Probabilistic Digital Twin for Additive Manufacturing Process Design and Control
JOURNAL OF MECHANICAL DESIGN(2022)
摘要
This paper proposes a detailed methodology for constructing an additive manufacturing (AM) digital twin for the laser powder bed fusion (LPBF) process. An important aspect of the proposed digital twin is the incorporation of model uncertainty and process variability. A virtual representation of the LPBF process is first constructed using a physics-based model. To enable faster computation required in uncertainty analysis and decision-making, the physics-based model is replaced by a cheaper surrogate model. A two-step surrogate model is proposed when the quantity of interest is not directly observable during manufacturing. The data collected from the monitoring sensors are used for diagnosis (of current part quality) and passed on to the virtual representation for model updating. The model updating consists of Bayesian calibration of the uncertain parameters and the discrepancy term representing the model prediction error. The resulting digital twin is thus tailored for the particular individual part being produced and is used for probabilistic process parameter optimization (initial, before starting the printing) and online, real-time adjustment of the LPBF process parameters, in order to control the porosity in the manufactured part. A robust design optimization formulation is used to minimize the mean and standard deviation of the difference between the target porosity and the predicted porosity. The proposed methodology includes validation of the digital twin in two stages. Validation of the initial model in the digital twin is performed using available data, whereas data collected during manufacturing are used to validate the overall digital twin.
更多查看译文
关键词
digital twin, additive manufacturing, uncertainty quantification, process design, process control, sensing, model updating, design for manufacturing, simulation-based design, uncertainty analysis, uncertainty modeling
AI 理解论文
溯源树
样例
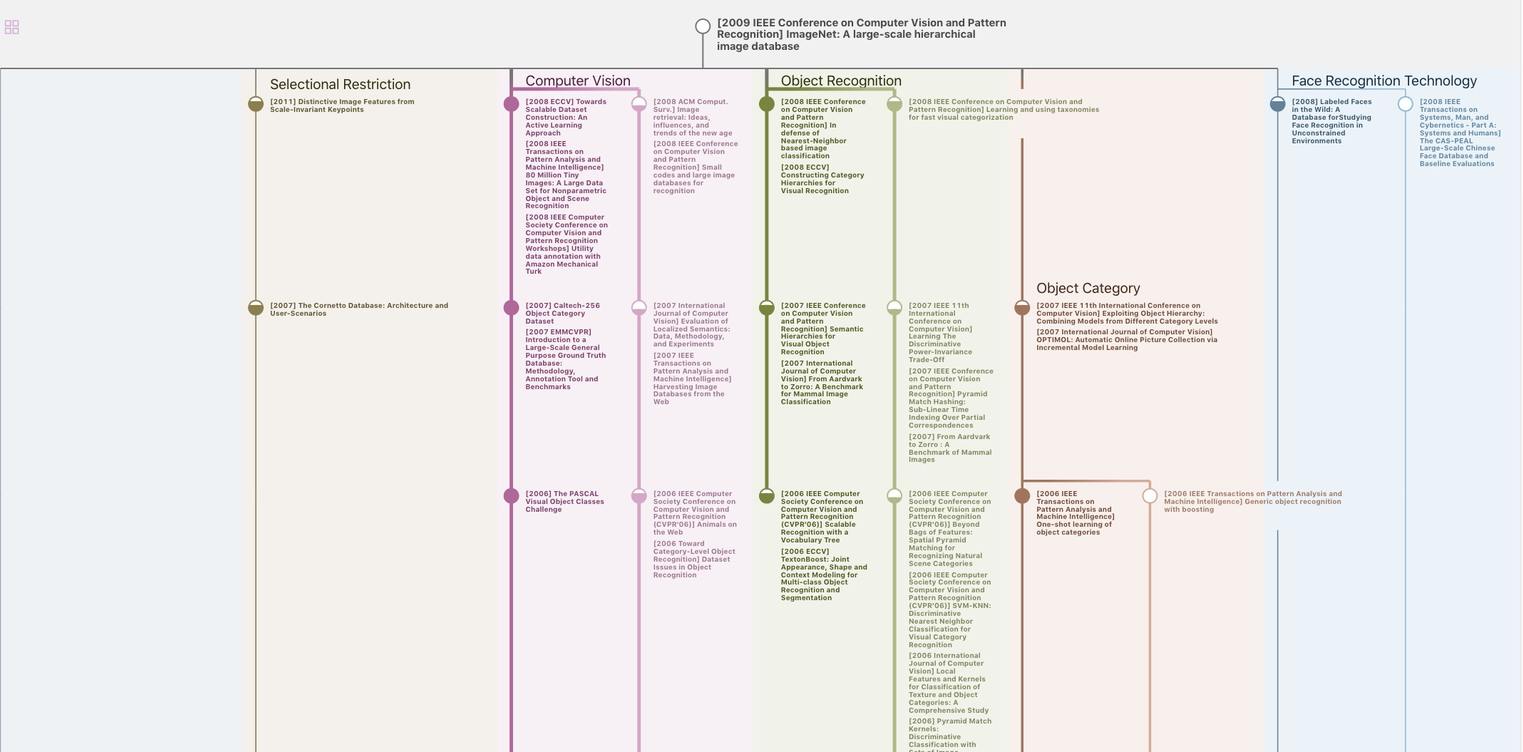
生成溯源树,研究论文发展脉络
Chat Paper
正在生成论文摘要