Employing a Neural Network Approach for Reducing the Convergence Speed of Diffuse Optical Image Reconstruction Algorithms
HIGH-SPEED BIOMEDICAL IMAGING AND SPECTROSCOPY VII(2022)
摘要
Time-domain tomographic image reconstruction is typically based on an iterative process that requires repeated solving of the forward model of time-dependent light propagation in tissue. As a result, image reconstruction times remain relatively high. This has been one of the main obstacles in the practical use of time-domain data, for example, for realtime monitoring of brain function, in which case results have to be displayed in less than a second. To overcome this problem, we have developed a neural-network-based approach that promises to deliver image reconstructions in the subseconds range. The inputs to this network are parameterized data derived from the Mellin and Laplace transforms of the time of flight (ToF) distribution. In this study, we specifically focused on three data types: the integrated intensity (E), the mean time of flight (), and the exponential feature (L). The network tested consisted of an input layer, three hidden layers, and an output layer that represents the spatial distribution of absorption values for the medium. We trained the parameters of the network with simulated brain diffuse optical tomography data. The inverse problem is then solved with a single-feed forward pass through the network. We demonstrate that this network, once trained, can recover single and multiple inclusions in a 3D medium with accurate localization within milliseconds and outperforms constrained iterative reconstruction methods.
更多查看译文
关键词
brain imaging, diffuse optical tomography, deep learning, neural networks
AI 理解论文
溯源树
样例
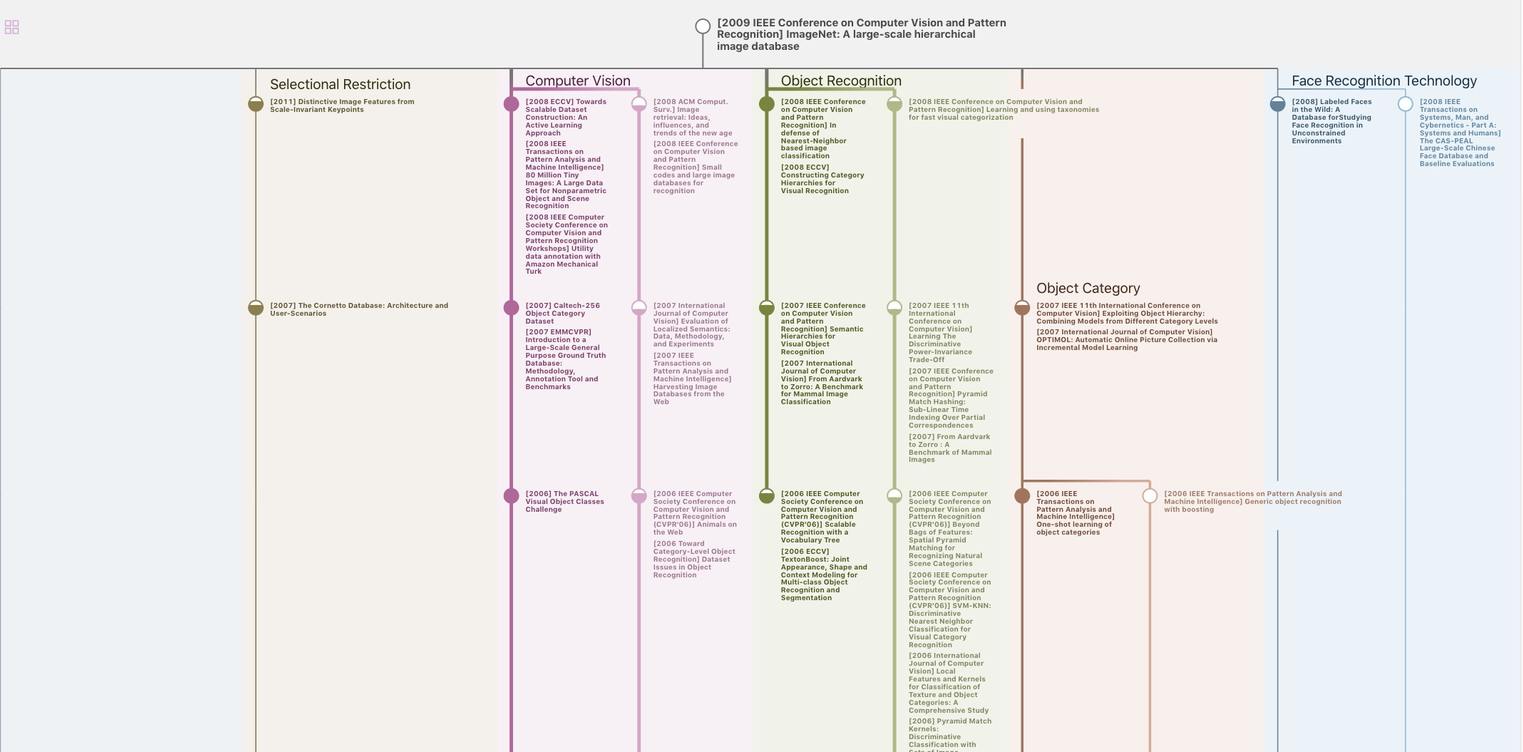
生成溯源树,研究论文发展脉络
Chat Paper
正在生成论文摘要