Effect of computerized decision support on diagnostic accuracy and intra-observer variability in multi-institutional observer performance study for bladder cancer treatment response assessment in CT urography
MEDICAL IMAGING 2022: COMPUTER-AIDED DIAGNOSIS(2022)
摘要
We have previously developed a computerized decision support system for bladder cancer treatment response assessment (CDSS-T) in CT urography (CTU). In this work, we conducted an observer study to evaluate the diagnostic accuracy and intra-observer variability with and without the CDSS-T system. One hundred fifty-seven pre- and post-treatment lesion pairs were identified in pre- and post-chemotherapy CTU scans of 123 patients. Forty lesion pairs had T0 stage (complete response) after chemotherapy. Multi-disciplinary observers from 4 different institutions participated in reading the lesion pairs, including 5 abdominal radiologists, 4 radiology residents, 5 oncologists, 1 urologist, and 1 medical student. Each observer provided estimates of the T0 likelihood after treatment without and then with the CDSS-T aid for each lesion. To assess the intra-observer variability, 51 cases were evaluated two times - the original and the repeated evaluation. The average area under the curve (AUC) of 16 observers for estimation of T0 disease after treatment increased from 0.73 without CDSS-T to 0.77 with CDSS-T (p = 0.003). For the evaluation with CDSS-T, the average AUC performance for different institutions was similar. The performance with CDSS-T was improved significantly and the AUC standard deviations were slightly smaller showing potential trend of more accurate and uniform performance with CDSS-T. There was no significant difference between the original and repeated evaluation. This study demonstrated that our CDSS-T system has the potential to improve treatment response assessment of physicians from different specialties and institutions, and reduce the inter- and intra-observer variabilities of the assessments.
更多查看译文
关键词
observer study,computer-aided diagnosis,bladder cancer,treatment response assessment,deep learning,radiomics
AI 理解论文
溯源树
样例
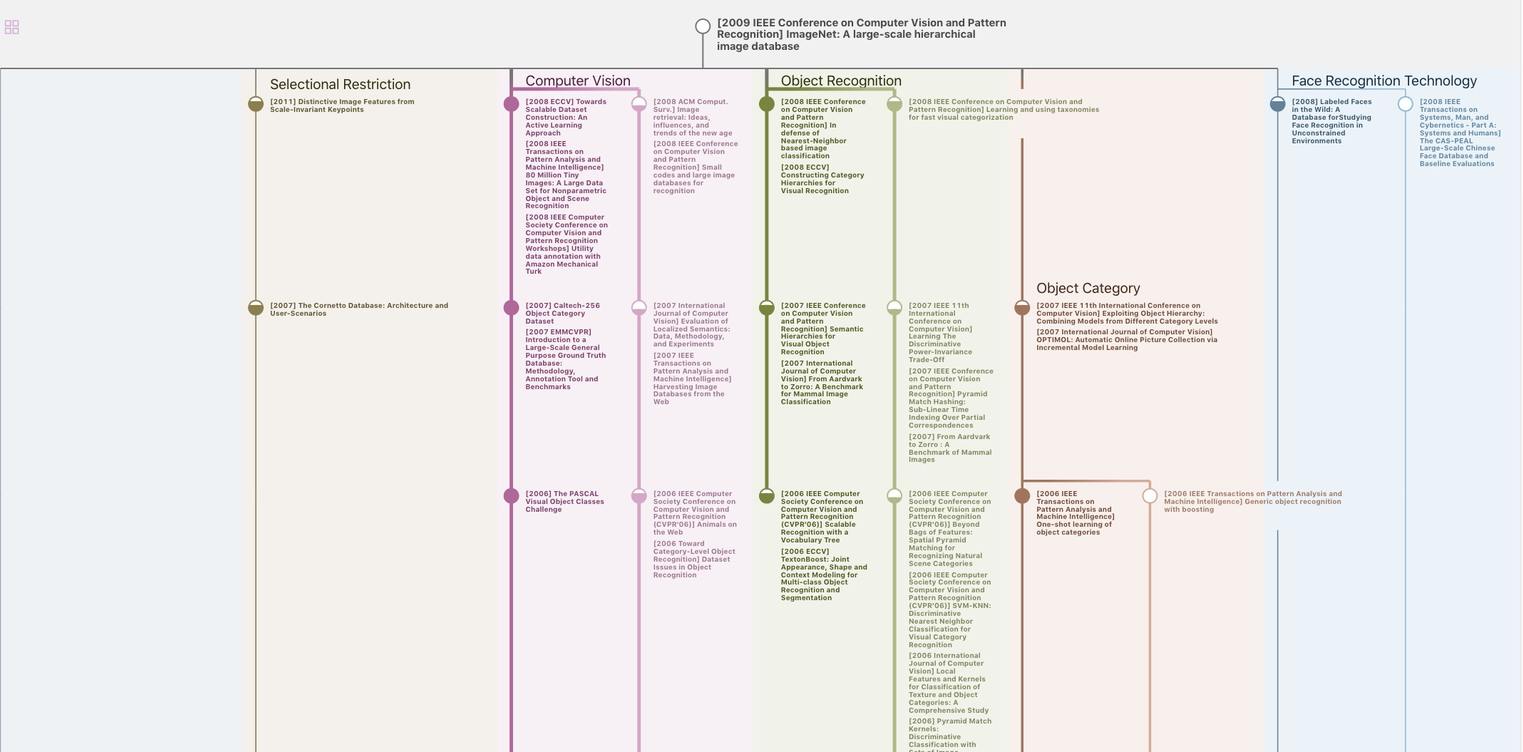
生成溯源树,研究论文发展脉络
Chat Paper
正在生成论文摘要