OMNI-SUPERVISED DOMAIN ADVERSARIAL TRAINING FOR WHITE MATTER HYPERINTENSITY SEGMENTATION IN THE UK BIOBANK
2022 IEEE INTERNATIONAL SYMPOSIUM ON BIOMEDICAL IMAGING (IEEE ISBI 2022)(2022)
摘要
White matter hyperintensities (WMHs, or lesions) appear as hyperintense, localized regions on T2-weighted and FLAIR brain MR images. The heterogeneity in lesion characteristics due to subject-level (e.g., local intensity/contrast) and population-level (e.g., demographic, scanner-related) variations make their segmentation highly challenging. Here, we propose a framework for adapting a state-of-the-art WMH segmentation method with high accuracy from a small, labeled source data (MICCAI WMH segmentation challenge 2017 training data) to a larger dataset such as the UK Biobank without the need of additional manual training labels, using domain adversarial training with omni-supervised learning. Given the well-known association of WMHs with age, the proposed method uses a multi-tasking model for learning lesion segmentation, domain adaptation and age prediction simultaneously. On a subset of the UK Biobank dataset, the proposed method achieves a lesion-level recall, lesion-level F1-measure and Dice overlap value of 0.95, 0.65 and 0.84 respectively, when compared to values of 0.75, 0.49 and 0.80 obtained from the pretrained state-of-the-art baseline method. The code for the method is available at https://github.com/vsundaresan/omnisup_agepred_semidann.
更多查看译文
关键词
UK Biobank, omni-supervised learning, white matter hyperintensities, domain adaptation, adversarial training
AI 理解论文
溯源树
样例
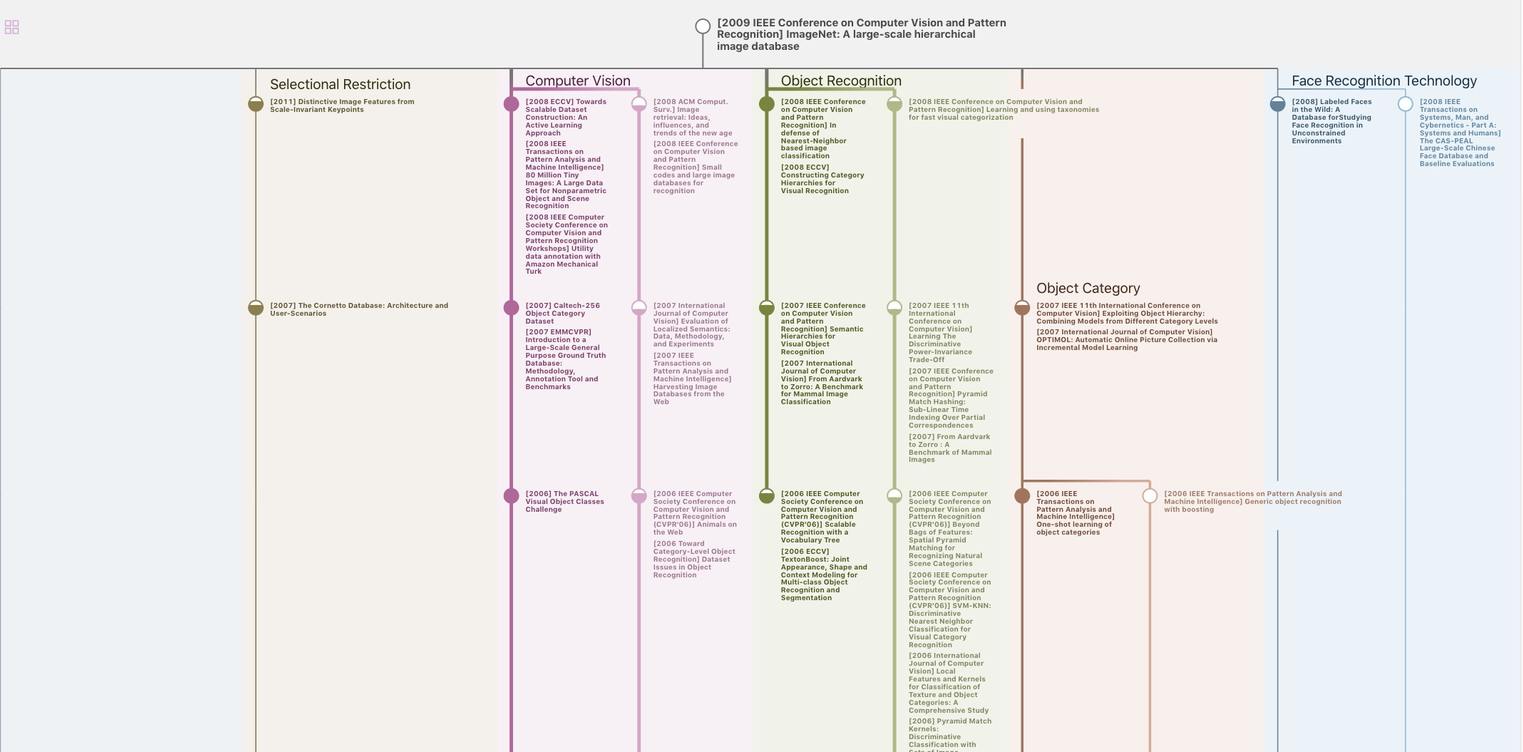
生成溯源树,研究论文发展脉络
Chat Paper
正在生成论文摘要