A Bayesian Approach for Data-Driven Dynamic Equation Discovery
JOURNAL OF AGRICULTURAL BIOLOGICAL AND ENVIRONMENTAL STATISTICS(2022)
摘要
Many real-world scientific and engineering processes are governed by complex nonlinear interactions, and differential equations are commonly used to explain the dynamics of these complex systems. While the differential equations generally capture the dynamics of the system, they impose a rigid modeling structure that assumes the dynamics of the system are known. Even when some of the dynamical relationships are known, rarely do we know the form of the governing equations. Learning these governing equations can improve our understanding of the mechanisms driving the complex systems. Here, we present a Bayesian data-driven approach to nonlinear dynamic equation discovery. The Bayesian framework can accommodate measurement noise and missing data, which are common in these systems, and accounts for model parameter uncertainty. We illustrate our method using simulated data as well as three real-world applications for which dynamic equations are used to study real-world processes. Supplementary materials accompanying this paper appear online.
更多查看译文
关键词
Dynamic discovery uncertainty quantification, Nonlinear dynamic equation, Ordinary differential equation, Probabilistic system discovery, Statistical differential equations
AI 理解论文
溯源树
样例
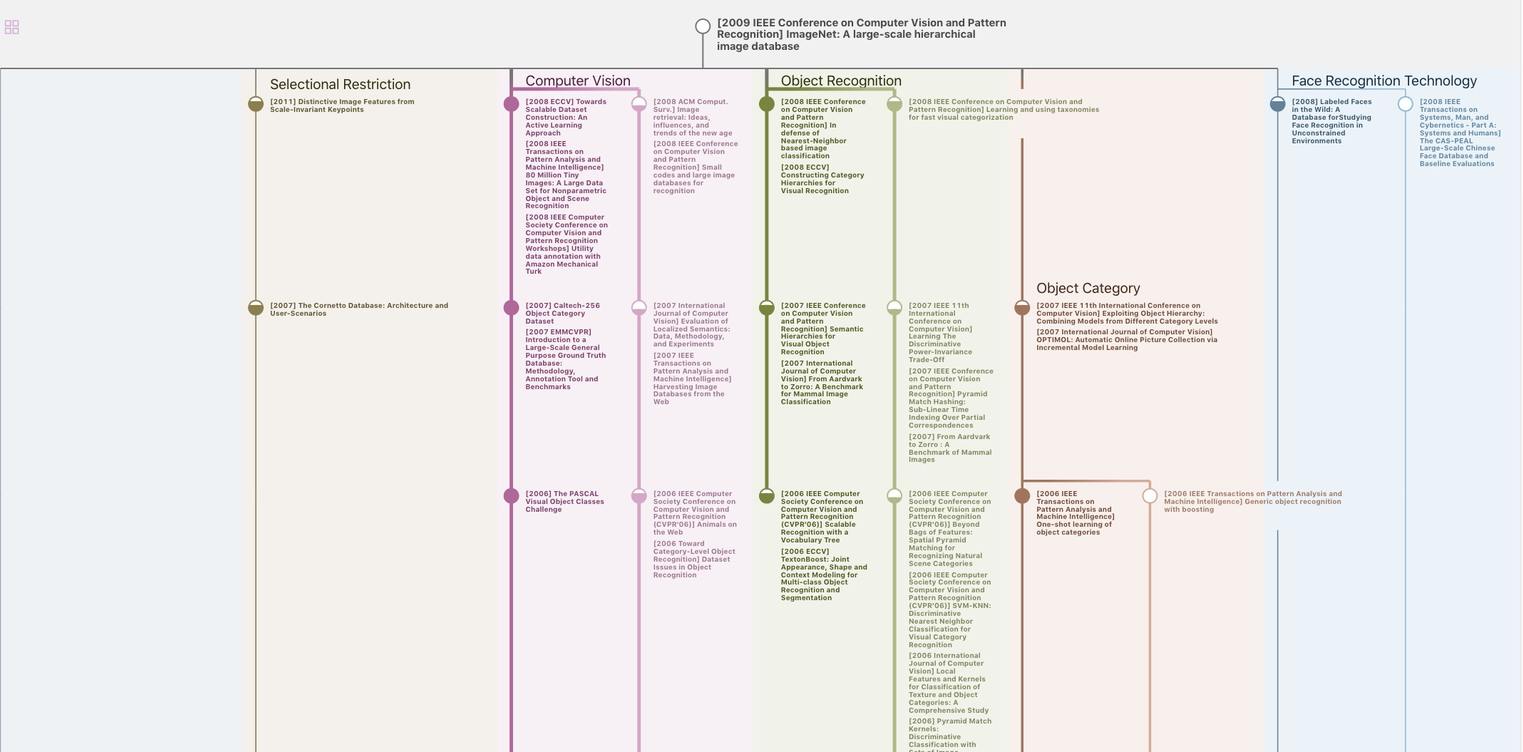
生成溯源树,研究论文发展脉络
Chat Paper
正在生成论文摘要