ELGONBP: A grouped neighboring intensity difference encoding for texture classification
MULTIMEDIA TOOLS AND APPLICATIONS(2022)
摘要
Local binary pattern (LBP) plays a crucial part in texture classification. Although plenty of LBP-based methods for texture extraction have achieved good classification results, most LBP variants focus on the relationships between the neighboring pixels and its central pixel in an image patch whereas ignoring the information among neighboring pixels, making the extracted texture descriptors not robust enough to external changes (illumination, rotation, noise, etc.). In this paper, a new texture descriptor, the extended local grouped order and non-local binary pattern (ELGONBP) is introduced. Firstly, we propose a first-order difference coding scheme which is based on the sign difference to encode grouped neighborhood difference information. In this way, we can obtain a more complete representation among neighboring sampling points. To further promote the robustness of texture descriptor, we perform cross-image domain information fusion, which combines the texture information in the original image domain and the gradient domain since image gradients contain rich structural information. Comprehensive experiments are implemented on four public representative texture databases and the noise robustness of different descriptors is evaluated. The experimental results prove that the presented ELGONBP descriptor has better classification performance and noise robustness compared with other state-of-the-art LBP descriptors.
更多查看译文
关键词
Texture descriptors,Local binary pattern (LBP),Neighboring intensity difference,Different image domains,Noise robustness
AI 理解论文
溯源树
样例
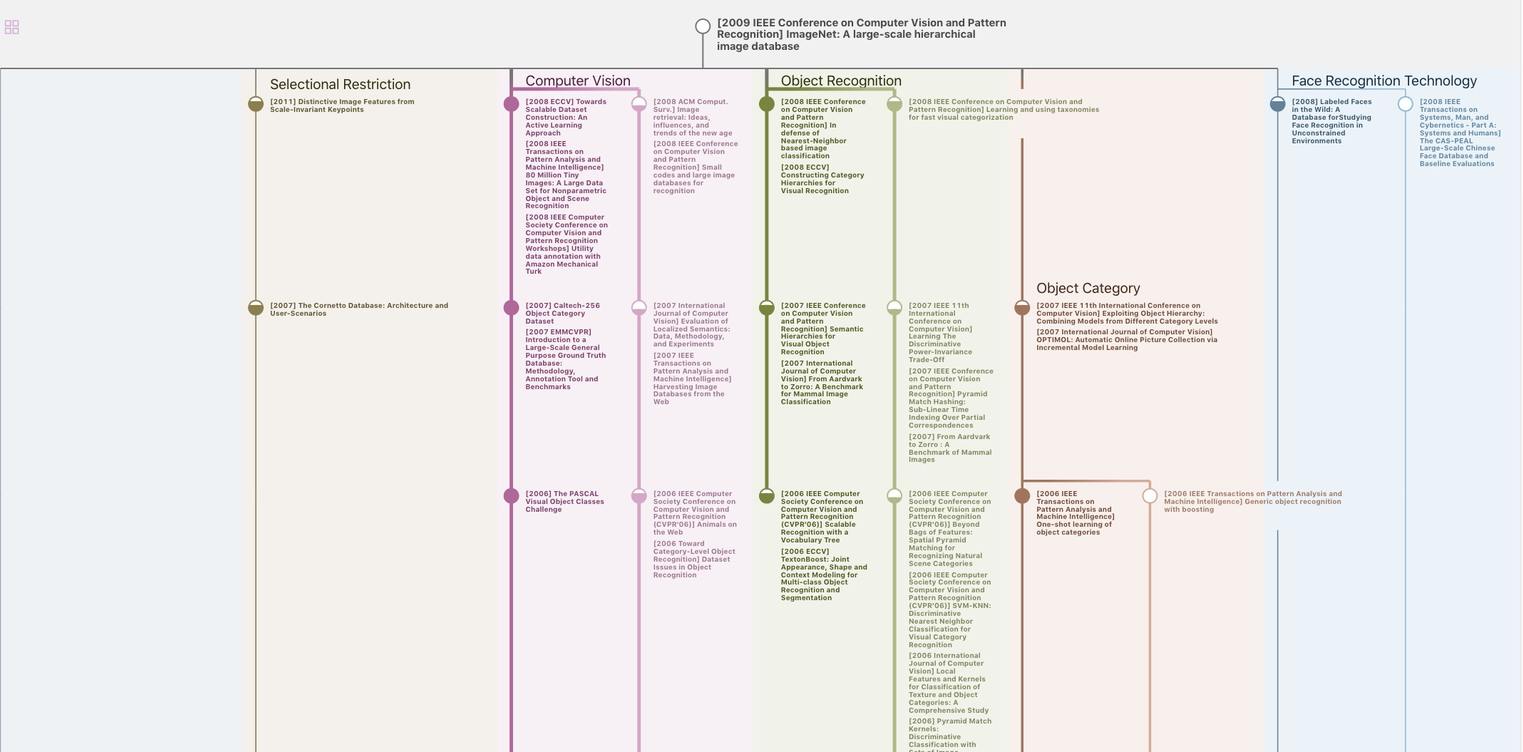
生成溯源树,研究论文发展脉络
Chat Paper
正在生成论文摘要