Integration of global and local information for text classification
NEURAL COMPUTING & APPLICATIONS(2022)
摘要
Text classification is the most fundamental and foundational problem in many natural language processing applications. Recently, the graph-based model (e.g., GNN-based model and GCN-based model) has been applied to this task and achieved excellent performance because of their superior capacity of modeling context from the global perspective. However, a multitude of existing graph-based models constructs a corpus-level graph structure which causes a high memory consumption and overlooks the local contextual information. To address these issues, we present a novel GNN-based model which contains a new model for building a text graph for text classification. The proposed model is called two sliding windows text GNN-based model (TSW-GNN). To be more specific, a unique text-level graph is constructed for each text, which contains a dynamic global window and a local sliding window. The local window slides inside the text to construct local word connections. Additionally, the dynamic global window slides between texts to determine word edge weights, which conquers the limitation of a single local sliding window and provides more abundant global information. We perform extensive experiments on seven benchmark datasets, and the experimental results manifest the amelioration of TSW-GNN over the most advanced models in terms of the classification accuracy.
更多查看译文
关键词
Text classification,Graph neural network,Global information,Local information
AI 理解论文
溯源树
样例
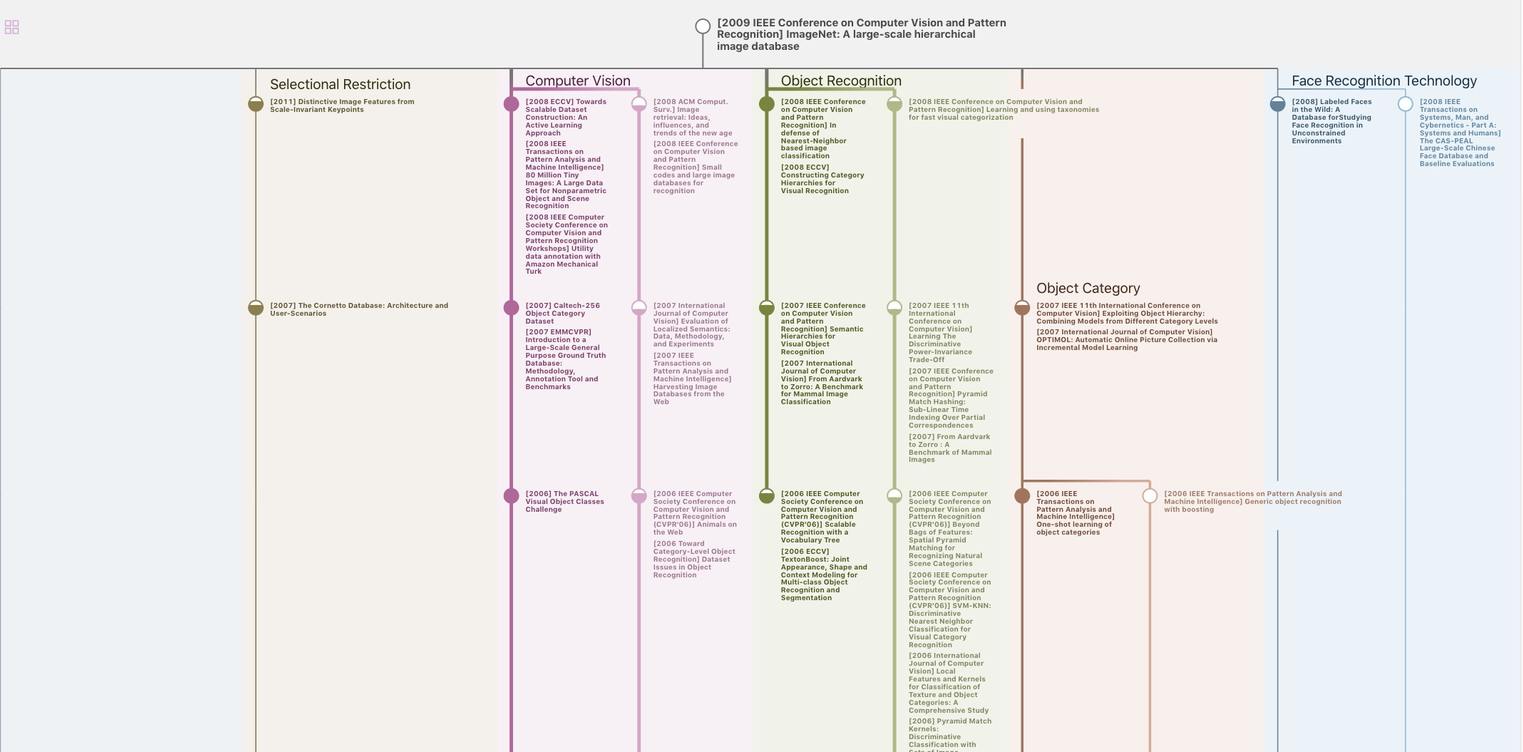
生成溯源树,研究论文发展脉络
Chat Paper
正在生成论文摘要