Screening interface passivation materials intelligently through machine learning for highly efficient perovskite solar cells
JOURNAL OF MATERIALS CHEMISTRY A(2022)
摘要
Intelligently screening passivation materials is critical for improving the power conversion efficiency (PCE) values of perovskite solar cells (PSCs), which are still lacking. Herein, machine learning is employed to map the correlations between the PCE and interface passivation material at the atomic level, enabling intelligent material screening. The dataset includes around 100 interface materials used at the perovskite/hole transport layer interface. The random forest model best predicts the PCE, with a root mean square error of 0.7%. High-throughput predictions are further made and rationalized using density functional theory calculations. It is revealed that a material with a high binding energy with the [PbI4](2-) surface promotes strong passivation effects, and small organic cations with an NH3+ terminal have high potential. Experimental validation using methylammonium iodide and phenethylammonium iodide as the interface materials reveals the reliability of the predictions. Our work enables the high-throughput and rapid screening/design of interface materials for highly efficient PSCs, and it also provides general screening rules for interface materials at the atomic level.
更多查看译文
AI 理解论文
溯源树
样例
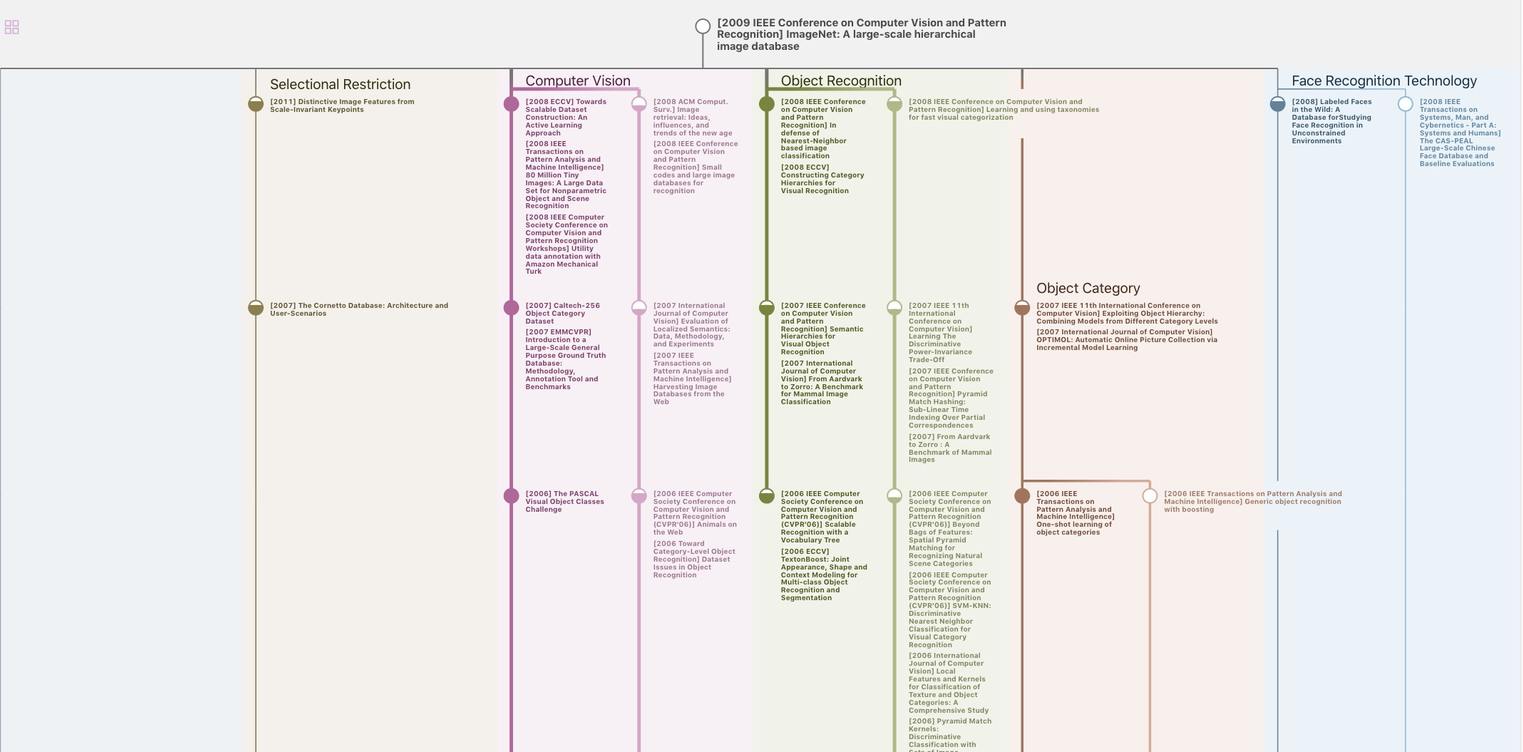
生成溯源树,研究论文发展脉络
Chat Paper
正在生成论文摘要