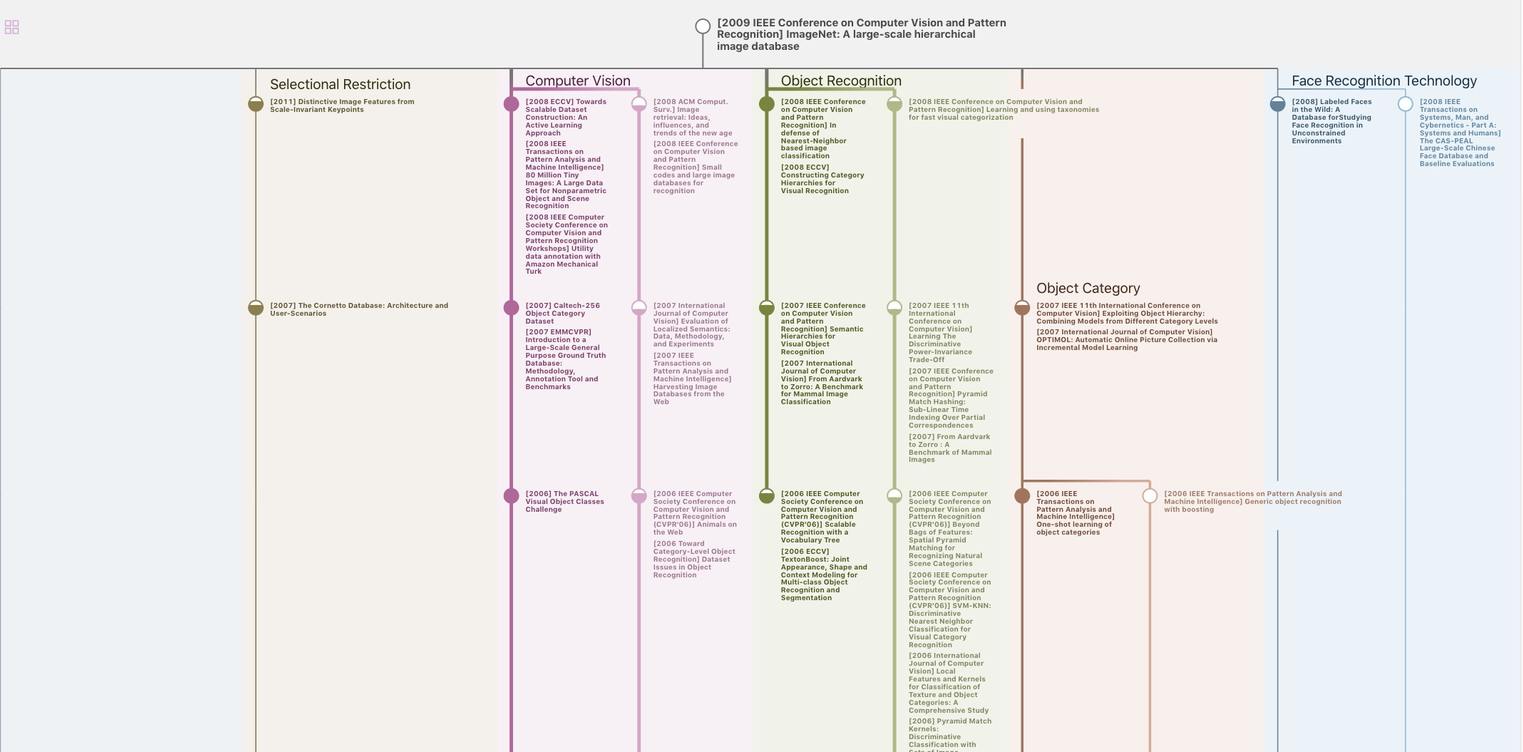
生成溯源树,研究论文发展脉络
Chat Paper
正在生成论文摘要