Discriminating Fake and Real Smiles Using Electroencephalogram Signals With Convolutional Neural Networks
IEEE ACCESS(2022)
摘要
Genuineness of smiles is of particular interest in the field of human emotions and social interactions. In this work, we develop an experimental protocol to elicit genuine and fake smile expressions on 28 healthy subjects. Then, we assess the type of smile expressions using electroencephalogram (EEG) signals with convolutional neural networks (CNNs). Five different architectures (CNN1, CNN2, CNN3, CNN4, and CNN5) were examined to differentiate between fake and real smiles. We transform the temporal EEG signals into normalized gray-scale images and perform three-way classification to classify fake smiles, genuine smiles, and neutral expressions in the form of subject-dependent classification. We achieved the highest classification accuracy of 90.4% using CNN1 for the full EEG spectrum. Likewise, we achieved classification accuracies of 87.4%, 88.3%, 89.7%, and 90.0% using Beta, Alpha, Theta, and Delta EEG bands respectively. This paper suggests that CNNs models, widely used in image classification problems, can provide an alternative approach for smile detection from physiological signals such as the EEG.
更多查看译文
关键词
Electroencephalography, Convolutional neural networks, Videos, Gesture recognition, Support vector machines, Hidden Markov models, Feature extraction, Smile, emotion, electroencephalogram (EEG), convolutional neural networks (CNNs), machine learning
AI 理解论文
溯源树
样例
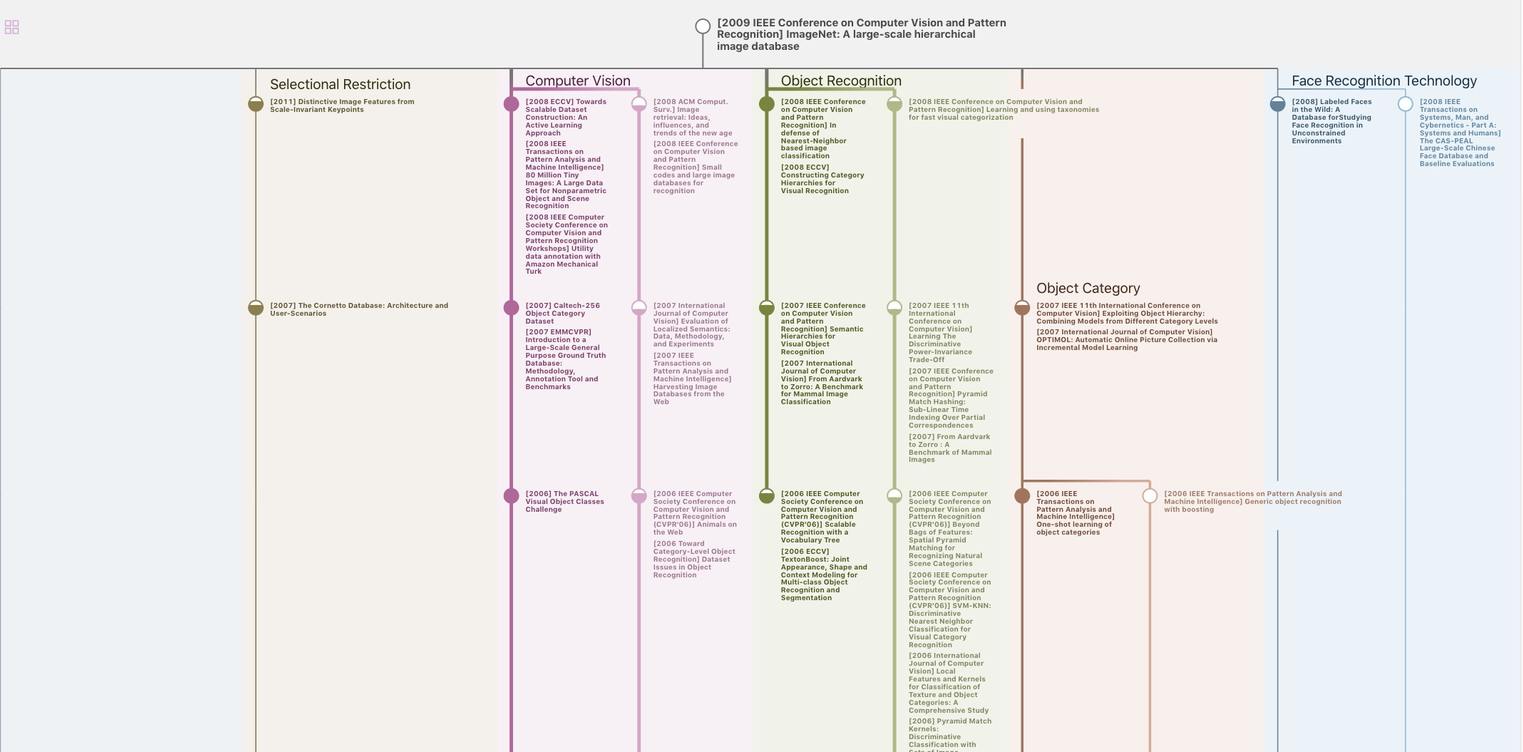
生成溯源树,研究论文发展脉络
Chat Paper
正在生成论文摘要