Knowledge Graph Recommendation Model Based on Adversarial Training
APPLIED SCIENCES-BASEL(2022)
摘要
The recommendation model based on the knowledge graph (KG) alleviates the problem of data sparsity in the recommendation to a certain extent and further improves the accuracy, diversity, and interpretability of recommendations. Therefore, the knowledge graph recommendation model has become a major research topic, and the question of how to utilize the entity and relation information fully and effectively in KG has become the focus of research. This paper proposes a knowledge graph recommendation model based on adversarial training (ATKGRM), which can dynamically and adaptively adjust the knowledge graph aggregation weight based on adversarial training to learn the features of users and items more reasonably. First, the generator adopts a novel long- and short-term interest model to obtain user features and item features and generates a high-quality set of candidate items. Then, the discriminator discriminates candidate items by comparing the user's scores of positive items, negative items, and candidate items. Finally, experimental studies on five real-world datasets with multiple knowledge graph recommendation models and multiple adversarial training recommendation models prove the effectiveness of our model.
更多查看译文
关键词
recommendation model, knowledge graph, adversarial training, self-attention mechanism
AI 理解论文
溯源树
样例
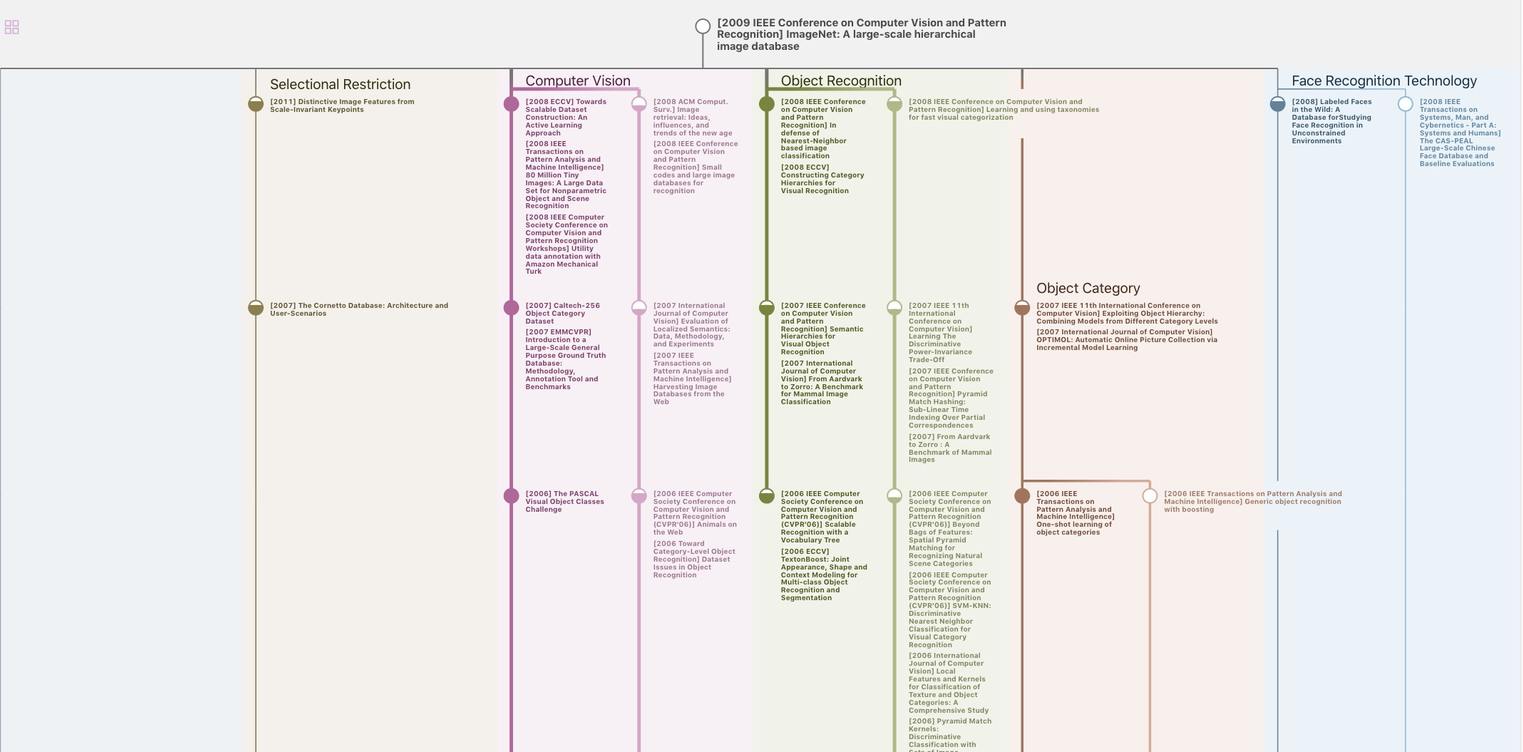
生成溯源树,研究论文发展脉络
Chat Paper
正在生成论文摘要