Multiagent reinforcement learning for autonomous driving in traffic zones with unsignalized intersections
JOURNAL OF INTELLIGENT TRANSPORTATION SYSTEMS(2024)
摘要
In this work we present a multiagent deep reinforcement learning approach for autonomous driving vehicles that is able to operate in traffic networks with unsignalized intersections. The key aspects of the proposed study are the introduction of route-agents as the main building block of the system, as well as a collision term that allows the cooperation among vehicles and the construction of an efficient reward function. These have the advantage of establishing an enhanced collaborative multiagent deep reinforcement learning scheme that manages to control multiple vehicles and navigate them safely and efficiently-economically to their destination. In addition, it provides the beneficial flexibility to lay down a platform for transfer learning and reusing knowledge from the agents' policies in handling unknown traffic scenarios. We provide several experimental results in simulated road traffic networks of variable complexity and diverse characteristics using the SUMO environment that empirically illustrate the efficiency of the proposed multiagent framework.
更多查看译文
关键词
autonomous driving,coordinating vehicles,knowledge reusing,multiagent reinforcement learning,traffic unsignalized intersections
AI 理解论文
溯源树
样例
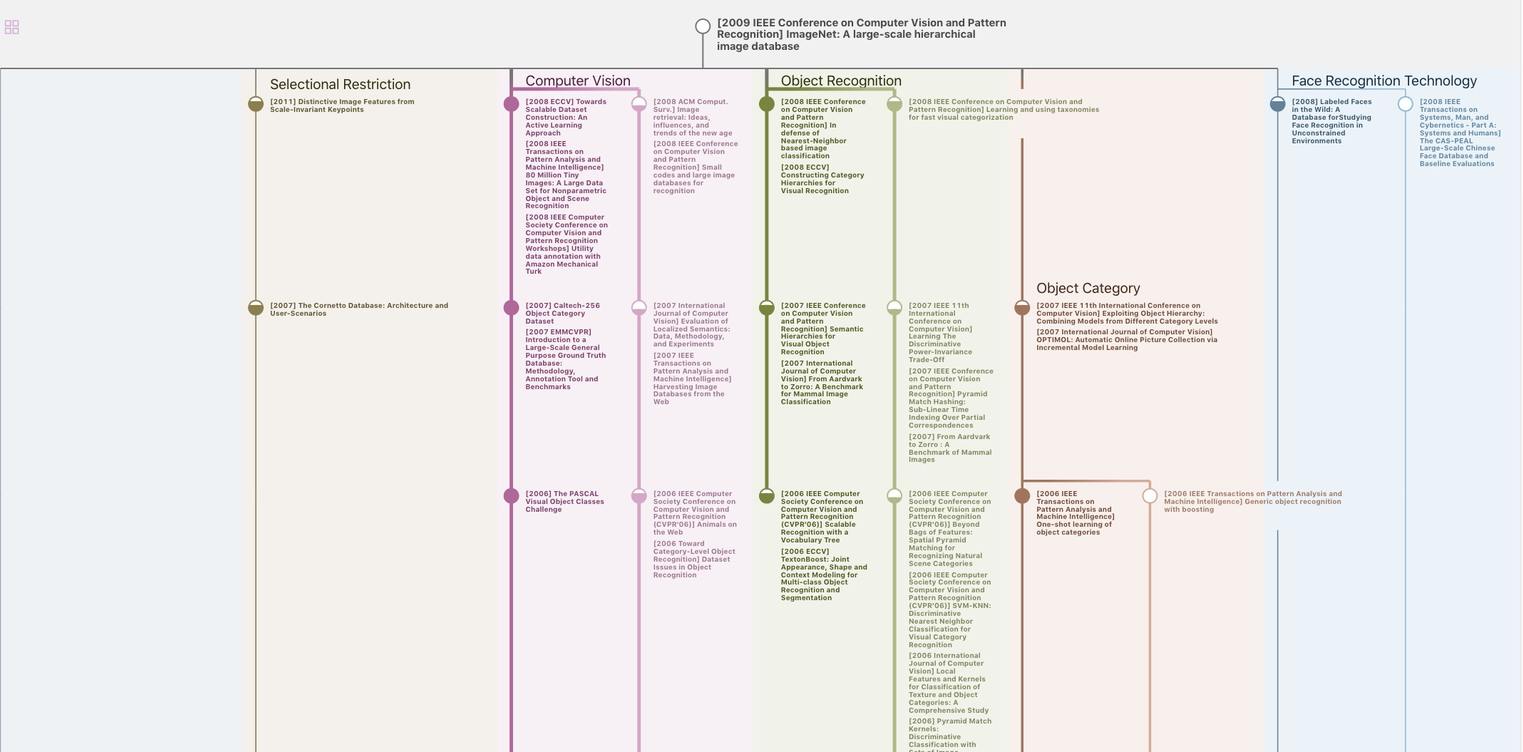
生成溯源树,研究论文发展脉络
Chat Paper
正在生成论文摘要