Poincare Plot Nonextensive Distribution Entropy: A New Method for Electroencephalography (EEG) Time Series
SENSORS(2022)
摘要
As a novel form of visual analysis technique, the Poincare plot has been used to identify correlation patterns in time series that cannot be detected using traditional analysis methods. In this work, based on the nonextensive of EEG, Poincare plot nonextensive distribution entropy (NDE) is proposed to solve the problem of insufficient discrimination ability of Poincare plot distribution entropy (DE) in analyzing fractional Brownian motion time series with different Hurst indices. More specifically, firstly, the reasons for the failure of Poincare plot DE in the analysis of fractional Brownian motion are analyzed; secondly, in view of the nonextensive of EEG, a nonextensive parameter, the distance between sector ring subintervals from the original point, is introduced to highlight the different roles of each sector ring subinterval in the system. To demonstrate the usefulness of this method, the simulated time series of the fractional Brownian motion with different Hurst indices were analyzed using Poincare plot NDE, and the process of determining the relevant parameters was further explained. Furthermore, the published sleep EEG dataset was analyzed, and the results showed that the Poincare plot NDE can effectively reflect different sleep stages. The obtained results for the two classes of time series demonstrate that the Poincare plot NDE provides a prospective tool for single-channel EEG time series analysis.
更多查看译文
关键词
nonextensive, distribution entropy, Poincare plot, sector ring subinterval
AI 理解论文
溯源树
样例
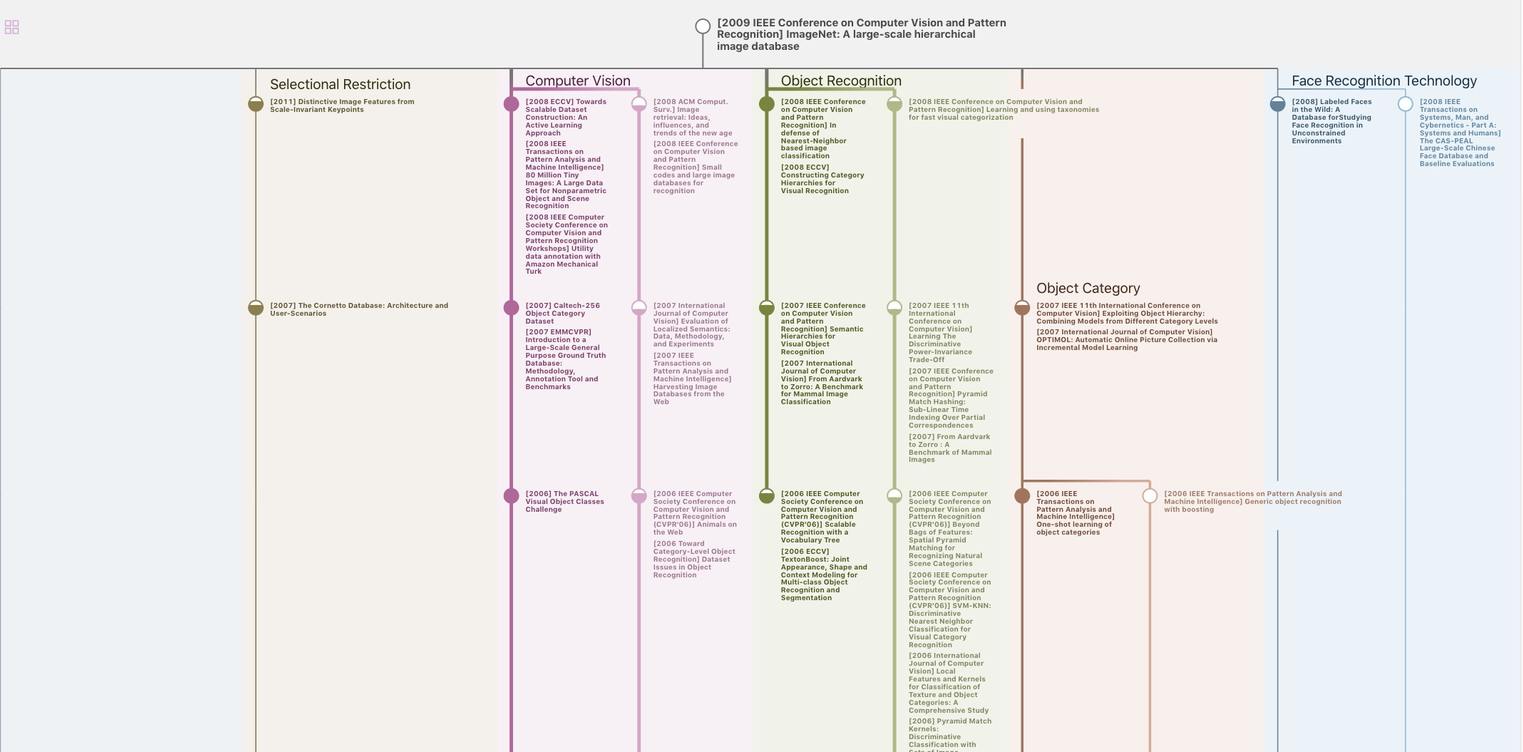
生成溯源树,研究论文发展脉络
Chat Paper
正在生成论文摘要