Global Prescribed Performance Control for Strict Feedback Systems Pursuing Uncertain Target
IEEE TRANSACTIONS ON NEURAL NETWORKS AND LEARNING SYSTEMS(2024)
摘要
In this work, an online solution for reconstructing and predicting the uncertain target trajectory in real-time is proposed based on general regression neural network (GRNN). On this basis, an adaptive tracking control scheme guaranteeing prescribed performance is suggested for a class of strict-feedback systems with unknown control directions. In contrast to existing trajectory reconstruction methods, the one presented in this note does not require prior modeling of the uncertain target or offline training. Contrary to most current state-of-the-art prescribed performance control (PPC) technology, a novel time-varying scaling function and its corresponding translation function are introduced such that no strict constraints on initial conditions are needed, that is, global stability is achieved. The proposed control scheme allows the output of the system to chase the predicted value of the uncertain target, and the tracking error converges to a prescribed small set within a preassigned time, despite unmatched uncertainties and unknown control directions. The benefits of the proposed control scheme are confirmed by numerical simulations.
更多查看译文
关键词
Trajectory,Target tracking,Real-time systems,Prediction algorithms,Noise measurement,Uncertainty,Monitoring,General regression neural network (GRNN),prescribed performance,uncertain target trajectory,unknown control directions,unmatched uncertainties
AI 理解论文
溯源树
样例
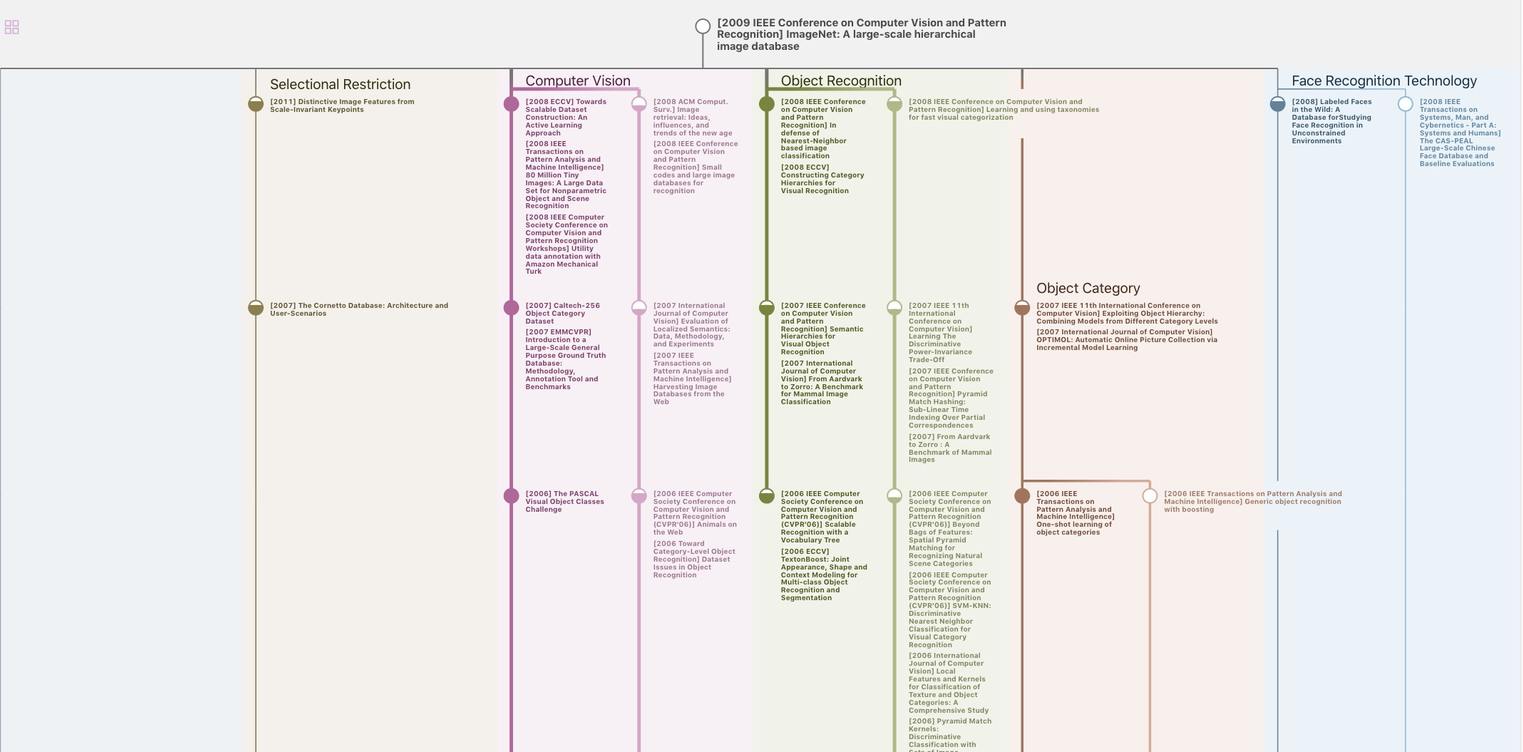
生成溯源树,研究论文发展脉络
Chat Paper
正在生成论文摘要