Instance-Aware Plant Disease Detection by Utilizing Saliency Map and Self-Supervised Pre-Training
AGRICULTURE-BASEL(2022)
摘要
Plant disease detection is essential for optimizing agricultural productivity and crop quality. With the recent advent of deep learning and large-scale plant disease datasets, many studies have shown high performance of supervised learning-based plant disease detectors. However, these studies still have limitations due to two aspects. First, labeling cost and class imbalance problems remain challenging in supervised learning-based methods. Second, plant disease datasets are either unstructured or weakly-unstructured and the shapes of leaves and diseased areas on them are variable, rendering plant disease detection even more challenging. To overcome these limitations, we propose an instance-aware unsupervised plant disease detector, which leverages normalizing flows, a visual saliency map and positional encodings. A novel way to explicitly combine these methods is the proposed model, in which the focus is on reducing background noise. In addition, to better fit the model to the plant disease detection domain and to enhance feature representation, a feature extractor is pre-trained in a self-supervised learning manner using only unlabeled data. In our extensive experiments, it is shown that the proposed approach achieves state-of-the-art performance on widely-used datasets, such as BRACOL (Weakly-unstructured) and PlantVillage (Unstructured), regardless of whether the dataset is weakly-structured or unstructured.
更多查看译文
关键词
computer vision, plant diseases, plant disease detection, normalizing flows, anomaly detection
AI 理解论文
溯源树
样例
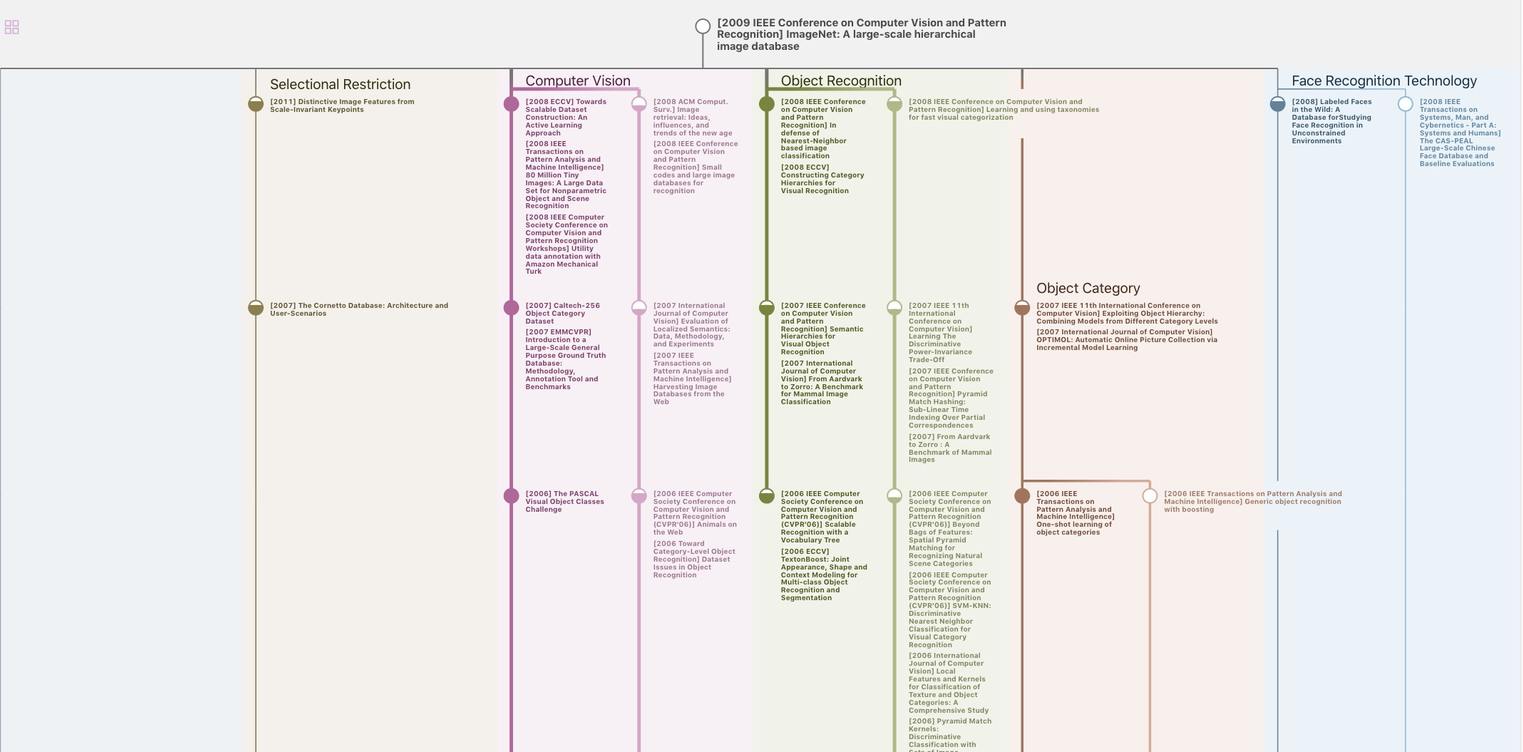
生成溯源树,研究论文发展脉络
Chat Paper
正在生成论文摘要