Bearing Fault Detection Based on Improved Multiscale Dispersion Entropy and Single Value Classification
IEEE Sensors Journal(2022)
摘要
In view of the difficulty in extracting bearing fault feature data and the problem of fault detection affected by the inaccurate determination of classical domains when using Extenics for fault detection. In this paper, a bearing fault detection method based on improved multi-scale dispersion entropy and single value classification is proposed. The improved multi-scale dispersion entropy is used to extract the characteristic parameters representing the running state of the bearing, the Fisher ratio is used for feature selection and dimension reduction, and the matter element models of the four running states of the bearing are established. The classical domains of the characteristic parameters of the four running states of the bearing are determined by using the single value classification method, and the matter elements of the four running states of the bearing are established. The running state of the bearing is determined by calculating the comprehensive correlation value between the samples to be tested and the matter elements in different running states. The results show that the accuracy of the proposed method is 99.25%. In order to verify the effectiveness of the feature extraction method proposed in this paper, the improved multi-scale dispersion entropy is compared with the refined composite multiscale dispersion entropy method. In order to verify the superiority of the classical domain determination method proposed in this paper, the single value classification method is compared with the mathematical statistics method. The experimental results verify the effectiveness of the methods proposed in this paper.
更多查看译文
关键词
Bearing fault detection,improved multi-scale dispersion entropy,single value classification
AI 理解论文
溯源树
样例
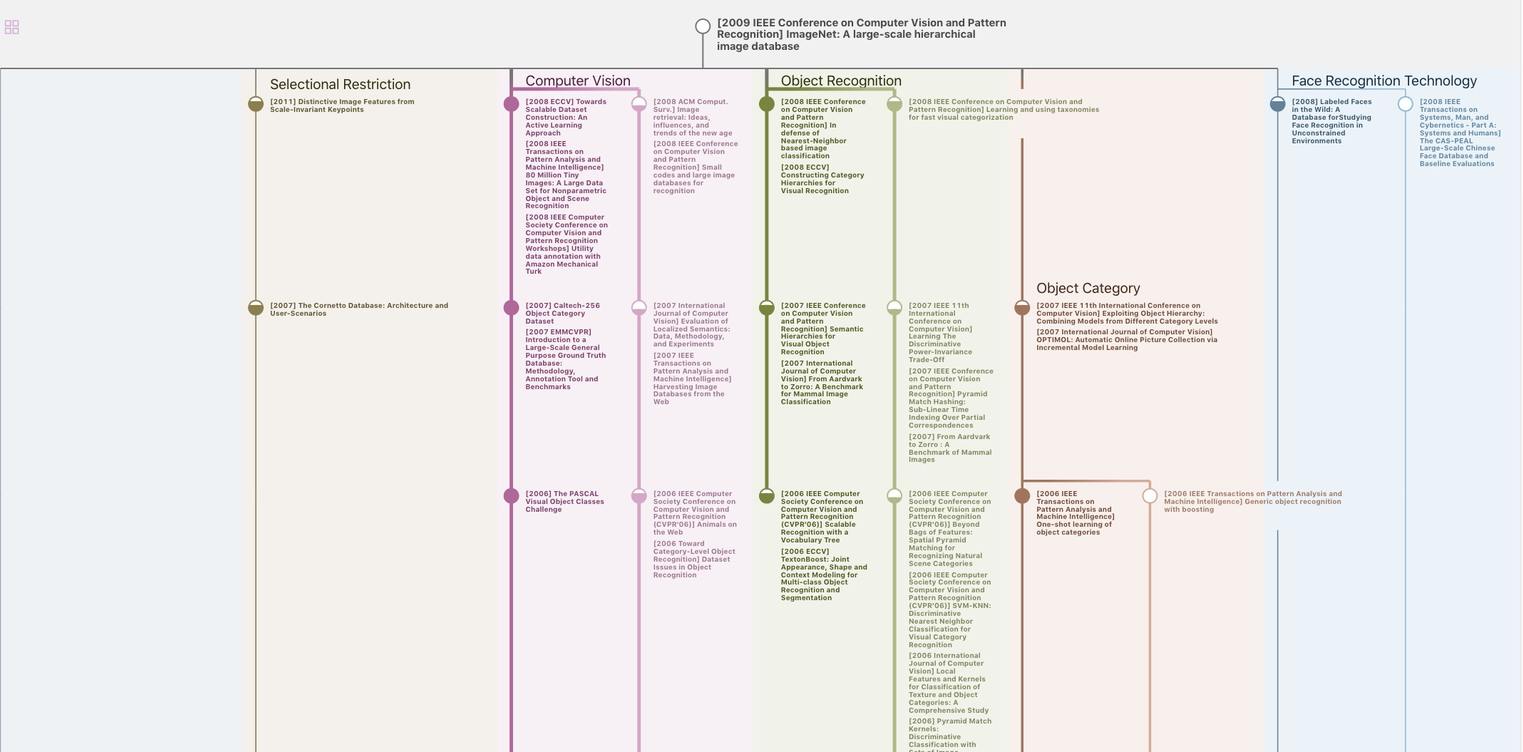
生成溯源树,研究论文发展脉络
Chat Paper
正在生成论文摘要