NATURAL LANGUAGE PROCESSING AND NAMED ENTITY RECOGNITION IN INFLAMMATORY BOWEL DISEASE REFERRALS
GUT(2022)
摘要
Introduction
Clinical natural language processing (NLP) techniques are evolving, such that over the next few years they will start to support clinicians to interpret clinical information. Named entity recognition and linkage (NER+L) to standard ontologies with millions of concepts, such as The Unified Medical Language System (UMLS) add value to otherwise unstructured textual data. However, little research has been done in the field of Inflammatory Bowel Disease (IBD).Methods
Anonymised GP referral letters triaged between 1st January 2017 to 31st March 2021 using an agreed protocol by a panel of Gastroenterologists as likely new or recurrent IBD were randomly extracted. NLP in python was applied to referral free text using MedCAT, a model trained on the UMLS database. Manual validation was performed to determine sensitivity vs ground truth for finding positive mentions of four cardinal clinical signs and symptoms. Sensitivity = TP/(TP + FN) was the outcome of greatest interest. Chi2 was used for statistical comparison at the p<0.05 level.Results:
125 referral letters were included in this study. Median age: 39(IQR:[30-50]), 51.2% Male[95%CI:42.4-60.1]. 22.4%(n=28) of the cohort had pre-existing IBD. Table 1 summarises the performance of the algorithm against the correct human validations: Diarrhoea and abdominal pain were both most mentioned and most successfully detected by MedCAT, however, significant differences were flagged in all cases.Conclusions:
Significant differences were observed between human validations and model predictions for four common IBD signs and symptoms, suggesting that these models are not yet mature enough for use in clinical practice. Annotations for more difficult concepts, such as rectal bleeding and weight loss need to be improved in major open-source NLP corpora.查看译文
关键词
entity recognition,natural language processing
AI 理解论文
溯源树
样例
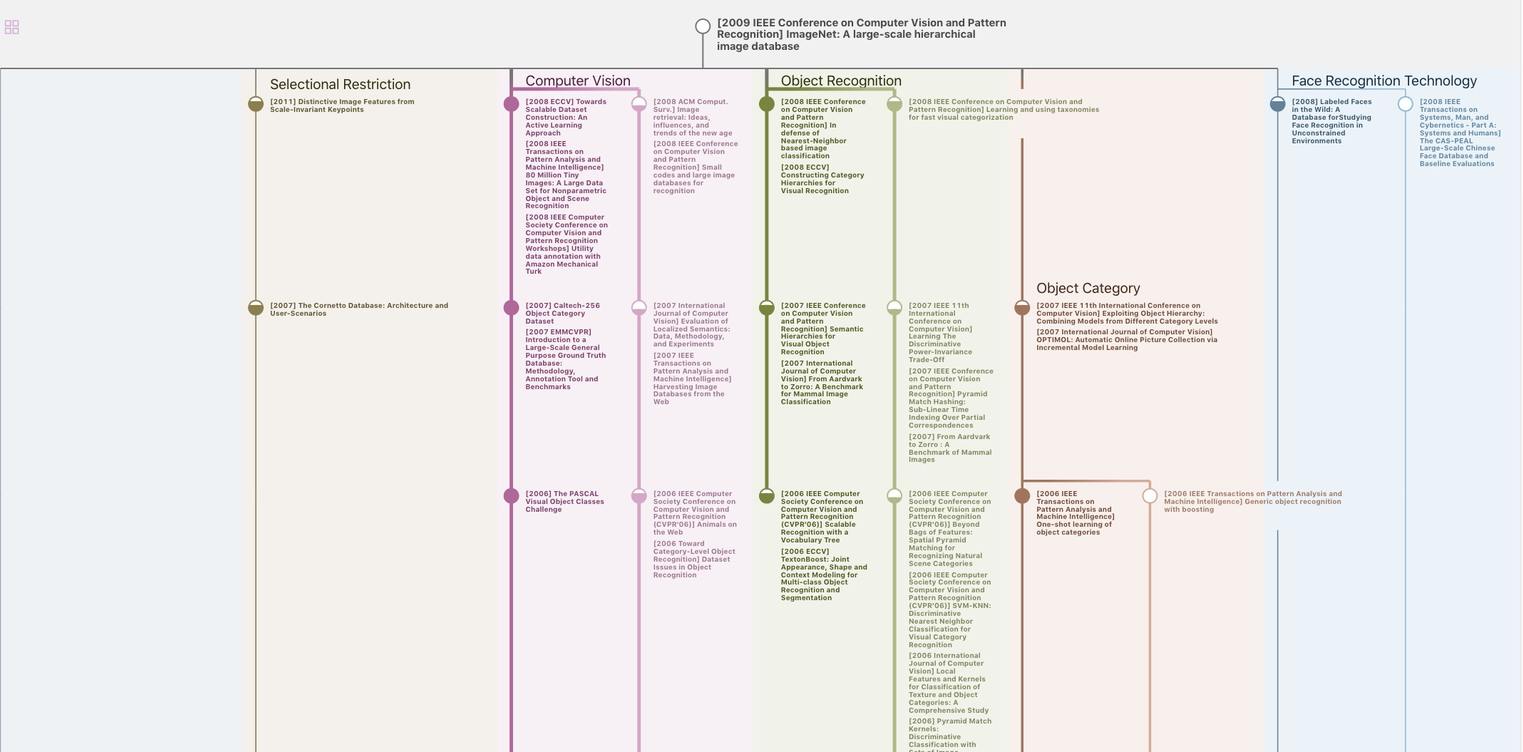
生成溯源树,研究论文发展脉络
Chat Paper
正在生成论文摘要