Self-Supervised Classification of Weather Systems Based on Spatiotemporal Contrastive Learning
GEOPHYSICAL RESEARCH LETTERS(2022)
摘要
The classification of weather systems provides a simple description of atmospheric circulations and bridges the gap between large-scale atmospheric conditions and local-scale environmental variables. However, the existing classification methods are challenged due to a lack of labels and inaccurate similarity measures between data samples. In this letter, we propose a self-supervised Spatiotemporal Contrastive Learning (SCL) framework for the classification of weather systems without manual labels. In particular, we operate both spatial and temporal augmentation on multivariate meteorological data to fully explore temporal context information and spatial stability in accordance with synoptic nature. For the assessment and comparison of the classification results, we apply a statistical method based on analog forecasting using the classification results as input. The experimental results demonstrate that the proposed SCL model outperforms traditional classification methods.
更多查看译文
关键词
weather system classifications, contrastive learning, CNNs, analog forecasting
AI 理解论文
溯源树
样例
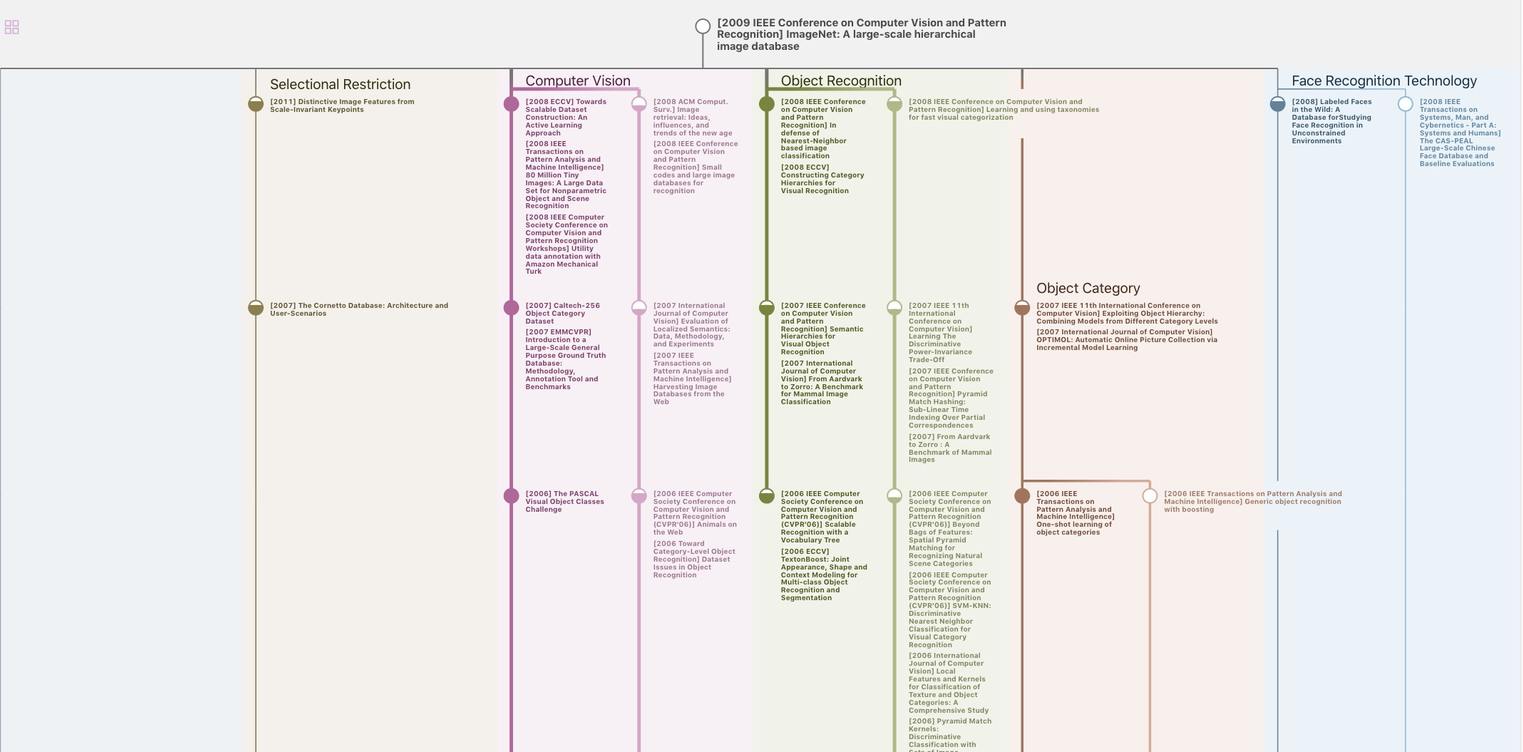
生成溯源树,研究论文发展脉络
Chat Paper
正在生成论文摘要