Spatio-Temporal Image Representation and Deep-Learning-Based Decision Framework for Automated Vehicles
IEEE TRANSACTIONS ON INTELLIGENT TRANSPORTATION SYSTEMS(2022)
摘要
Driving maneuver decision-making is critical to the development and mass deployment of automated vehicles (AVs). The prevailing approaches are stuck with either specific optimization objectives or separate maneuver planning. Inspired by the inherent driving mechanism of human beings, we develop a comprehensive deep learning scheme that extracts and establishes informative image-based representation of dynamic traffic flows and then learns driving strategies from collected driving datasets based on convolutional neural networks (CNNs). Our scheme can effectively capture comprehensive and dynamic features of traffic flows surrounding the ego car and construct dynamic motion images through processing the spatio-temporal signals of all neighbor cars. These constructed virtual images cover all informative dynamic states of the neighbor cars, located in the maneuver-critical area, defined as the motion-sensitive area (MSA). Then, sequential spatio-temporal images together with labeled driving behaviors are fed into our proposed CNN model. The network can extract underlying motion patterns and learn proper driving behaviors, including both the lateral maneuver and longitudinal speed. We demonstrate the effectiveness of the proposed scheme in a typical highway scenario. Results suggest that the proposed scheme merits further investigation to promote the launch of AVs.
更多查看译文
关键词
Automobiles, Vehicles, Vehicle dynamics, Decision making, Dynamics, Image representation, Behavioral sciences, Spatio-temporal image representation, intelligent decision-making, convolutional neural network, automated vehicles
AI 理解论文
溯源树
样例
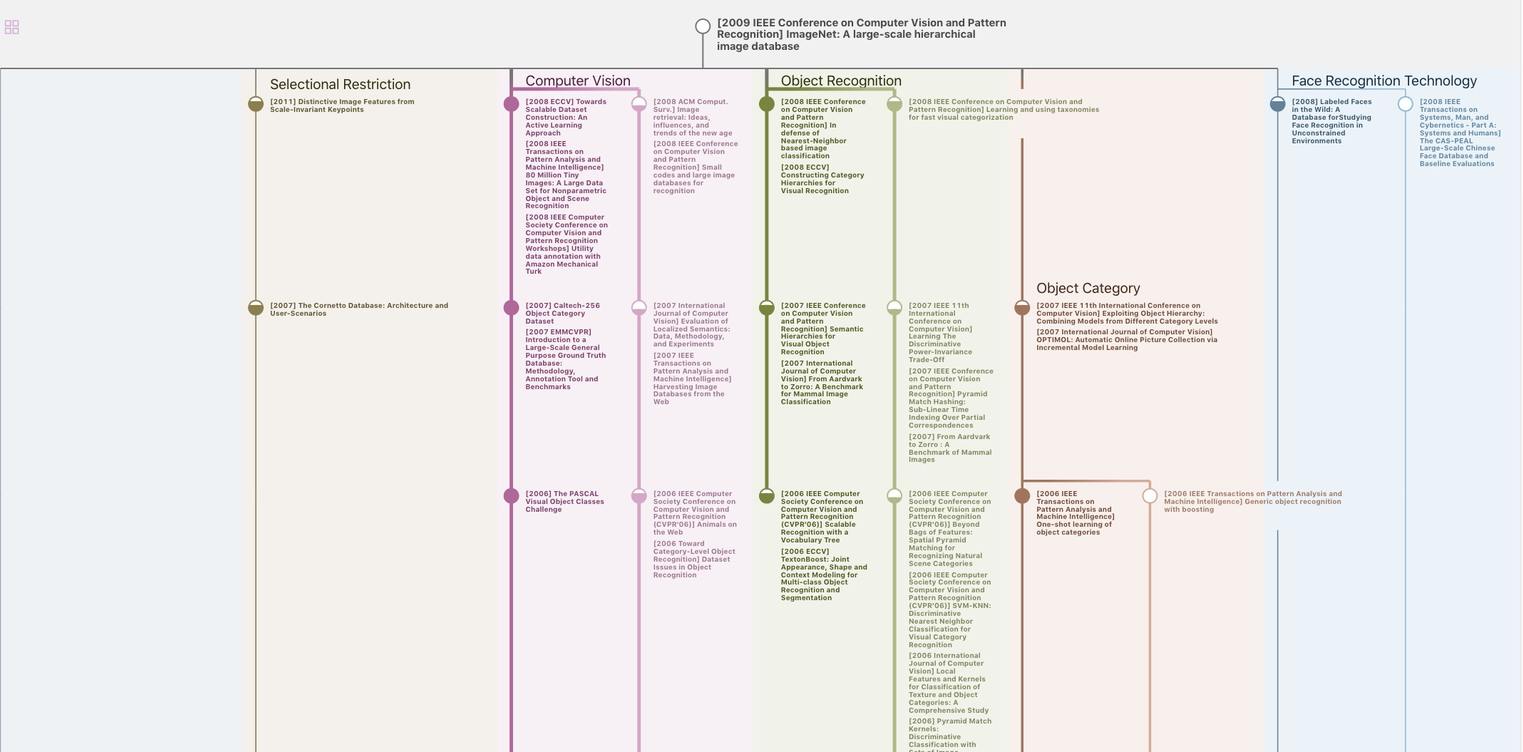
生成溯源树,研究论文发展脉络
Chat Paper
正在生成论文摘要