Coherent noise suppression in digital holographic microscopy based on label-free deep learning
FRONTIERS IN PHYSICS(2022)
摘要
Deep learning techniques can be introduced into the digital holography to suppress the coherent noise. It is often necessary to first make a dataset of noisy and noise-free phase images to train the network. However, noise-free images are often difficult to obtain in practical holographic applications. Here we propose a label-free training algorithms based on self-supervised learning. A dilated blind spot network is built to learn from the real noisy phase images and a noise level function network to estimate a noise level function. Then they are trained together via maximizing the constrained negative log-likelihood and Bayes' rule to generate a denoising phase image. The experimental results demonstrate that our method outperforms standard smoothing algorithms in accurately reconstructing the true phase image in digital holographic microscopy.
更多查看译文
关键词
digital holography, noise suppression, self-supervised learning, label-free, digital holographic microscopy (DHM)
AI 理解论文
溯源树
样例
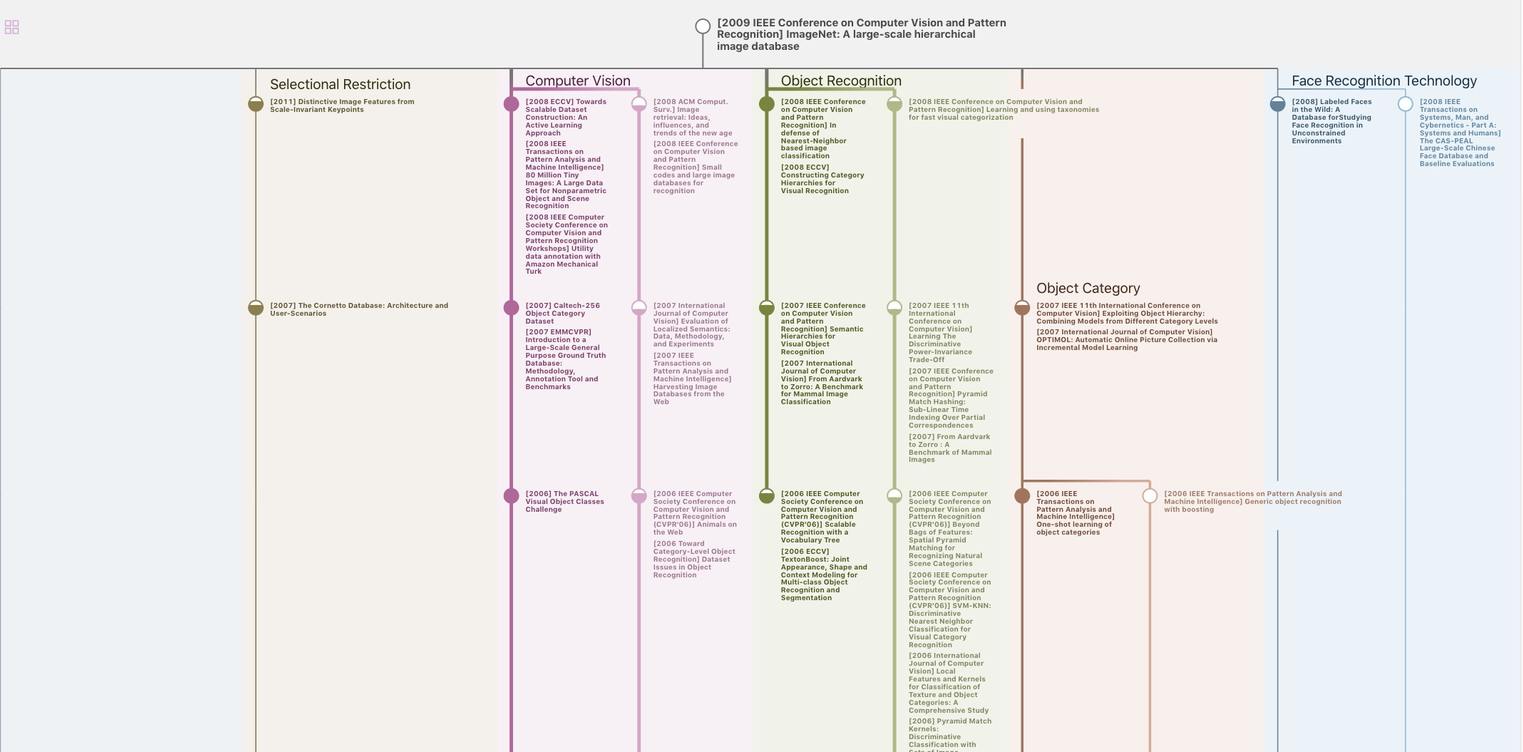
生成溯源树,研究论文发展脉络
Chat Paper
正在生成论文摘要