Multimodal Prediction of Five-Year Breast Cancer Recurrence in Women Who Receive Neoadjuvant Chemotherapy
CANCERS(2022)
摘要
Simple Summary An important clinical issue to consider when selecting neoadjuvant chemotherapy treatment for breast cancer is the likelihood of cancer recurrence. Accurately predicting the future outcome of the patient based on data available prior to treatment initiation could impact the treatment selection. We study a cohort of 1738 patients and explore the contribution of clinical history, immunohistochemical markers, and multiparametric magnetic resonance imaging to the prediction of post-treatment cancer recurrence. We analyzed this multimodal data using classical machine learning, image processing, and deep learning to increase the set of discriminating features. Our results demonstrate the ability to predict recurrence using each modality alone, and the possible improvement achieved by combining the modalities. We show that the models are especially accurate for differentiating specific groups of young women with poor prognoses. These methods were also used on a different dataset of 193 patients in an international challenge, where they won second place. In current clinical practice, it is difficult to predict whether a patient receiving neoadjuvant chemotherapy (NAC) for breast cancer is likely to encounter recurrence after treatment and have the cancer recur locally in the breast or in other areas of the body. We explore the use of clinical history, immunohistochemical markers, and multiparametric magnetic resonance imaging (DCE, ADC, Dixon) to predict the risk of post-treatment recurrence within five years. We performed a retrospective study on a cohort of 1738 patients from Institut Curie and analyzed the data using classical machine learning, image processing, and deep learning. Our results demonstrate the ability to predict recurrence prior to NAC treatment initiation using each modality alone, and the possible improvement achieved by combining the modalities. When evaluated on holdout data, the multimodal model achieved an AUC of 0.75 (CI: 0.70, 0.80) and 0.57 specificity at 0.90 sensitivity. We then stratified the data based on known prognostic biomarkers. We found that our models can provide accurate recurrence predictions (AUC > 0.89) for specific groups of women under 50 years old with poor prognoses. A version of our method won second place at the BMMR2 Challenge, with a very small margin from being first, and was a standout from the other challenge entries.
更多查看译文
关键词
breast cancer recurrence, neoadjuvant chemotherapy, magnetic resonance imaging (MRI), machine learning, deep learning, image processing, radiomics
AI 理解论文
溯源树
样例
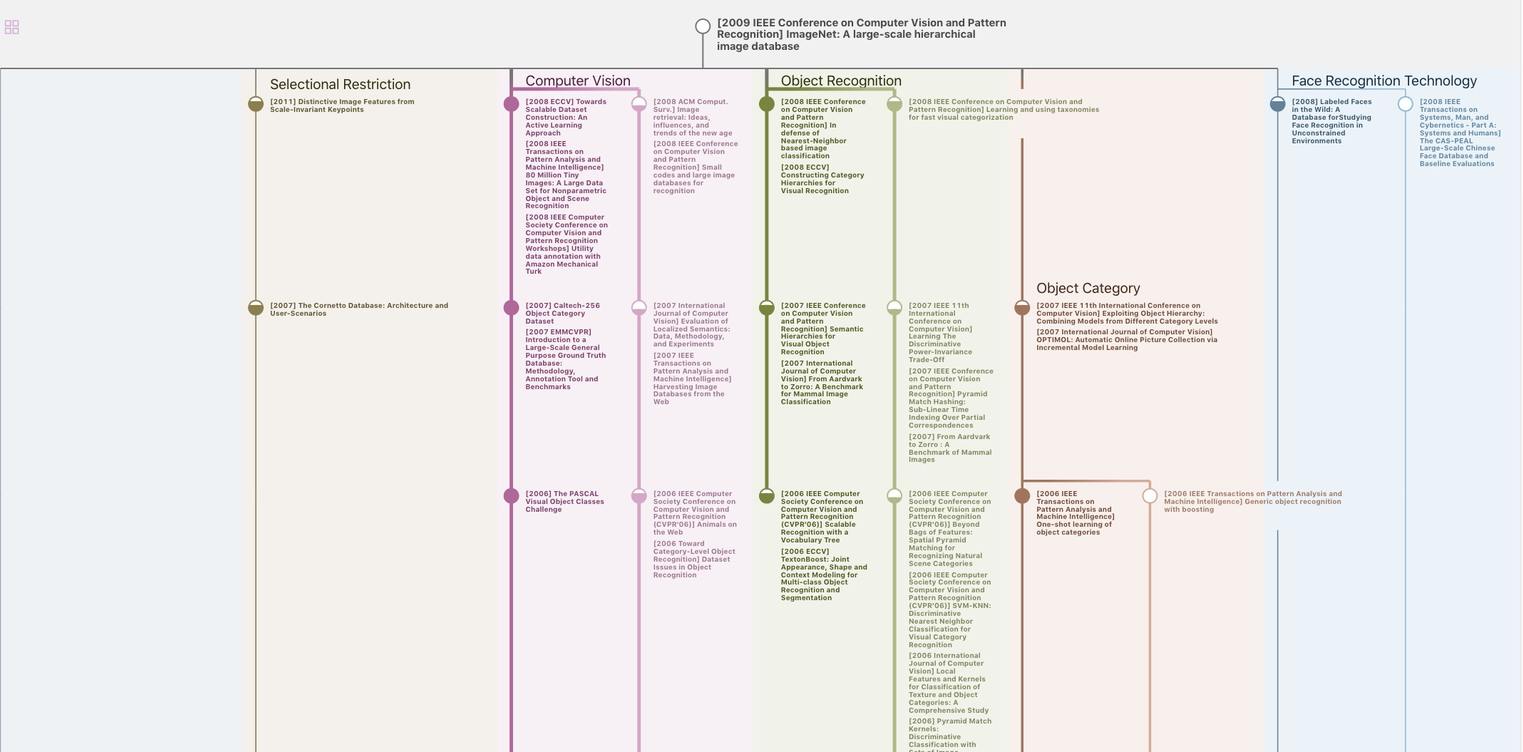
生成溯源树,研究论文发展脉络
Chat Paper
正在生成论文摘要