A Few-Shot Learning-Based Crashworthiness Analysis and Optimization for Multi-Cell Structure of High-Speed Train
MACHINES(2022)
摘要
Due to the requirement of significant manpower and material resources for the crashworthiness tests, various modelling approaches are utilized to reduce these costs. Despite being informative, finite element models still have the disadvantage of being time-consuming. A data-driven model has recently demonstrated potential in terms of computational efficiency, but it is also accompanied by challenges in collecting an amount of data. Few-shot learning is a perspective approach in addressing the problem of insufficient data in engineering. In this paper, using a novel hybrid data augmentation method, we investigate a deep-learning-based few-shot learning approach to evaluate and optimize the crashworthiness of multi-cell structures. Innovatively, we employ wide and deep neural networks to develop a surrogate model for multi-objective optimization. In comparison with the original results, the optimized result of the multi-cell structure demonstrates that the mean crushing force (F-m) and specific energy absorption (SEA) are increased by 17.1% and 30.1%, respectively, the mass decreases by 4.0%, and the optimized structure offers a significant improvement in design space. Overall, this proposed method exhibits great potential in relation to the crashworthiness analysis and optimization for multi-cell structures of the high-speed train.
更多查看译文
关键词
crashworthiness, few-shot learning, multi-cell structures, high-speed train
AI 理解论文
溯源树
样例
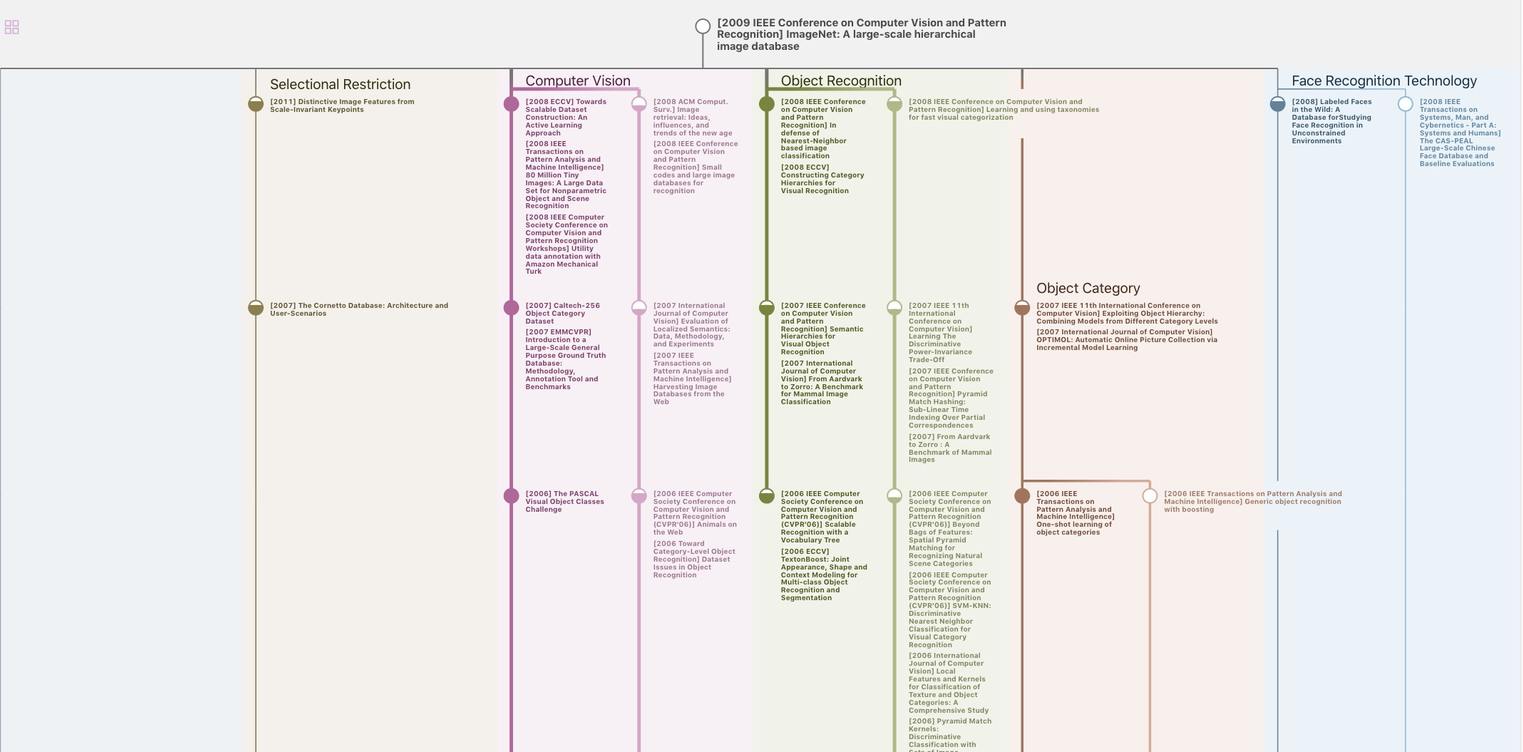
生成溯源树,研究论文发展脉络
Chat Paper
正在生成论文摘要