Complex-Valued Multi-Scale Fully Convolutional Network with Stacked-Dilated Convolution for PolSAR Image Classification
REMOTE SENSING(2022)
摘要
Polarimetric synthetic aperture radar (PolSAR) image classification is a pixel-wise issue, which has become increasingly prevalent in recent years. As a variant of the Convolutional Neural Network (CNN), the Fully Convolutional Network (FCN), which is designed for pixel-to-pixel tasks, has obtained enormous success in semantic segmentation. Therefore, effectively using the FCN model combined with polarimetric characteristics for PolSAR image classification is quite promising. This paper proposes a novel FCN model by adopting complex-valued domain stacked-dilated convolution (CV-SDFCN). Firstly, a stacked-dilated convolution layer with different dilation rates is constructed to capture multi-scale features of PolSAR image; meanwhile, the sharing weight is employed to reduce the calculation burden. Unfortunately, the labeled training samples of PolSAR image are usually limited. Then, the encoder-decoder structure of the original FCN is reconstructed with a U-net model. Finally, in view of the significance of the phase information for PolSAR images, the proposed model is trained in the complex-valued domain rather than the real-valued domain. The experiment results show that the classification performance of the proposed method is better than several state-of-the-art PolSAR image classification methods.
更多查看译文
关键词
fully convolutional network, stacked dilated convolution, complex-valued domain, PolSAR image classificationn
AI 理解论文
溯源树
样例
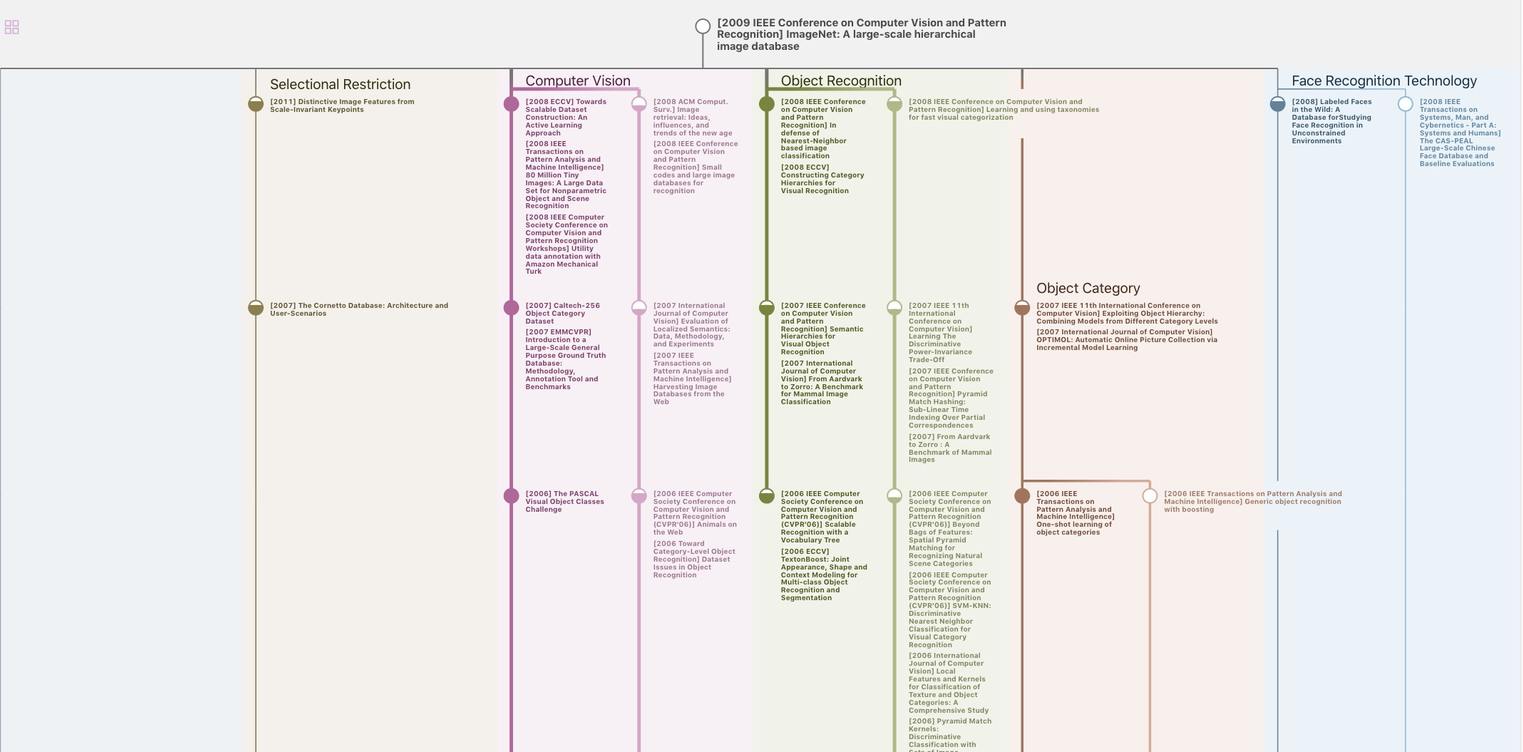
生成溯源树,研究论文发展脉络
Chat Paper
正在生成论文摘要