Deciphering deep ensembles for lung nodule analysis
MEDICAL IMAGING 2022: COMPUTER-AIDED DIAGNOSIS(2022)
摘要
Empirical ensembles of deep convolutional neural network (DNN) models have been shown to outperform individual DNN models as seen in the last few ImageNet challenges. Several studies have also shown that ensemble DNNs are robust against out-of-sample data, making it an ideal approach for machine learning-enabled medical imaging tasks. In this work, we analyze deep ensembles for the task of classifying true and false lung nodule candidates in computed tomography (CT) volumes. Six ImageNet pretrained DNN models with minimal modifications for lung nodule classification were used to generate 63 ensemble DNNs using all possible combinations of DNN models. The checkpoint predictions during the training of the DNN models were used as a surrogate to understand the training trajectories each model took to result in the finalized model. The predictions from each checkpoint across the six DNN models were projected to a 2-dimensional space using uniform manifold approximation and projection method. The output scores from these six models were compared using a rank-biased overlap measure that incorporates larger weights for top scoring candidates and the ability to handle arbitrary sized list of candidates. Both these analyses indicate that diversity in the training process leads to diversity of the scores for the same training and test samples. The competition performance metric (CPM) from the free-response operating characteristic curve shows that as the number of DNN models in each ensemble increases, the CPM increases from an average of 0.750 to 0.79.
更多查看译文
关键词
ensemble,lung nodule,transfer learning,deep-learning,artificial intelligence,deep convolutional neural network
AI 理解论文
溯源树
样例
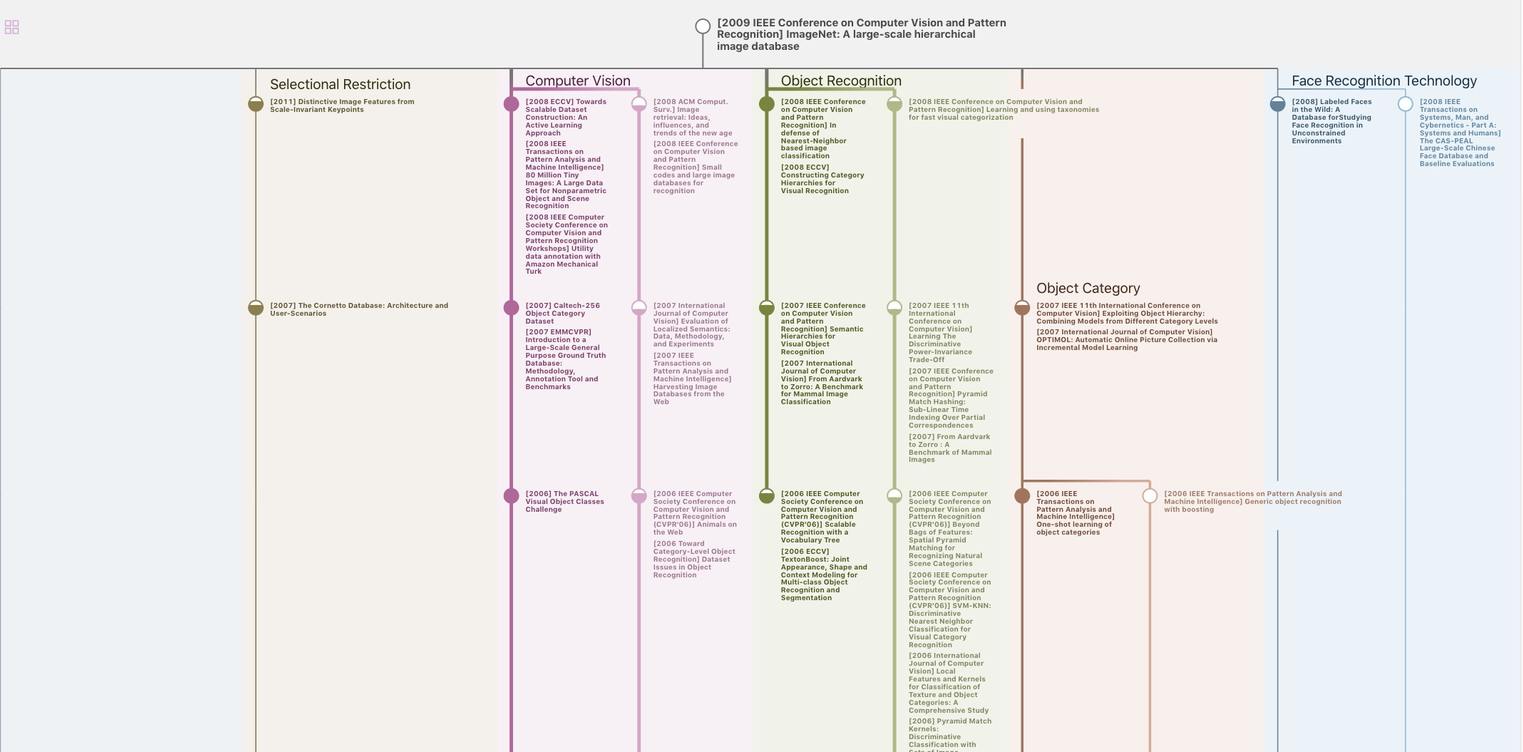
生成溯源树,研究论文发展脉络
Chat Paper
正在生成论文摘要