Ocular diseases classification using a lightweight CNN and class weight balancing on OCT images
MULTIMEDIA TOOLS AND APPLICATIONS(2022)
摘要
Optical coherence tomography (OCT) is a non-invasive technique to capture cross-sectional volumes of the human retina. OCT images are used for the diagnosis of various ocular diseases. However, OCT datasets generally suffer from the problem of class imbalance. This work aims to leverage CNN capability for OCT images classification in the presence of class imbalance. A lightweight convolutional neural network (CNN) with class weight balancing (CWB) is proposed for OCT image classification. Training of CNN is done while penalizing the classes having a higher number of samples using the CWB method. The performance of the proposed method is evaluated on spectral-domain OCT (SD-OCT) images from two publicly available datasets, namely, ZhangLab and Duke. The performance of the proposed method is evaluated on the confusion matrix-based parameters like accuracy, sensitivity, specificity, and F 1 − s c o r e . The proposed method achieved 99.17% and 98.46% accuracy for ZhangLab and Duke datasets, respectively. It is observed that the proposed method performs better as compared to most of the state-of-the-art OCT classification methods The generalizability and interpretability of the proposed method are also evaluated to improve the understanding of the CNN model.
更多查看译文
关键词
Ocular disease classification, Optical coherence tomography, Convolutional neural networks, Class weight balancing
AI 理解论文
溯源树
样例
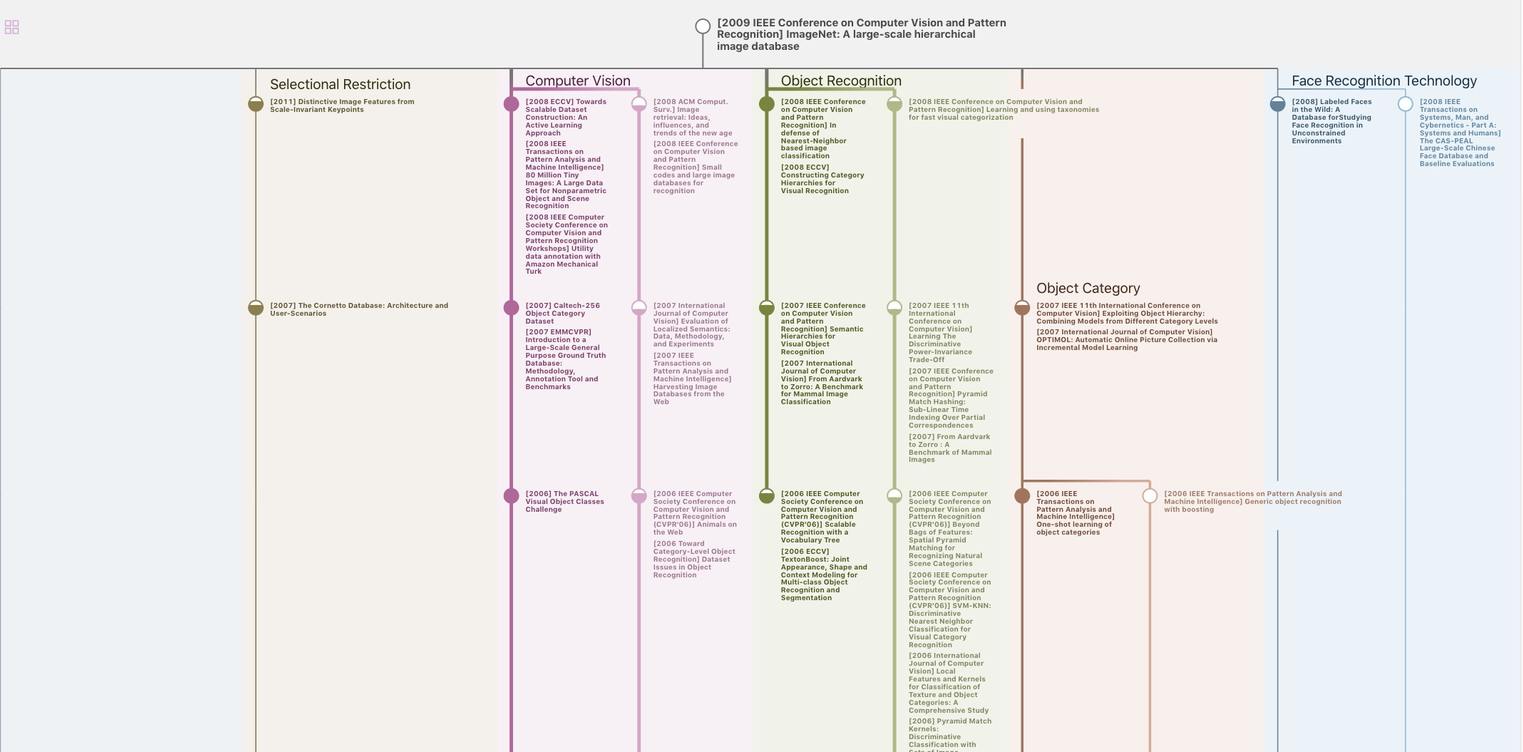
生成溯源树,研究论文发展脉络
Chat Paper
正在生成论文摘要