Deep Learning Based Low Complexity Symbol Detection and Modulation Classification Detector
IEICE TRANSACTIONS ON COMMUNICATIONS(2022)
摘要
This paper presents a deep neural network (DNN) based symbol detection and modulation classification detector (SDMCD) for mixed blind signals detection. Unlike conventional methods that employ symbol detection after modulation classification, the proposed SDMCD can perform symbol recovery and modulation identification simultaneously. A cumulant and moment feature vector is presented in conjunction with a low complexity sparse autoencoder architecture to complete mixed signals detection. Numerical results show that SDMCD scheme has remarkable symbol error rate performance and modulation classification accuracy for various modulation formats in AWGN and Rayleigh fading channels. Furthermore, the proposed detector has robust performance under the impact of frequency and phase offsets.
更多查看译文
关键词
symbol detection, modulation classification, deep neural network (DNN), multi-cumulant and moment features (MCMF), frequency and phase offsets
AI 理解论文
溯源树
样例
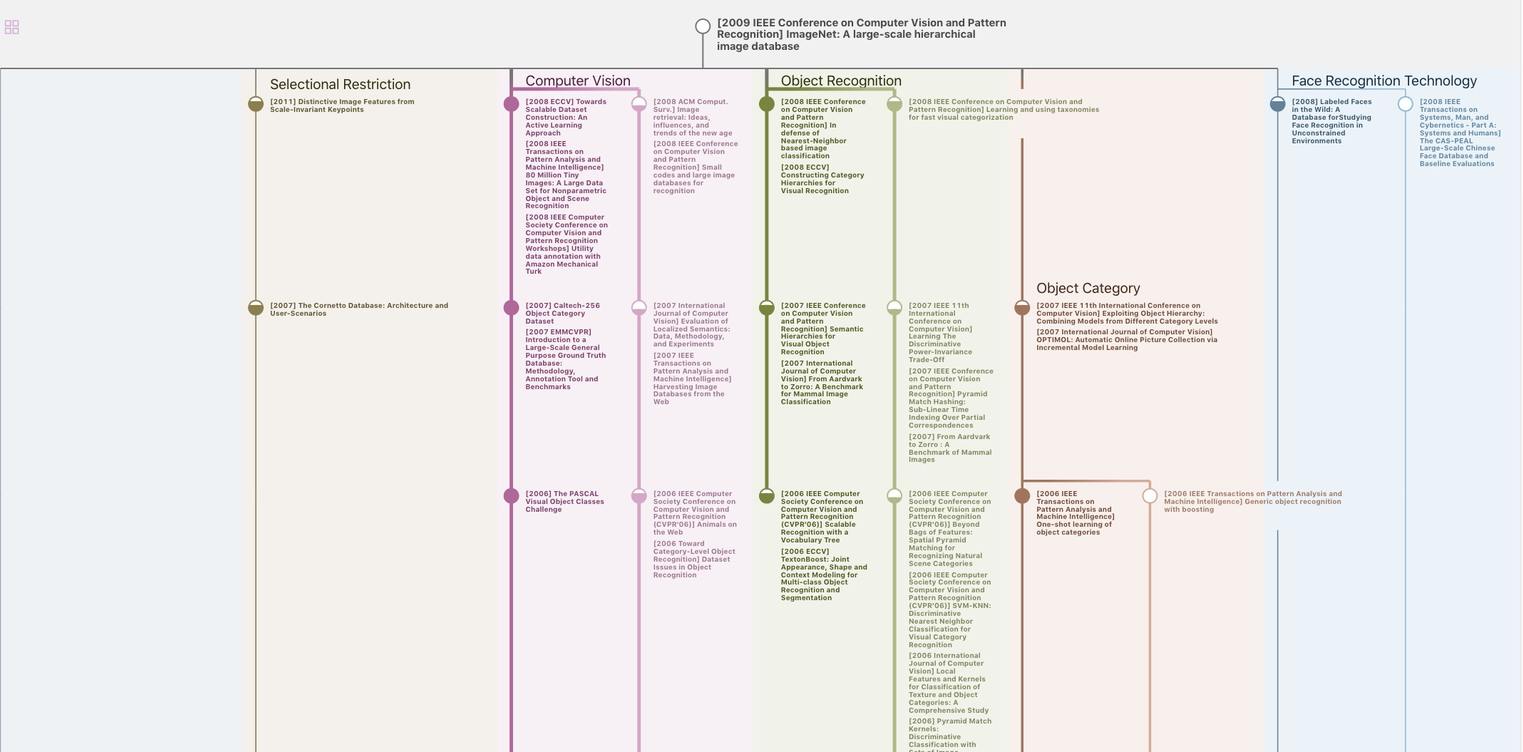
生成溯源树,研究论文发展脉络
Chat Paper
正在生成论文摘要