Residual Dense Autoencoder Network for Nonlinear Hyperspectral Unmixing
IEEE JOURNAL OF SELECTED TOPICS IN APPLIED EARTH OBSERVATIONS AND REMOTE SENSING(2022)
摘要
Hyperspectral unmixing is a popular research topic in hyperspectral processing, aiming at obtaining the ground features contained in the mixed pixels and their proportion. Recently, nonlinear mixing models have received particular attention in hyperspectral decomposition since the linear mixing model cannot suitably apply in the situation that exists in multiple scattering. In this article, we constructed a residual dense autoencoder network (RDAE) for nonlinear hyperspectral unmixing in multiple scattering scenarios. First, an encoder was built based on the residual dense network (RDN) and attention layer. The RDN is employed to characterize multiscale representations, which are further transformed with the attention layer to estimate the abundance maps. Second, we designed a decoder based on the unfolding of a generalized bilinear model to extract endmembers and estimate their second-order scattering interactions. Comparative experiments between the RDAE and six other state-of-the-art methods under synthetic and real hyperspectral datasets demonstrate that the proposed method achieved a better performance in terms of endmember extraction and abundance estimation.
更多查看译文
关键词
Hyperspectral imaging, Feature extraction, Decoding, Spatial resolution, Scattering, Licenses, Kernel, Autoencoder, generalized bilinear model (GBM), nonlinear hyperspectral unmixing, residual dense network (RDN)
AI 理解论文
溯源树
样例
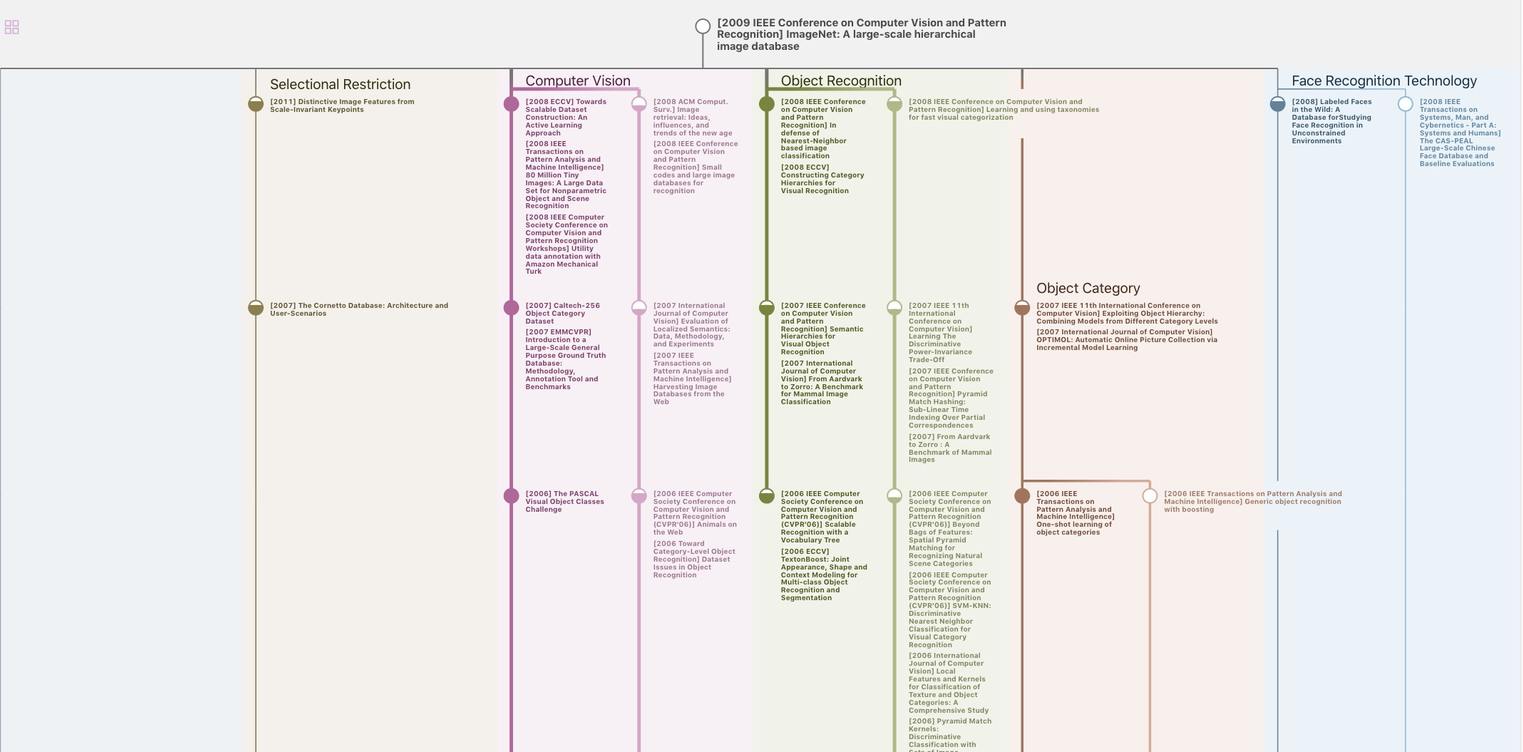
生成溯源树,研究论文发展脉络
Chat Paper
正在生成论文摘要