A new clustering method to explore the dynamics of research communities
Scientometrics(2022)
摘要
Description of temporal networks and detection of dynamic communities have been hot topics of research for the last decade. However, no consensual answers to these challenges have been found due to the complexity of the task. Static communities are not well defined objects, and adding a temporal dimension makes the description even more difficult. In this article, we propose a coherent temporal clustering method to explore the dynamics of research communities: the Best Combination of Local Communities (BCLC). Our method aims at finding a good balance between two contradictory objectives: closely following the short-term evolution by finding optimal partitions at each time step, on the one hand, and temporal smoothing, which privileges historical continuity, on the other hand. We test our algorithm on two bibliographic data sets by comparing their mesoscale dynamic description to those derived from a (static) simple clustering algorithm applied over the whole data set. We show that our clustering algorithm can reveal more complex dynamics than the simple approach and reach a good agreement with expert’s knowledge.
更多查看译文
关键词
Dynamic community detection, Research communities, Temporal networks, Bibliographic networks
AI 理解论文
溯源树
样例
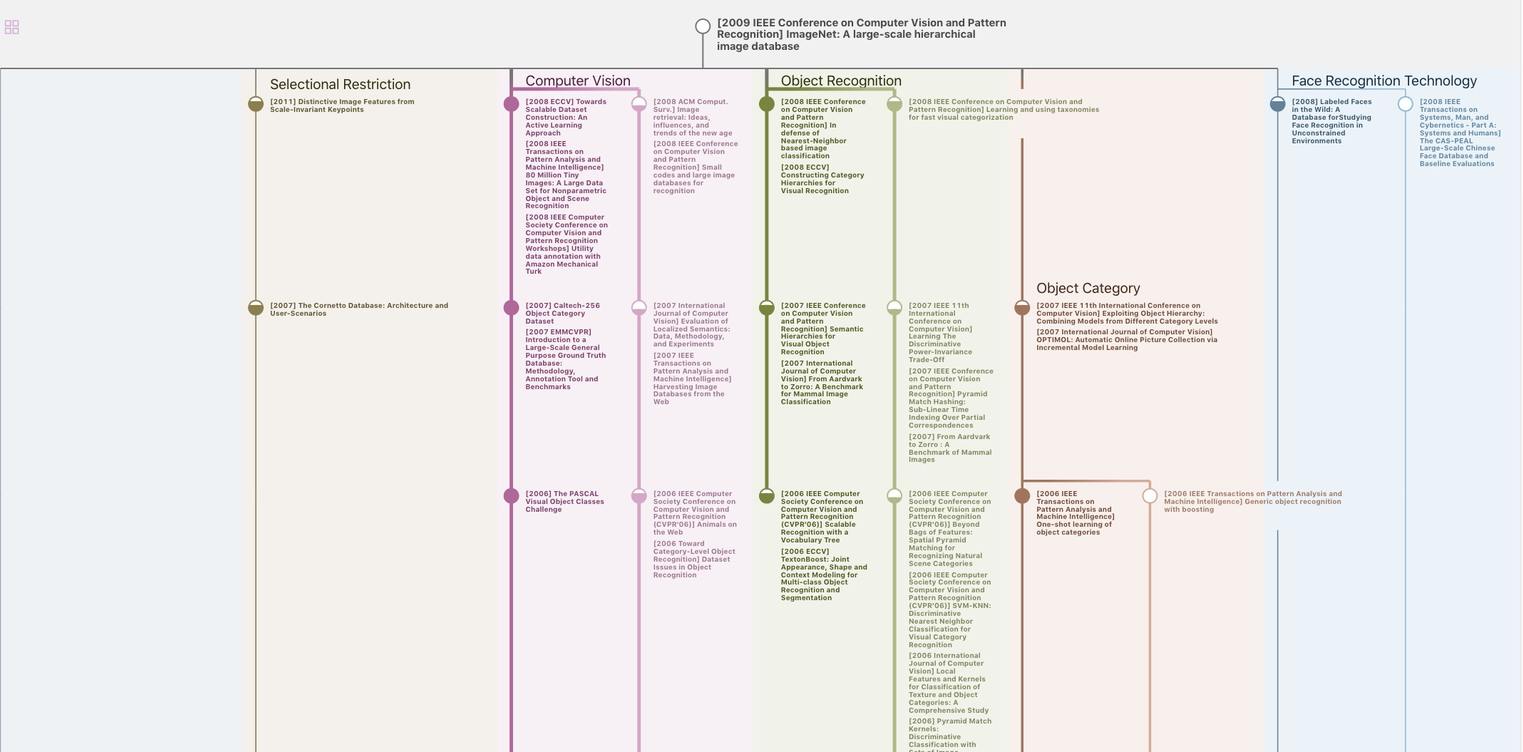
生成溯源树,研究论文发展脉络
Chat Paper
正在生成论文摘要