Regional Infrasonic and Seismic Event Classification with Machine Learning
CHEMICAL, BIOLOGICAL, RADIOLOGICAL, NUCLEAR, AND EXPLOSIVES (CBRNE) SENSING XXIII(2022)
摘要
The present work details how convolutional and recurrent deep learning networks can be used to classify infrasonic and seismic events of regional-field rocket demolitions, rocket motor burns, quarry blasts, and earthquakes. To accelerate machine learning adoption within the geophysical sciences, we illustrate the full machine learning pipeline: data acquisition and cleaning, preprocessing, model construction and training, and model understanding using feature-space analysis. Multiple deep learning architectures are evaluated to provide practical lessons learned and insights. The LSTM-RNN suffers from degraded learning on long geophysical signals, while a CNN is more robust to variance in data quality and length. Geophysical time-series signals should be learned with the instrument response deconvolved to avoid gross resampling or decimation of the signal. Frequency-domain feature inputs like spectrograms exhibit improved classification performance, and mapping event-based attributes to the learned feature space of deep networks can provide explainable physical context. All network configurations are validated on geophysical data collected in the Utah region, with experimental results including ablation studies examining different input types, preprocessing strategies, and hyperparameter settings.
更多查看译文
关键词
Infrasound, Seismic, Time-Series Classification, Machine Learning, Deep Learning, Neural Networks
AI 理解论文
溯源树
样例
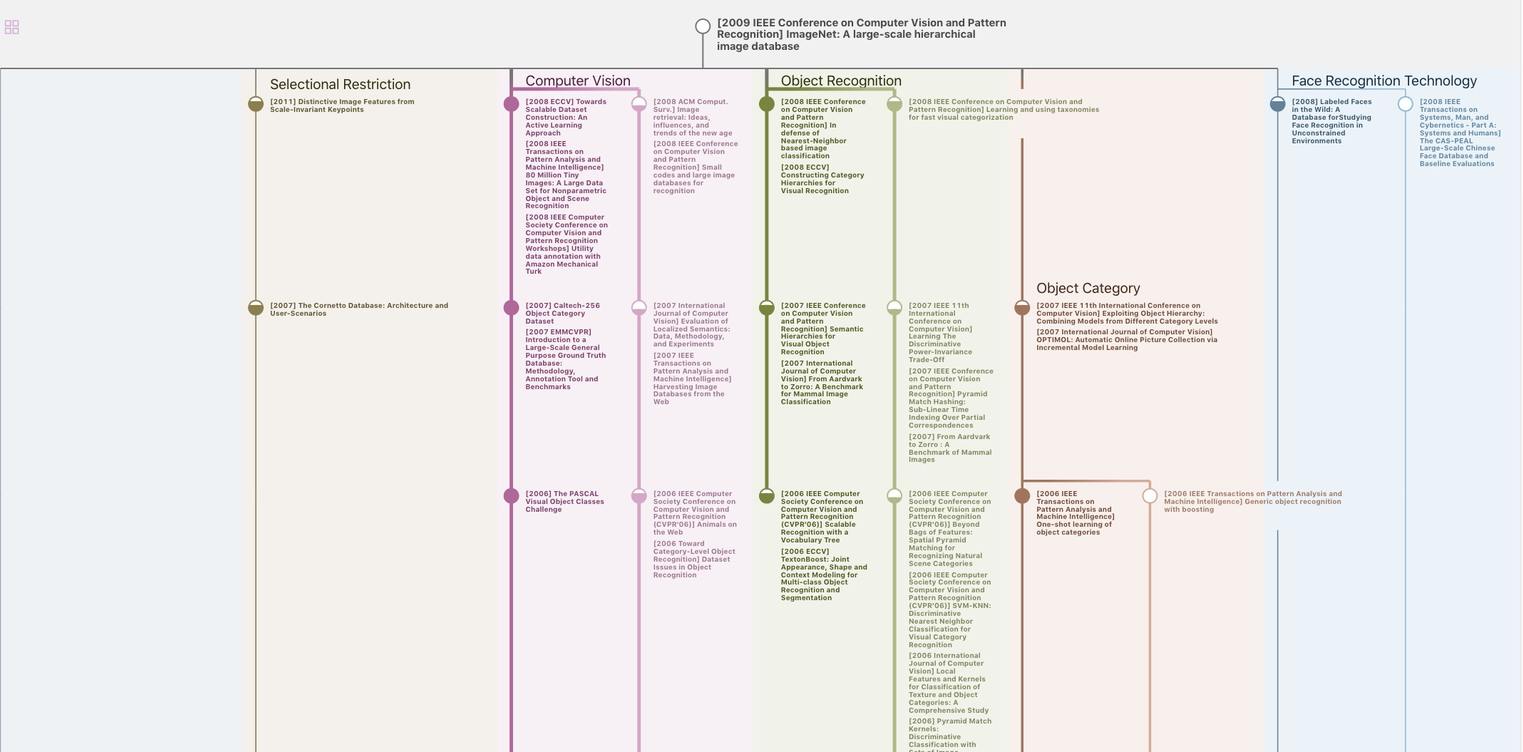
生成溯源树,研究论文发展脉络
Chat Paper
正在生成论文摘要