A Novel Minimization Approximation Cost Classification Method to Minimize Misclassification Rate for Dichotomous and Homogeneous Classes
RMS-RESEARCH IN MATHEMATICS & STATISTICS(2021)
摘要
Dependence of the linear discriminant analysis on location and scale weakens its performance when predicting class under the presence of homogeneous covariance matrices for the candidate classes. Further, outlying samples render the method to suffer from higher rates of misclassification. In this study, we propose the minimization approximation cost classification (MACC) method that accounts for some specific cost function 23.9. The theoretical derivation is made to find an optimal linear hyperplane theta, which yields maximum separation between the dichotomous groups. Real-life data and simulations were used to validate the method against the standard classifiers. Results show that the proposed method is more efficient and outperforms the standard methods when the data are crowded at the class boundaries.
更多查看译文
关键词
Linear discriminant analysis, majorization-minimization principle, misclassification rate, minimization approximation cost function, optimal linear hyperplane
AI 理解论文
溯源树
样例
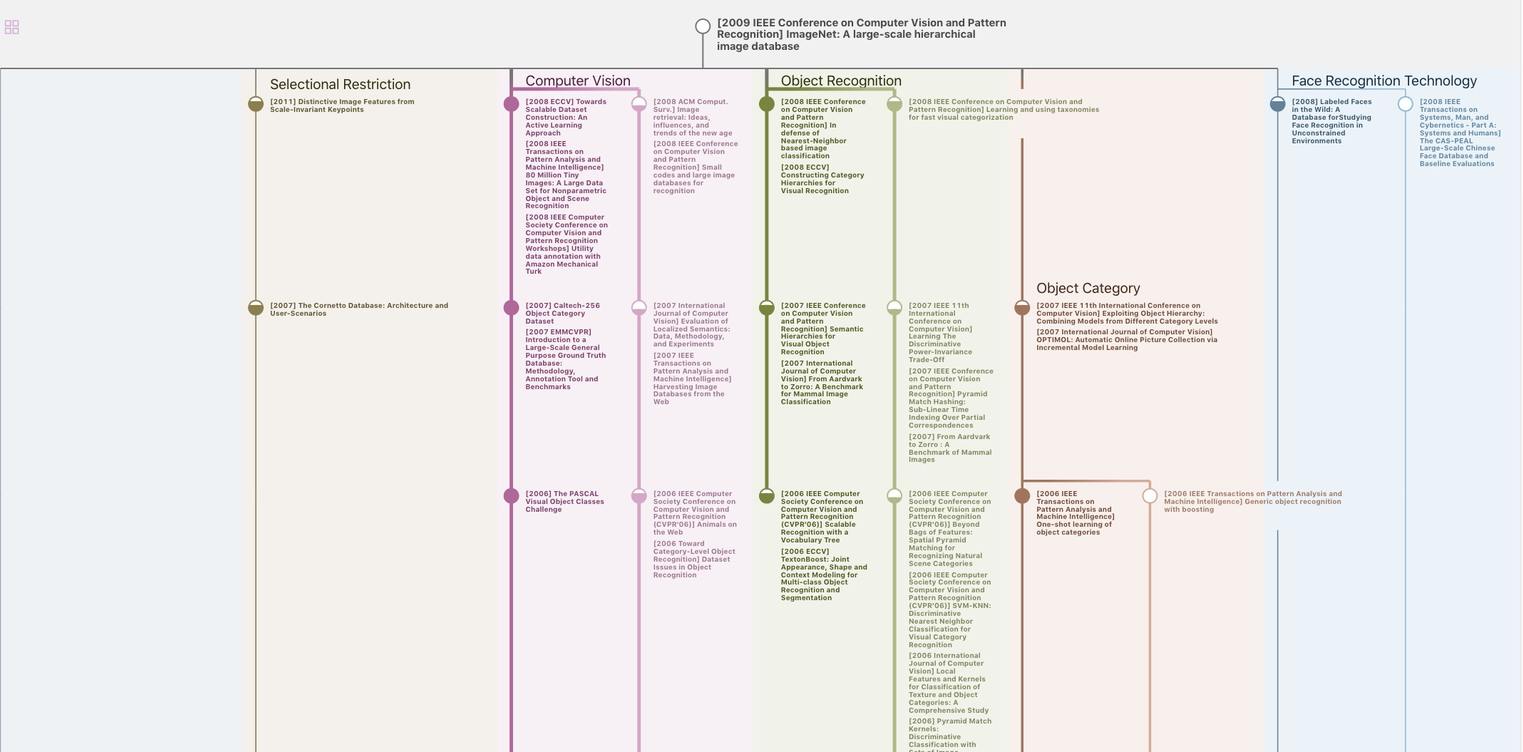
生成溯源树,研究论文发展脉络
Chat Paper
正在生成论文摘要