Application of Generative Adversarial Network and Diverse Feature Extraction Methods to Enhance Classification Accuracy of Tool-Wear Status
ELECTRONICS(2022)
摘要
The means of accurately determining tool-wear status has long been important to manufacturers. Tool-wear status classification enables factories to avoid the unnecessary costs incurred by replacing tools too early and to prevent product damage caused by overly worn tools. While researchers have examined this topic for over a decade, most existing studies have focused on model development but have neglected two fundamental issues in machine learning: data imbalance and feature extraction. In view of this, we propose two improvements: (1) using a generative adversarial network to generate realistic computer numerical control machine vibration data to overcome data imbalance and (2) extracting features in the time domain, the frequency domain, and the time-frequency domain simultaneously for modeling and integrating these in an ensemble model. The experiment results demonstrate how both proposed modifications are reasonable and valid.
更多查看译文
关键词
tool wear, data imbalance, GAN, ensemble learning
AI 理解论文
溯源树
样例
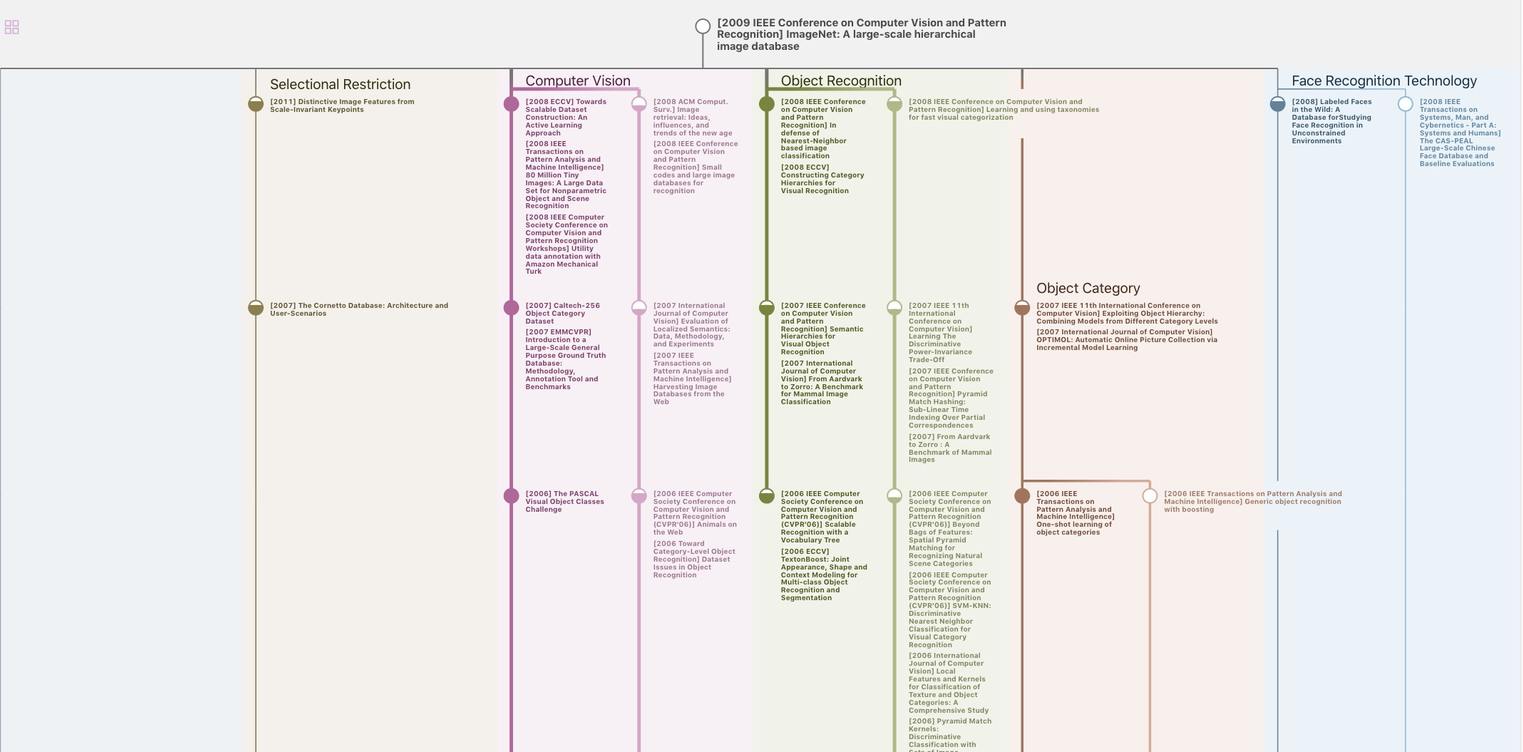
生成溯源树,研究论文发展脉络
Chat Paper
正在生成论文摘要