Resource Allocation for Cellular Zero-Touch Deterministic Industrial M2M Networks: A Reinforcement Learning-Based Scheme
IEEE Sensors Letters(2022)
摘要
In this letter, we investigate the resource allocation problem for cellular zero-touch deterministic industrial machine-to-machine networks. We consider a scenario in which multiple self-adaptive industrial machine-to-machine (M2M) networks are deployed underlaying cellular networks to emulate a variety of vertical use cases in future Industry 4.0 with the objective of guaranteeing the determinacy of latency by learning-inspired resource allocation policy. Specifically, in order to figure out the relationship between latency determinacy and the utilization of wireless resources meanwhile modeling the uncertainty of the stochastic environment, we first formulate the resource allocation problem as a Markov game by jointly considering transmission power control, frequency spectrum allocation, and the selection of base stations. Afterward, a random graph-based sparse long short-term memory network is proposed to solve the optimization problem while reducing the computational complexity. Finally, numerical result demonstrates the effectiveness of the proposed scheme.
更多查看译文
关键词
Sensor networks,deterministic networks,industrial IOT,long short-term memory (LSTM),resource allocation,zero-touch networks
AI 理解论文
溯源树
样例
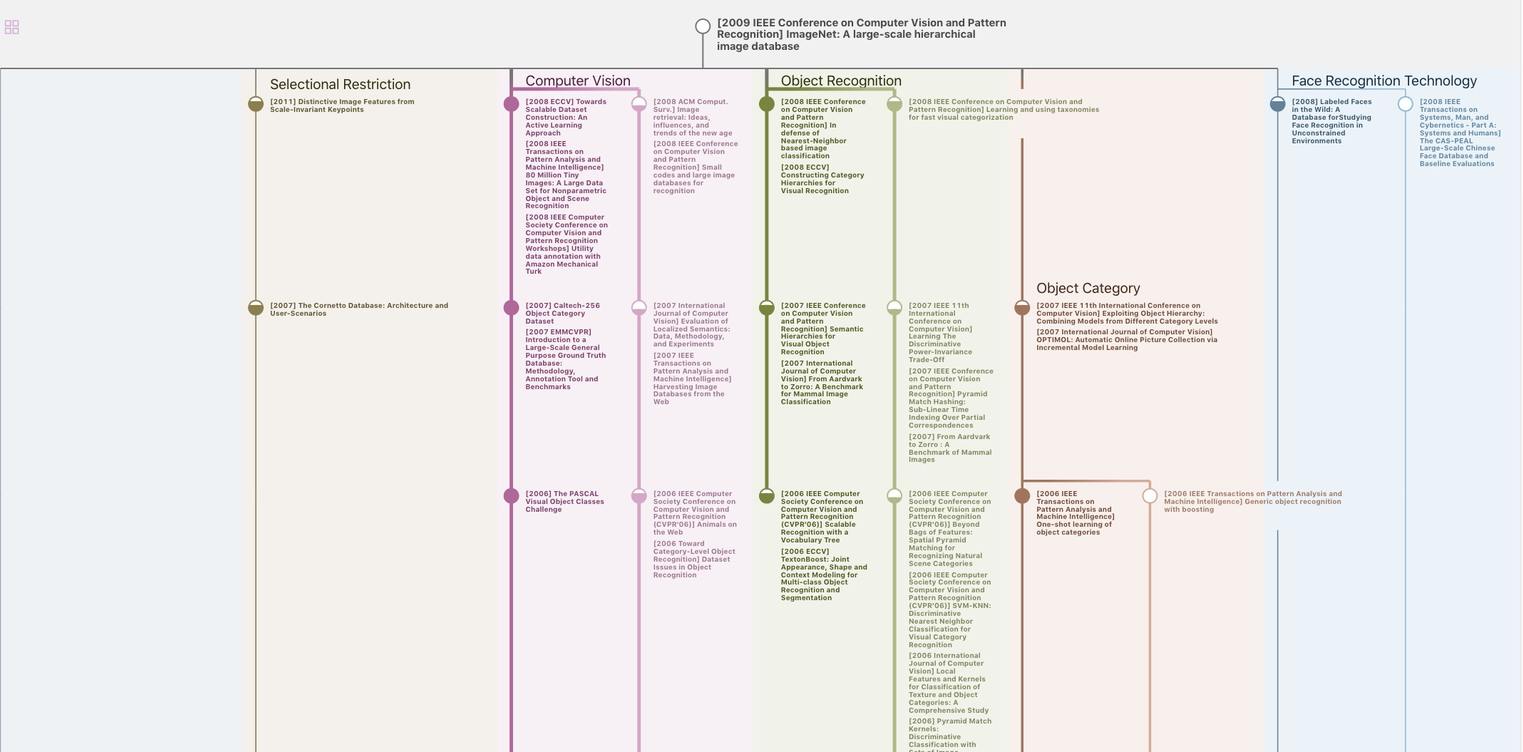
生成溯源树,研究论文发展脉络
Chat Paper
正在生成论文摘要