A novel DAVnet3+ method for precise segmentation of bladder cancer in MRI
VISUAL COMPUTER(2022)
摘要
Muscular invasion is a critical factor in the surgical selection of bladder cancer (BC) patients. Precise segmentation of bladder outer wall (OW), bladder inner wall (IW) and bladder tumor (BT) using MRI is an essential step in the computer-assisted diagnosis of muscle invasiveness in BC. Our study is aimed to develop a novel deep learning-based model to segment the OW, IW and BT, which could effectively aid physicians in BC diagnosis. We proposed a Dual Attention Vnet triple plus (DAVnet3+) network for the accurate segmentation of BC MRI. To fully utilize the feature maps information, full-scale skip connections between down-sampling layers and up-sampling layers were added. Moreover, spatial and channel attention were introduced to increase the weight of the target region, while suppressing the irrelevant background regions for the segmentation task. Two datasets are used to validate the segmentation performance of the proposed DAVnet3+ model. Dataset1 comes from the 2018 China University Computer Design Competition and includes 2200 T2WI BC slices, of which 1760 slices are used for training and 440 slices for testing. Dataset2 contains 1545 T2WI slices provided by the Urology Department of the First Affiliated Hospital of Nanjing Medical University, of which 1236 slices are used for training and 309 slices for testing. Compared to the state-of-the-art deep learning-based methods, the DAVnet3+ model achieves the relatively best segmentation results in terms of dice similarity coefficient (DSC) and average symmetric surface distance (ASSD) on both datasets. (Dataset1: IW: DSC = 0.9626 ± 0.0041, ASSD = 0.3327 ± 0.0363; OW: DSC = 0.8170 ± 0.0266, ASSD = 0.4001 ± 0.0847; BT: DSC = 0.8635 ± 0.0246, ASSD = 0.4884 ± 0.1521. Dataset2: IW: DSC = 0.9840 ± 0.0061, ASSD = 0.2772 ± 0.0268; OW: DSC = 0.8392 ± 0.0188, ASSD = 0.3363 ± 0.0681; BT: DSC = 0.9391 ± 0.0144, ASSD = 0.2921 ± 0.1154). Our findings suggest that DAVnet3+ network is capable of segmenting the OW, IW and BT accurately in T2-weighted MRI. Additionally, it also substantiates the feasibility of the deep learning-based model for multi-region segmentation of BC.
更多查看译文
关键词
Bladder cancer, MRI, Segmentation, Deep learning, Attention mechanism
AI 理解论文
溯源树
样例
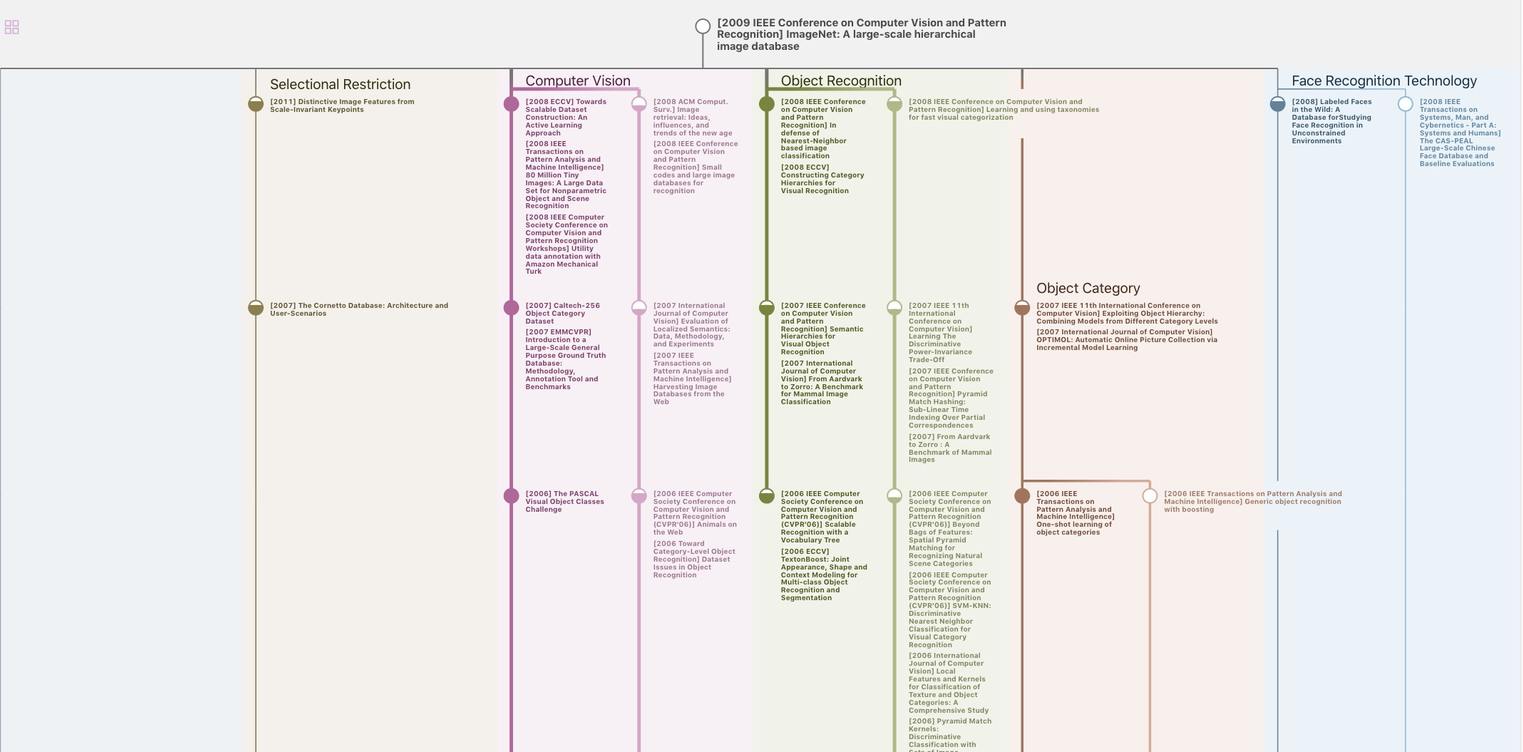
生成溯源树,研究论文发展脉络
Chat Paper
正在生成论文摘要