Whitening Technique Based on Gram-Schmidt Orthogonalization for Motor Imagery Classification of Brain-Computer Interface Applications
SENSORS(2022)
摘要
A novel whitening technique for motor imagery (MI) classification is proposed to reduce the accuracy variance of brain-computer interfaces (BCIs). This method is intended to improve the electroencephalogram eigenface analysis performance for the MI classification of BCIs. In BCI classification, the variance of the accuracy among subjects is sensitive to the accuracy itself for superior classification results. Hence, with the help of Gram-Schmidt orthogonalization, we propose a BCI channel whitening (BCICW) scheme to minimize the variance among subjects. The newly proposed BCICW method improved the variance of the MI classification in real data. To validate and verify the proposed scheme, we performed an experiment on the BCI competition 3 dataset IIIa (D3D3a) and the BCI competition 4 dataset IIa (D4D2a) using the MATLAB simulation tool. The variance data when using the proposed BCICW method based on Gram-Schmidt orthogonalization was much lower (11.21) than that when using the EFA method (58.33) for D3D3a and decreased from (17.48) to (9.38) for D4D2a. Therefore, the proposed method could be effective for MI classification of BCI applications.
更多查看译文
关键词
whitening technique, Gram-Schmidt orthogonalization, motor imagery classification, eigenface analysis
AI 理解论文
溯源树
样例
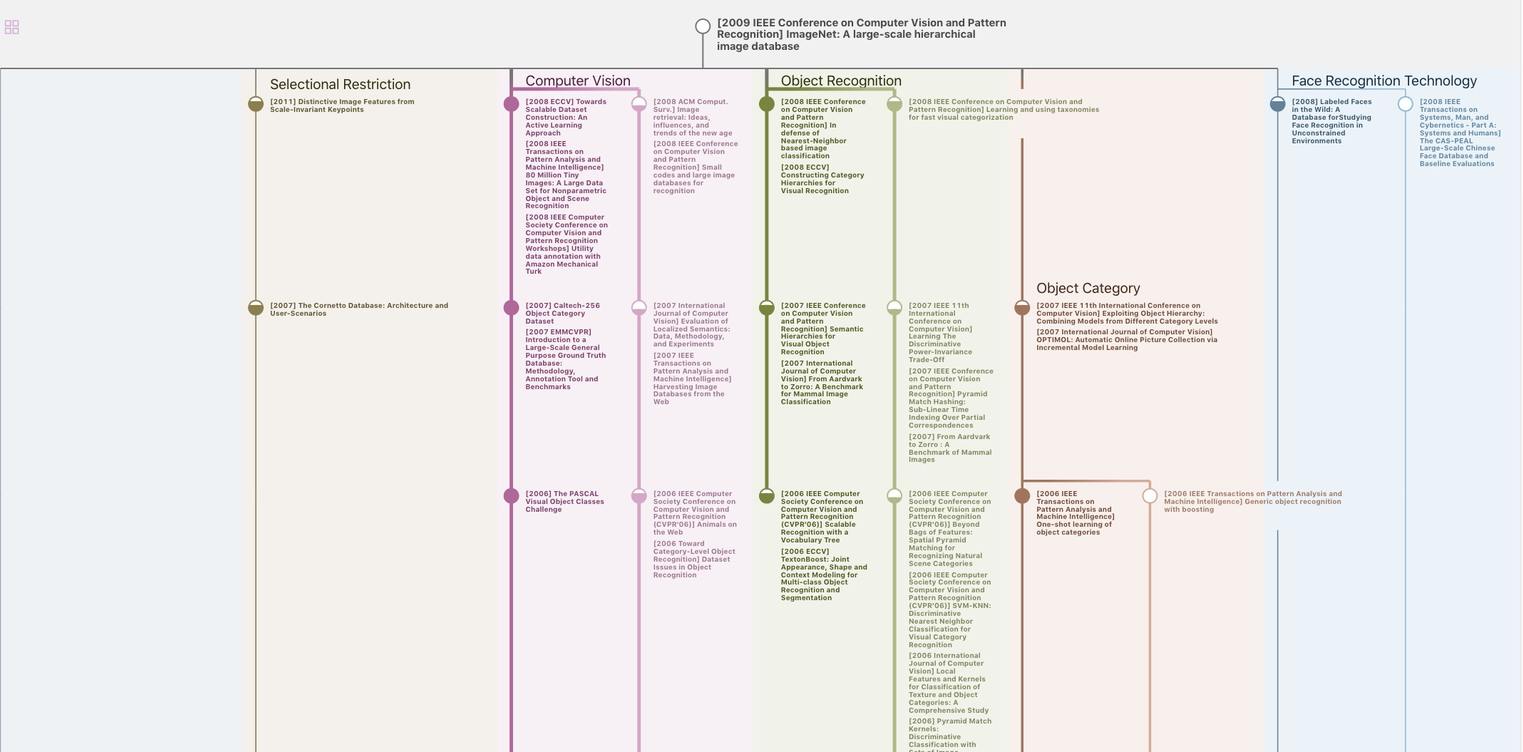
生成溯源树,研究论文发展脉络
Chat Paper
正在生成论文摘要