Infrared Target-Background Separation Based on Weighted Nuclear Norm Minimization and Robust Principal Component Analysis
MATHEMATICS(2022)
摘要
The target detection ability of an infrared small target detection (ISTD) system is advantageous in many applications. The highly varied nature of the background image and small target characteristics make the detection process extremely difficult. To address this issue, this study proposes an infrared patch model system using non-convex (IPNCWNNM) weighted nuclear norm minimization (WNNM) and robust principal component analysis (RPCA). As observed in the most advanced methods of infrared patch images (IPI), the edges, sometimes in a crowded background, can be detected as targets due to the extreme shrinking of singular values (SV). Therefore, a non-convex WNNM and RPCA have been utilized in this paper, where varying weights are assigned to the SV rather than the same weights for all SV in the existing nuclear norm minimization (NNM) of IPI-based methods. The alternate direction method of multiplier (ADMM) is also employed in the mathematical evaluation of the proposed work. The observed evaluations demonstrated that in terms of background suppression and target detection proficiency, the suggested technique performed better than the cited baseline methods.
更多查看译文
关键词
infrared search (IRST) and track system, RPCA, NNM, IPI, signal to clutter ratio (SCR), SCR gain (SCRG)
AI 理解论文
溯源树
样例
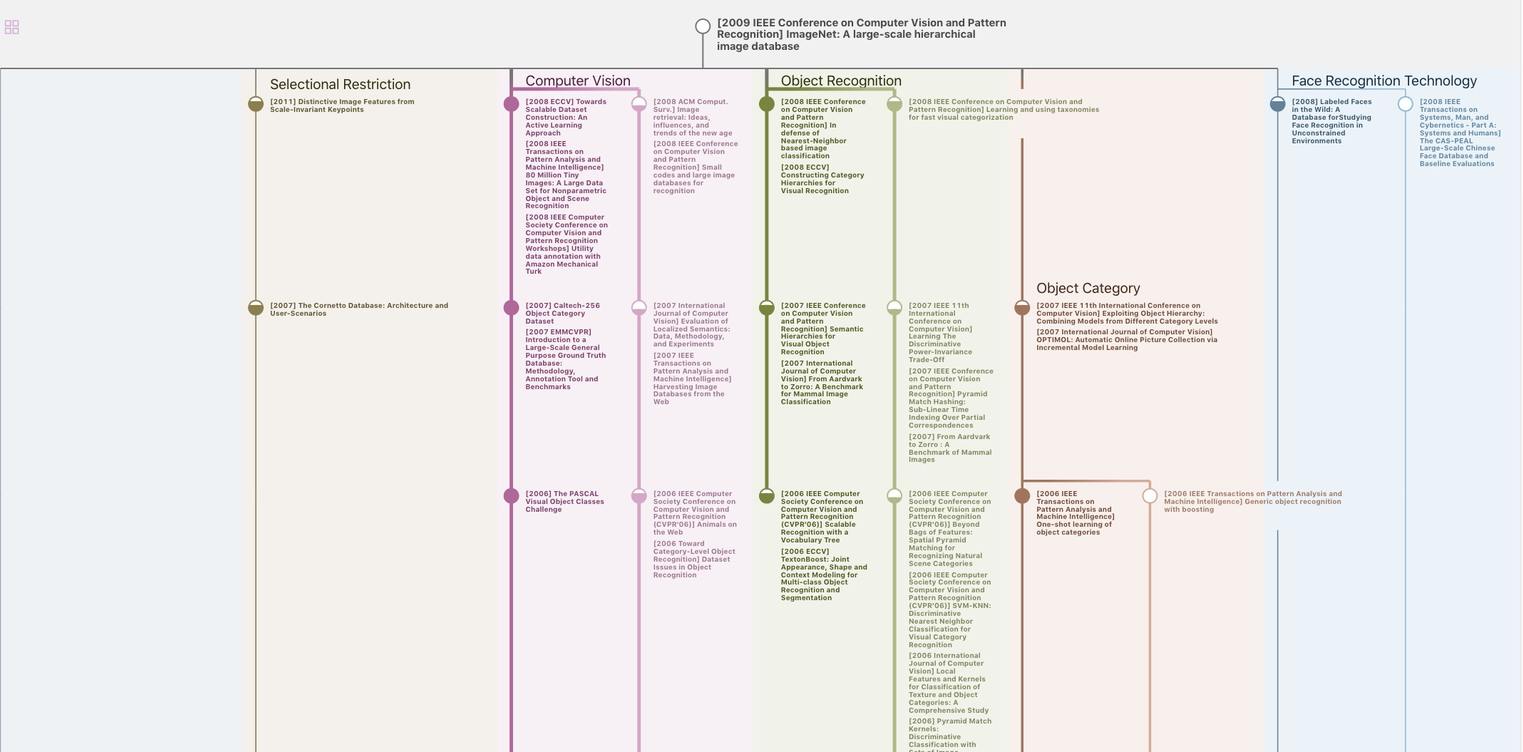
生成溯源树,研究论文发展脉络
Chat Paper
正在生成论文摘要