Which CAM is Better for Extracting Geographic Objects? A Perspective From Principles and Experiments
IEEE JOURNAL OF SELECTED TOPICS IN APPLIED EARTH OBSERVATIONS AND REMOTE SENSING(2022)
摘要
As a method of deep learning interpretability, class activation mapping (CAM) is efficient and convenient for extracting geographic objects supervised by image-level labels. However, in addition to the inherent problem of inaccuracy and incompleteness of CAM, we have to deal with the spectral and spatial variance of geographic objects when applying CAM methods to remote sensing images. To explore the capabilities of CAM methods on extracting various geographic objects, we make a comprehensive comparison of five commonly-used CAM methods, including original CAM, GradCAM, GradCAM++, SmoothGradCAM++, and ScoreCAM, in four aspects: efficiency; accuracy; effectiveness on dealing with the spectral and spatial variance; and performance of delineating different geographic object categories. The results demonstrate that the original CAM, GradCAM, and GradCAM++ achieves the highest efficiency, accuracy, and integrity for extracting geographic objects, respectively, which can help us choose the appropriate CAM methods according to the specific requirements of different extraction tasks. Benefiting from the capability in extracting various geographic objects and adaptability in complex scenes, GradCAM achieves the best performance in dealing with the spectral and spatial variance problem and shows the advantage of capturing object details and keeping object completeness at the same time. In addition to the comparison experiments and suggestions, we also provide the principle explanations of the performance differences. The findings of this article could contribute to a deep understanding of different CAM methods and benefit to selecting suitable CAM methods for extracting geographic objects from the perspectives of both principles and experiments.
更多查看译文
关键词
Feature extraction, Remote sensing, Convolutional neural networks, Task analysis, Heating systems, Data mining, Training, Class activation mapping (CAM), convolutional neural network (CNN), deep learning, geographic objects, weakly-supervised semantic segmentation
AI 理解论文
溯源树
样例
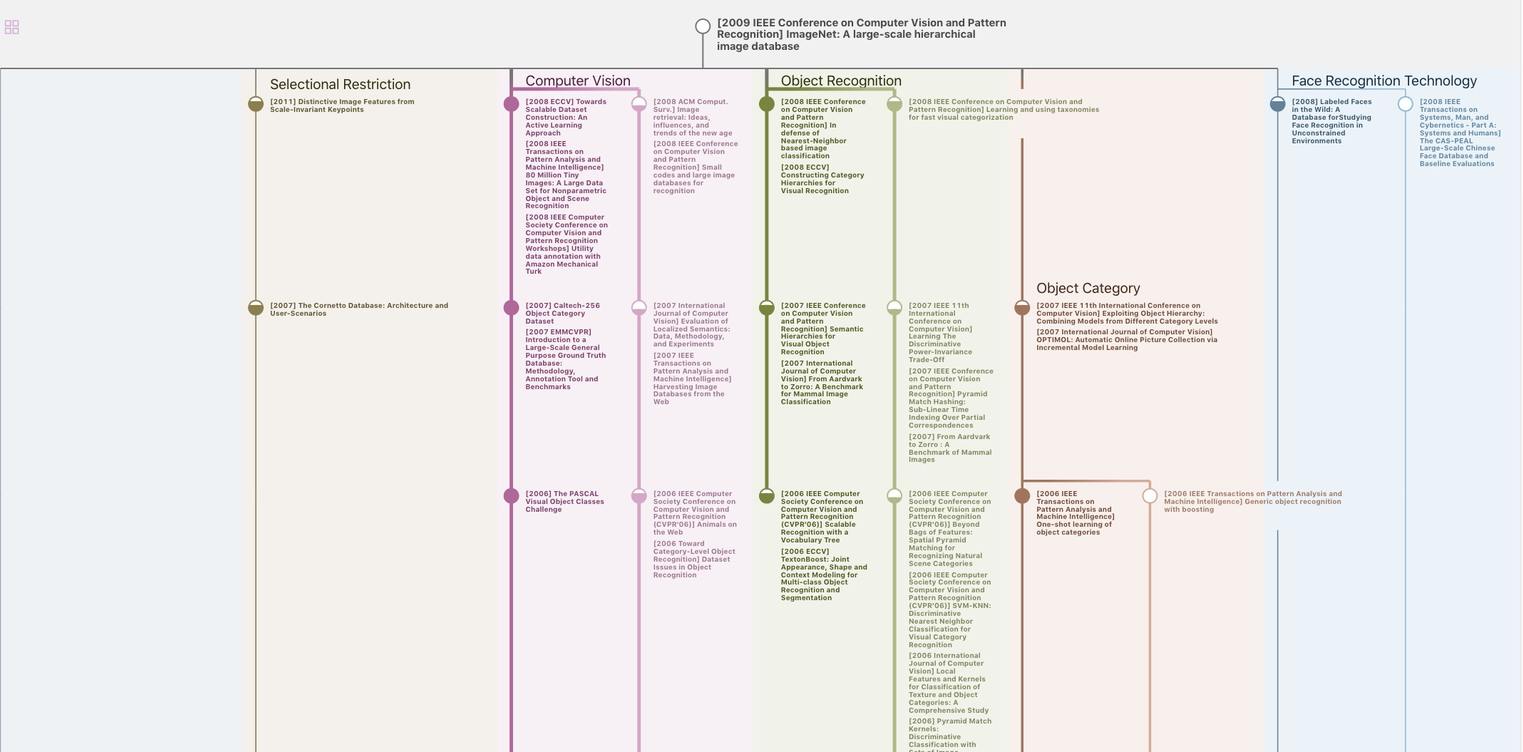
生成溯源树,研究论文发展脉络
Chat Paper
正在生成论文摘要