Estimation of Missing LiDAR Data for Accurate AGV Localization
IEEE ACCESS(2022)
摘要
This article evaluates several machine learning methods to substitute the missing light detection and ranging data for better spatial localization of industrial automated guided vehicles. Decision trees and ensemble of trees using bagging or boosting techniques have been considered. Also, the k-nearest neighbors algorithm was analyzed. Most of the algorithms have been evaluated based on multiple criteria and hyper parameter tuning. The analysis of the results was done in a comparative way, multiple regression evaluation metrics being considered. The experiments have shown that the extreme gradient boosting algorithm provides the best results in terms of performance, but with timing and resource allocation drawbacks. On the other hand, a simple decision tree model seems to give good results if a tradeoff between performance and prediction time must be made. The k-nearest neighbors algorithm is also performing pretty well, especially because we are experimenting in a static environment.
更多查看译文
关键词
Automated guided vehicle, digital twin, LiDAR, point cloud estimation
AI 理解论文
溯源树
样例
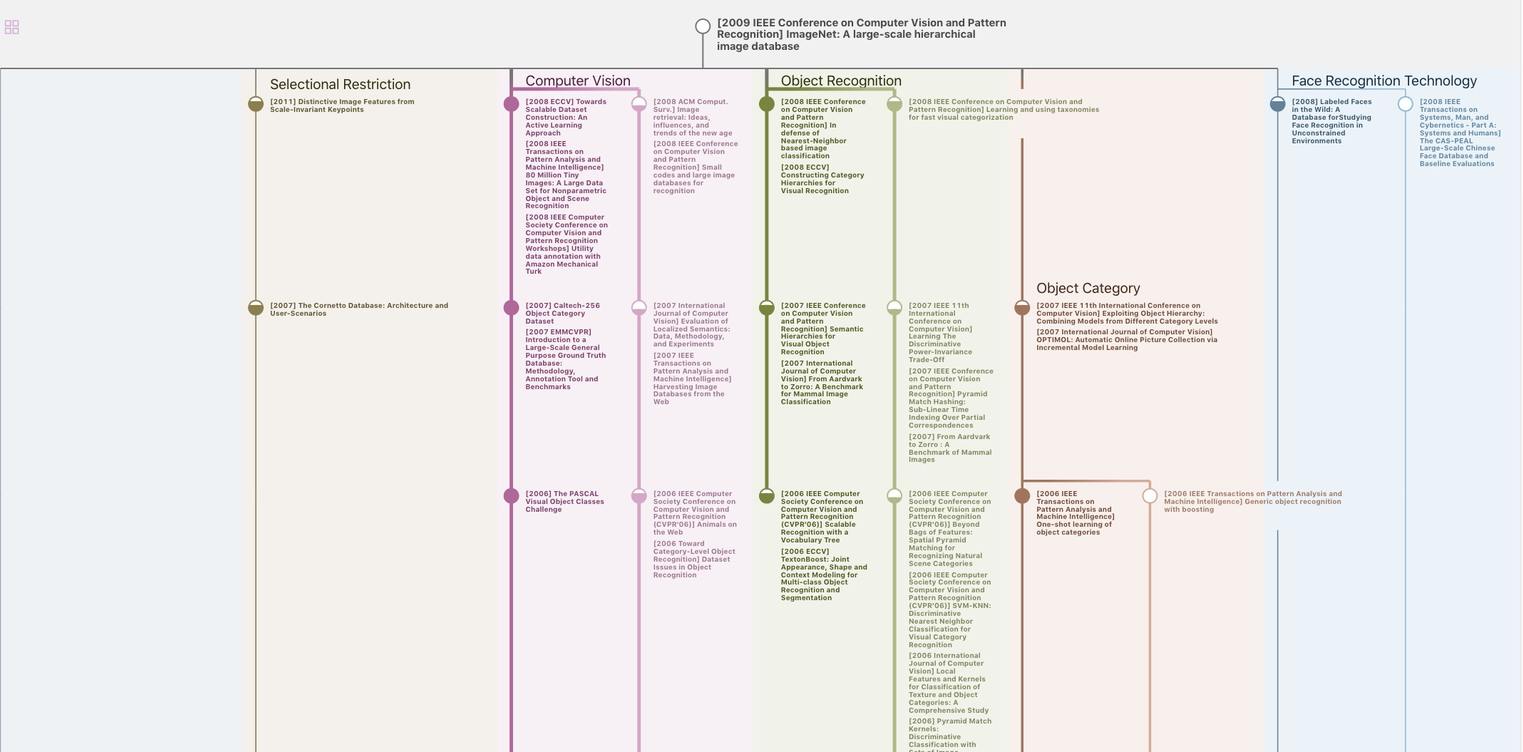
生成溯源树,研究论文发展脉络
Chat Paper
正在生成论文摘要