Principal component analysis based on graph embedding
Multimedia Tools and Applications(2022)
摘要
Dimensionality reduction plays an important role in image recognition and data mining. Traditional methods extract features from data itself and ignore the structure information of data even though it is crucial for effective representation. Considering graph embedding method can capture and model the complicated relationships among data, therefore, we consider to incorporate graph convolution learning into principal component analysis (GCPCA) to abstract more effective features in this paper. The key idea of the proposed model is embedding graph convolutional to realize linear representation by fusing the relationship of data points. Then PCA is operated on projected data to extract effective features. The model can be solved to obtain a globally optimal closed-form solution, which is convenient for implementation and practical application. Experiments on some publicly available datasets demonstrate that the proposed GCPCA model show the better performance than the existing classical algorithms in terms of classification accuracy.
更多查看译文
关键词
Dimensionality reduction, Graph embedding, Principal component analysis, Feature extraction
AI 理解论文
溯源树
样例
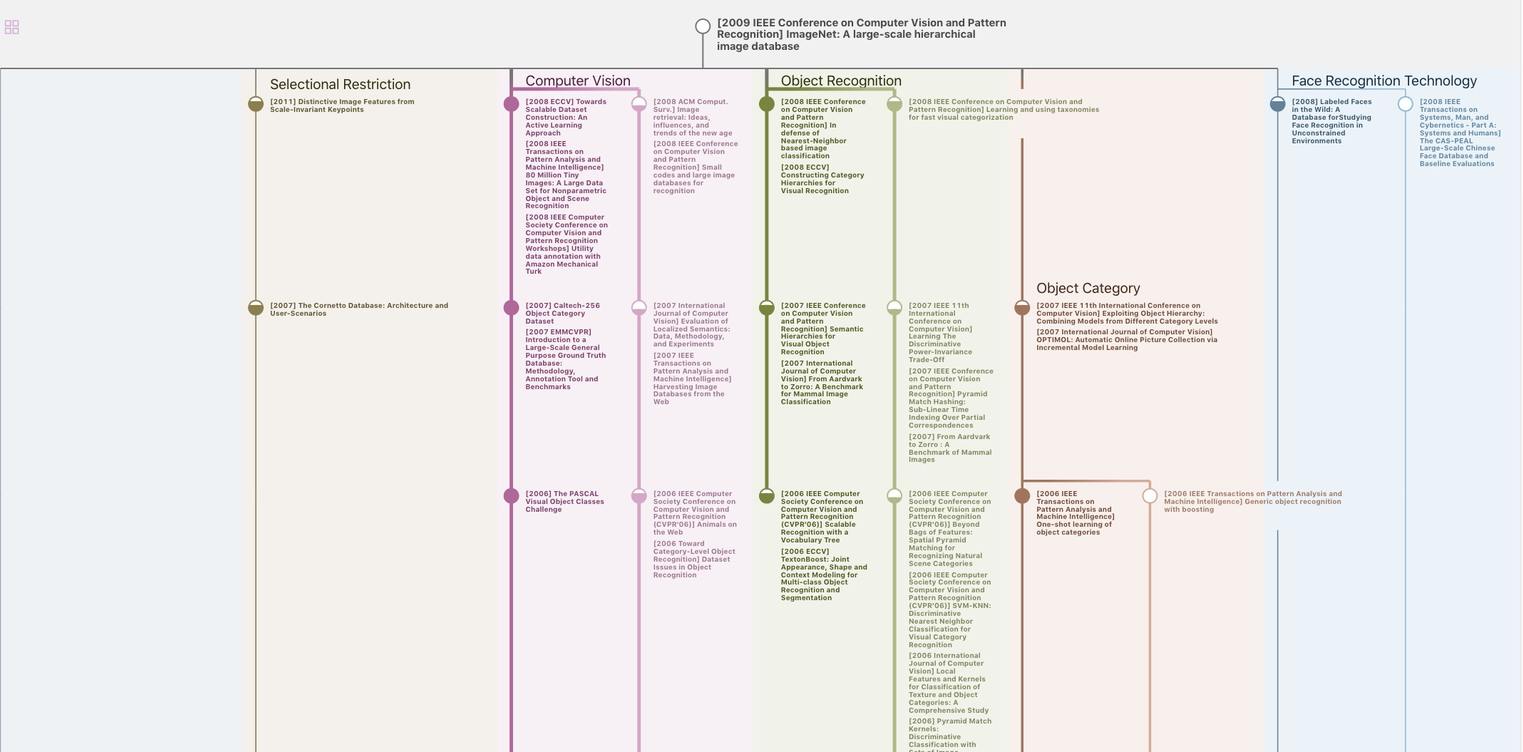
生成溯源树,研究论文发展脉络
Chat Paper
正在生成论文摘要