Regularizing the Deepsurv Network Using Projection Loss for Medical Risk Assessment
IEEE ACCESS(2022)
摘要
State-of-the-art deep survival prediction approaches expand network parameters to accommodate performance over a fine discretization of output time. For medical applications where data are limited, the regression-based Deepsurv approach is more advantageous because its continuous output design limits unnecessary network parameters. Despite the practical advantage, the typical network lacks control over the feature distribution causing the network to be more prone to noisy information and occasional poor prediction performance. We propose a novel projection loss as a regularizing objective to improve the time-to-event Deepsurv model. The loss formulation maximizes the lower bound of the multiple-correlation coefficient between the network's features and the desired hazard value. Reducing the loss also theoretically lowers the upper bound on the likelihood of discordant pair and improves C-index performance. We observe superior performances and robustness of regularized Deepsurv over many state-of-the-art approaches in our experiments with five public medical datasets and two cross-cohort validation tasks.
更多查看译文
关键词
Hazards, Biomedical imaging, Training, Data models, Task analysis, Indexes, Oncology, Machine learning, pattern recognition, supervised learning, medical expert systems, biomedical computing
AI 理解论文
溯源树
样例
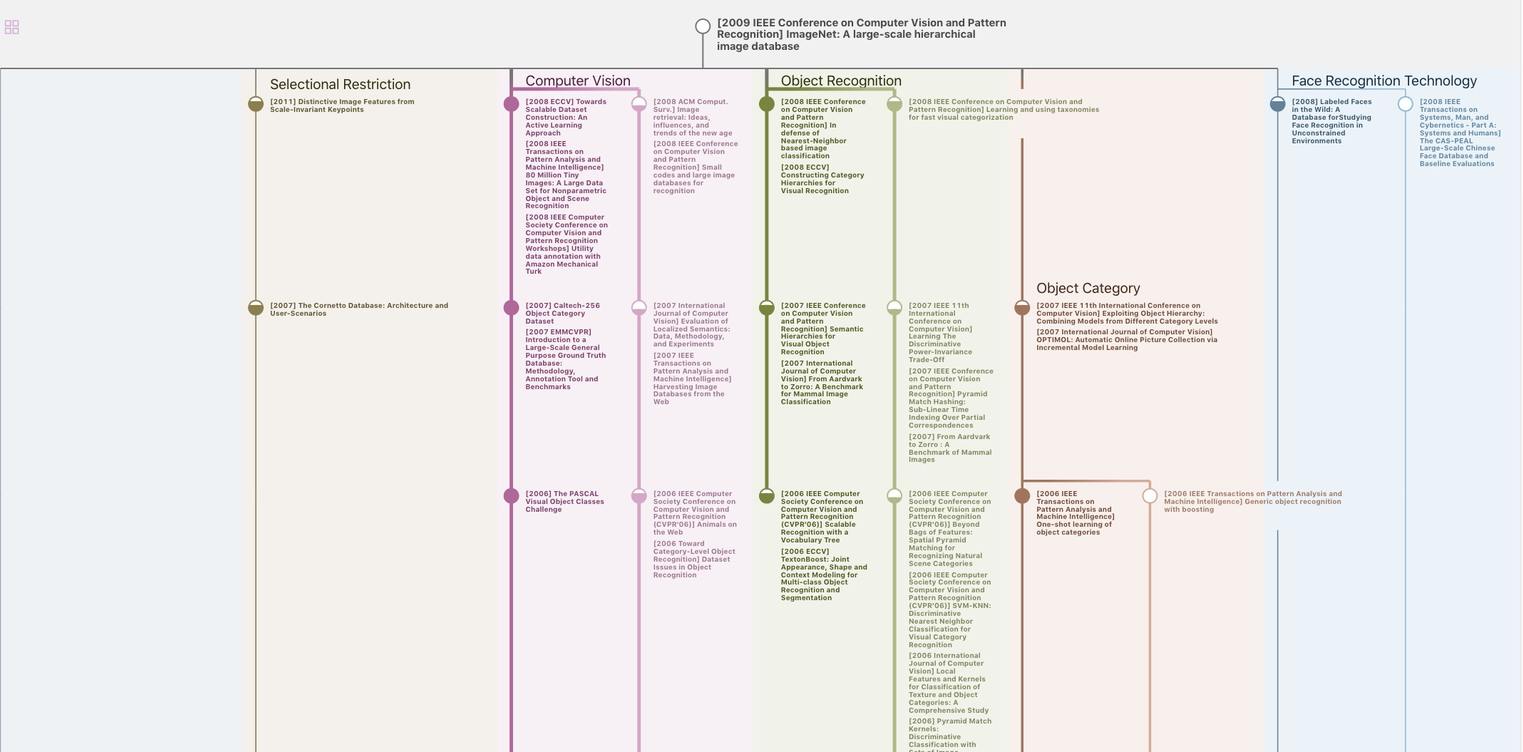
生成溯源树,研究论文发展脉络
Chat Paper
正在生成论文摘要