Combating Label Noise in Image Data Using MultiNET Flexible Confident Learning
APPLIED SCIENCES-BASEL(2022)
摘要
Deep neural networks (DNNs) have been used successfully for many image classification problems. One of the most important factors that determines the final efficiency of a DNN is the correct construction of the training set. Erroneously labeled training images can degrade the final accuracy and additionally lead to unpredictable model behavior, reducing reliability. In this paper, we propose MultiNET, a novel method for the automatic detection of noisy labels within image datasets. MultiNET is an adaptation of the current state-of-the-art confident learning method. In contrast to the original, our method aggregates the outputs of multiple DNNs and allows for the adjustment of detection sensitivity. We conduct an exhaustive evaluation, incorporating four widely used datasets (CIFAR10, CIFAR100, MNIST, and GTSRB), eight state-of-the-art DNN architectures, and a variety of noise scenarios. Our results demonstrate that MultiNET significantly outperforms the confident learning method.
更多查看译文
关键词
image classification, label noise, deep neural networks
AI 理解论文
溯源树
样例
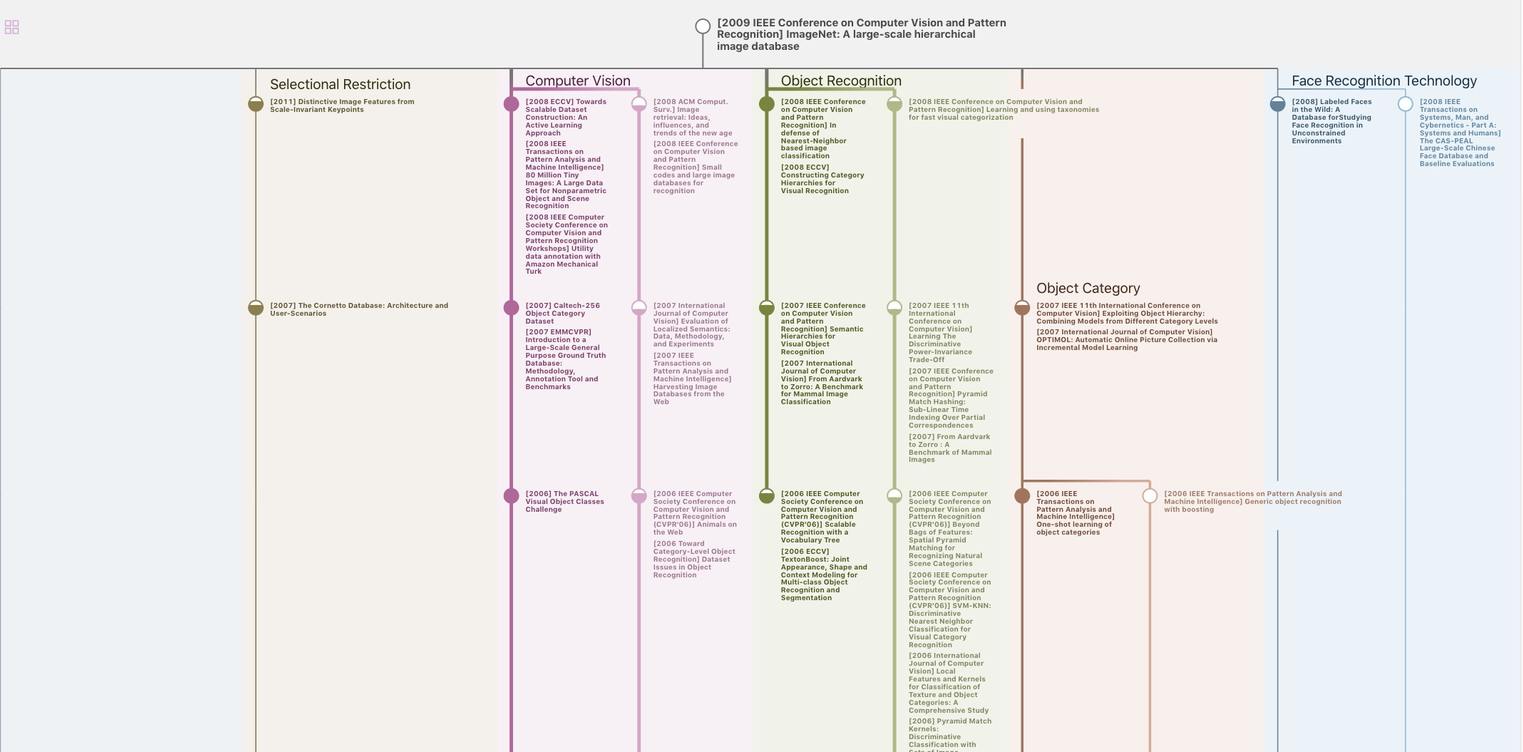
生成溯源树,研究论文发展脉络
Chat Paper
正在生成论文摘要