A traffic flow-forecasting model based on multi-head spatio-temporal attention and adaptive graph convolutional networks
INTERNATIONAL JOURNAL OF MODERN PHYSICS C(2022)
摘要
Accurate traffic flow forecasting is a prerequisite guarantee for the realization of intelligent transportation, but it is a challenging task due to the complex spatial-temporal dependence and uncertainty of traffic flow. In most existing approaches, spatial correlation is captured using graph convolution networks in a pre-determined graph structure. However, some nodes in such a graph structure have spatial correlations between them but are missing a connection, so the hidden spatial correlations between these nodes cannot be captured. Traffic flow has dynamic characteristics, showing different characteristics over time. Most methods ignore the dynamics of traffic flow when modeling the spatio-temporal correlation of traffic flow. We proposed a new network model (MSTA-GCN) to solve the above problem. The model presents a gated adaptive graph convolutional network that captures the hidden spatial correlations between graph nodes from the adaptive. In addition, the model introduces a multi-head spatial-temporal attention mechanism to pay attention to spatial-temporal information of different historical moments and different spatial dimensions to effectively capture the dynamics of spatial-temporal correlation of traffic flow. Extensive experiments were conducted on four datasets of PEMS. The experimental results show that the MSTA-GCN model has better forecasting performance compared with the baseline methods.
更多查看译文
关键词
Traffic flow forecasting, deep learning, multi-head spatio-temporal attention mechanism, graph convolutional network, spatio-temporal correlation
AI 理解论文
溯源树
样例
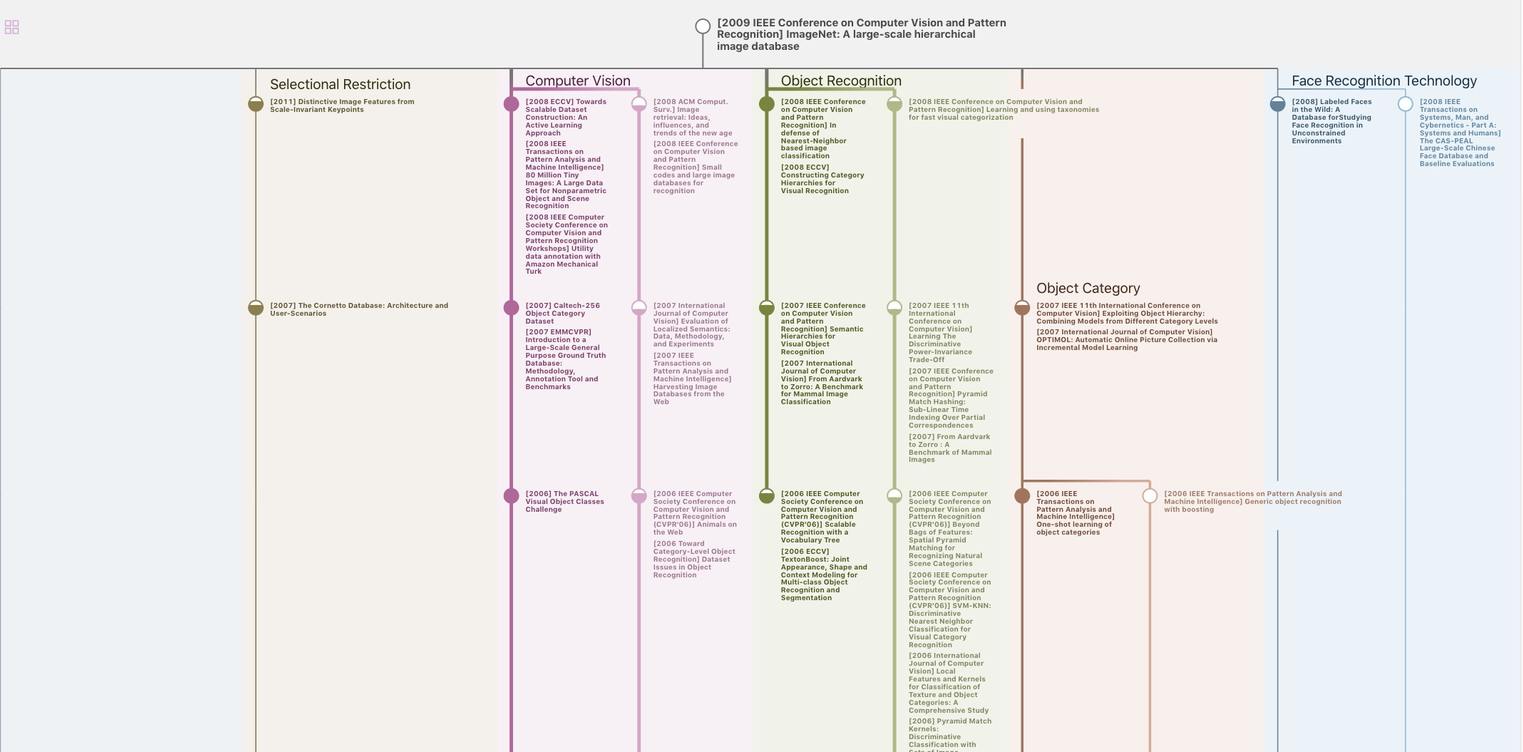
生成溯源树,研究论文发展脉络
Chat Paper
正在生成论文摘要