Zero-Attracting Kernel Maximum Versoria Criterion Algorithm for Nonlinear Sparse System Identification
IEEE SIGNAL PROCESSING LETTERS(2022)
摘要
Sparsity-induced kernel adaptive filters have emerged as a promising candidate for a nonlinear sparse system identification (SSI) problem. The existing zero-attracting kernel least mean square (ZA-KLMS) algorithm relies on minimum mean square error criterion, which considers only second order statistics of error, thereby resulting in suboptimal performance in the presence of non-Gaussian/impulsive distortions. In this letter, we propose a novel random Fourier features (RFF) based ZA kernel maximum Versoria criterion (ZA-KMVC) algorithm, and their variants, which are robust for nonlinear SSI in the presence of non-Gaussian distortions over both stationary and time-varying environments. Furthermore, the mean-square convergence analysis of the proposed RFF-ZA-KMVC algorithm is performed. It has been observed from the simulation results that the proposed algorithm delivers better convergence performance as compared to the existing state-of-art approaches.
更多查看译文
关键词
Signal processing algorithms, Kernel, Prediction algorithms, Cost function, Convergence, Adaptive filters, Steady-state, KLMS, maximum Versoria criterion, non-Gaussian, random Fourier features, reproducing kernel Hilbert space, sparsity-aware, ZA-KLMS, ZA-KMVC, zero-attracting
AI 理解论文
溯源树
样例
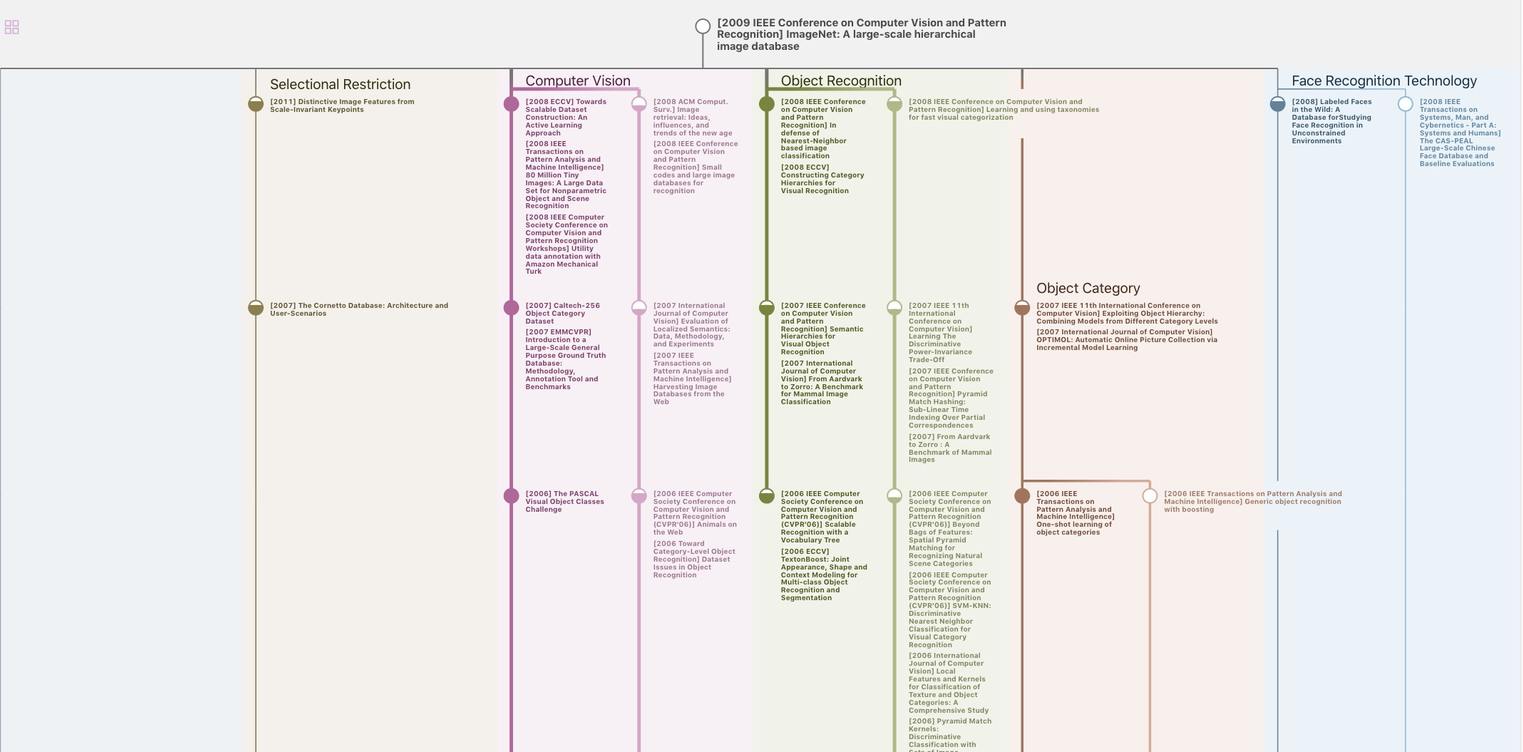
生成溯源树,研究论文发展脉络
Chat Paper
正在生成论文摘要