Early Prediction of All-Cause Clinical Deterioration in General Wards Patients: Development and Validation of a Biomarker-Based Machine Learning Model Derived From Rapid Response Team Activations
JOURNAL OF PATIENT SAFETY(2022)
摘要
Objective The aim of the study is to evaluate the performance of a biomarker-based machine learning (ML) model (not including vital signs) derived from reviewed rapid response team (RRT) activations in predicting all-cause deterioration in general wards patients. Design This is a retrospective single-institution study. All consecutive adult patients' cases on noncritical wards identified by RRT calls occurring at least 24 hours after patient admission, between April 2018 and June 2020, were included. The cases were reviewed and labeled for clinical deterioration by a multidisciplinary expert consensus panel. A supervised learning approach was adopted based on a set of biomarkers and demographic data available in the patient's electronic medical record (EMR). Setting The setting is a 250-bed tertiary university hospital with a basic EMR, with adult (>18 y) patients on general wards. Patients The study analyzed the cases of 514 patients for which the RRT was activated. Rapid response teams were extracted from the hospital telephone log data. Two hundred eighteen clinical deterioration cases were identified in these patients after expert chart review and complemented by 146 "nonevent" cases to build the training and validation data set. Interventions None Measurements and Main Results The best performance was achieved with the random forests algorithm, with a maximal area under the receiver operating curve of 0.90 and F-1 score of 0.85 obtained at prediction time T-0-6h, slightly decreasing but still acceptable (area under the receiver operating curve, >0.8; F-1 score, >0.75) at T-0-42h. The system outperformed most classical track-and-trigger systems both in terms of prediction performance and prediction horizon. Conclusions In hospitals with a basic EMR, a biomarker-based ML model could be used to predict clinical deterioration in general wards patients earlier than classical track-and-trigger systems, thus enabling appropriate clinical interventions for patient safety and improved outcomes.
更多查看译文
关键词
machine learning,clinical deterioration,artificial intelligence,predictive model,clinical decision support
AI 理解论文
溯源树
样例
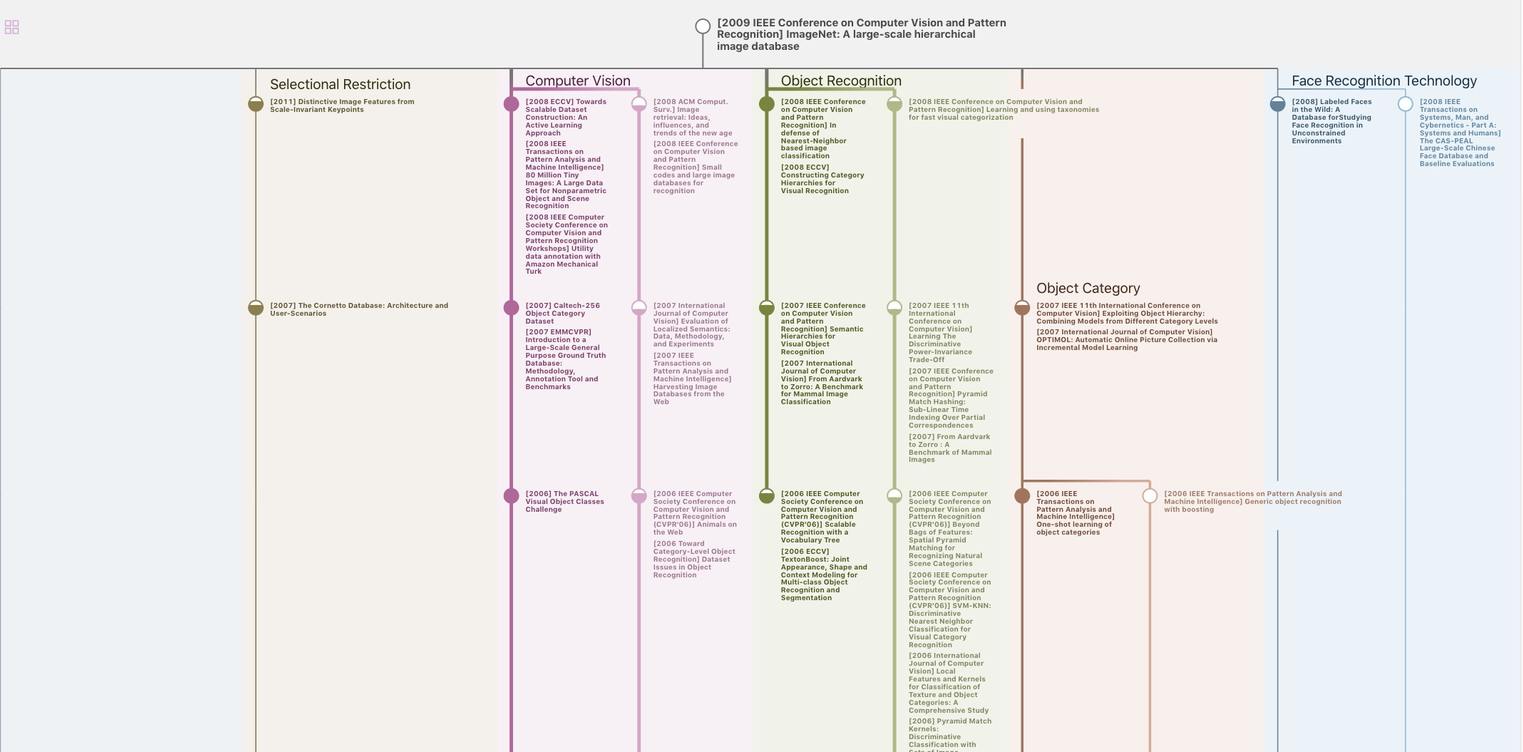
生成溯源树,研究论文发展脉络
Chat Paper
正在生成论文摘要