Improving Hierarchical Short Text Clustering through Dominant Feature Learning
INTERNATIONAL JOURNAL ON ARTIFICIAL INTELLIGENCE TOOLS(2022)
摘要
This paper focuses on the popular problem of short text clustering. Since the short text documents typically exhibit high degrees of data sparseness and dimensionality, the problem in question is generally considered more challenging than the traditional clustering scenarios. Our proposed solution, named VEPH, is based on a novel algorithm that was published recently with the aim of optimally clustering short text documents. VEPH includes two stages: During the first stage, the original text vectors are projected on a lower dimensional space and the documents with projection vectors lying on the same dimensional space are grouped in the same cluster. The second stage is a refinement process which attempts to improve the quality of the clusters that were generated during the previous stage. The quality of a cluster is determined by its homogeneity and completeness and these are the two primary design criteria of this stage. Initially VEPH cleanses the clusters by removing all dissimilar elements, and then, it iteratively merges the similar clusters in a hierarchical agglomerative manner. The proposed algorithm has been experimentally evaluated in terms of F1 and NMI, by employing three datasets with diverse attributes. The results demonstrated its superiority over other state-of-the-art works of the relevant literature.
更多查看译文
关键词
Clustering, short text clustering, machine learning, unsupervised learning
AI 理解论文
溯源树
样例
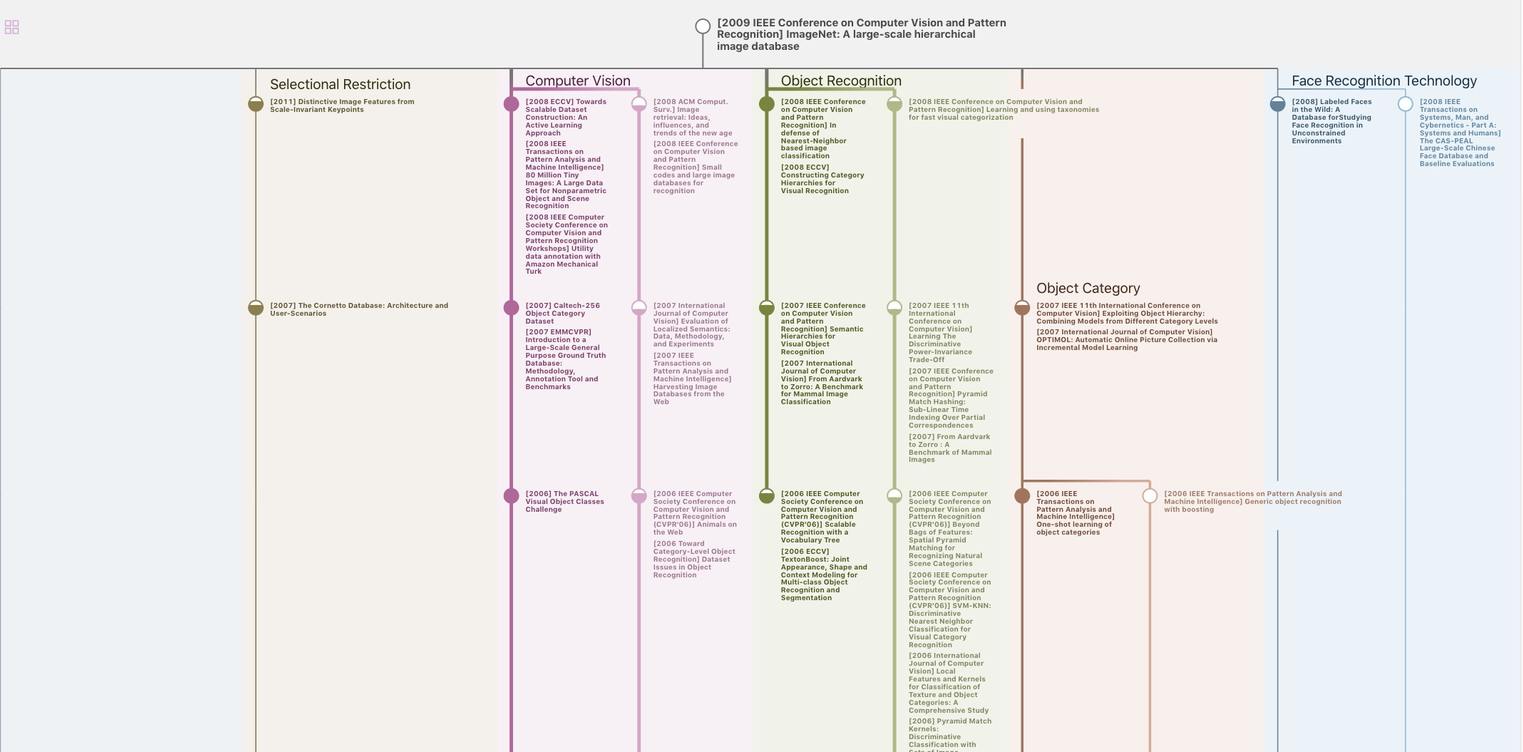
生成溯源树,研究论文发展脉络
Chat Paper
正在生成论文摘要