TGNET: A TASK-GUIDED NETWORK ARCHITECTURE FOR MULTI-ORGAN AND TUMOUR SEGMENTATION FROM PARTIALLY LABELLED DATASETS
2022 IEEE INTERNATIONAL SYMPOSIUM ON BIOMEDICAL IMAGING (IEEE ISBI 2022)(2022)
摘要
The cost of labour and expertise makes it challenging to collect a large amount of medical data and annotate 3D medical images at a voxel level. Most public medical datasets are only labelled with one type of organ or tumour. For example, in the liver segmentation task, only the liver is labelled while all the other organs, even when present, as well as irrelevant parts are annotated as background, resulting in partially labelled datasets. Current popular methods usually build multiple neural network models for different tasks that lead to great model redundancy, or design a unified network for all tasks which suffers from low ability to extract task-related features. In this paper, we propose a unified and task-guided network architecture to efficiently learn task-related features and avoid mixing representations of different organs and tumours from different tasks. Specifically, a novel residual block and attention module are devised in a task-guided way to fuse image features and task encoding constraints. Moreover, both designs significantly suppress task-unrelated features and highlight features related to the specific segmentation task. Experiments conducted on seven benchmark datasets illustrate that our task-guided model achieves more competitive performance compared with state-of-the-art approaches in segmenting multiple organs and tumours from partially labelled data.
更多查看译文
关键词
task-guided attention module, multiple organs and tumours, partially-labelled datasets, unified model
AI 理解论文
溯源树
样例
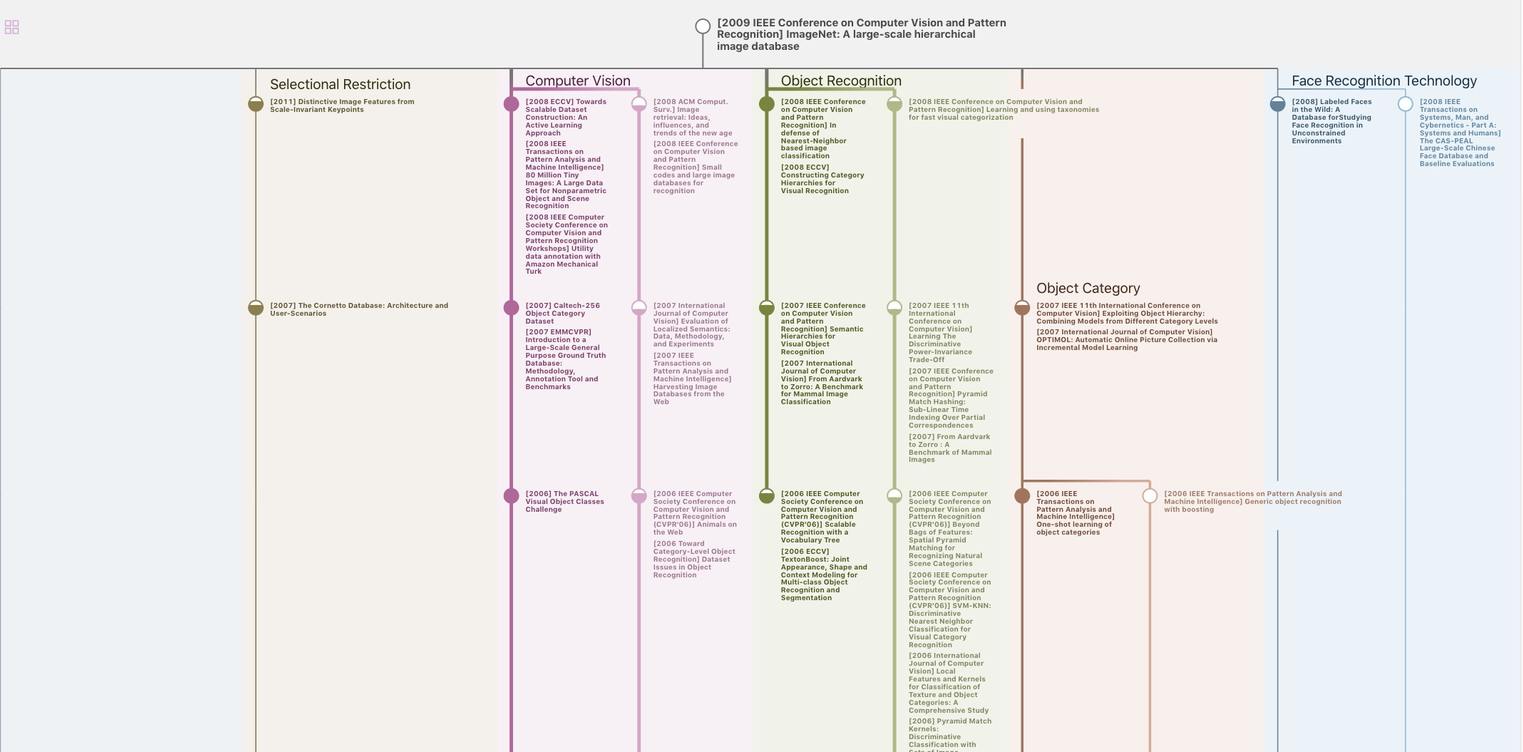
生成溯源树,研究论文发展脉络
Chat Paper
正在生成论文摘要