Automatic Flagging of AI Segmentation Errors in Computational Pathology
MEDICAL IMAGING 2022: DIGITAL AND COMPUTATIONAL PATHOLOGY(2022)
摘要
Active Learning (AL) is an artificial intelligence (AI) training paradigm that improves training efficiency in cases where labeled training is hard to obtain. In AL, unlabeled samples are selected for annotation using a bootstrap classifier to identify samples whose informational content is not represented in the current training set. Given a small number of samples, this optimizes training by focusing annotation on "informative" samples. For computational pathology, identifying the most-informative samples is non-trivial, particularly for segmentation. In this work, we develop a feature-driven approach to identifying informative samples. We use a feature extraction pipeline operating on segmentation results to find "outlier" samples which are likely incorrectly segmented. This process allows us to automatically flag samples for re-annotation based on architecture of segmentation (compared with less robust confidence-based approaches). We apply this process to the problem of segmenting oral cavity cancer (OCC) H&E stained whole-slide images (WSIs), where the architecture of OCC tumor growth is an aggressive pathological indicator. Improving segmentation requires costly annotation of WSIs; thus, we seek to employ an AL approach to improve annotation efficiency. Our results show that, while outlier features alone are not sufficient to flag samples for re-annotation, we can identify some WSIs which fail segmentation.
更多查看译文
关键词
Oral Cavity Cancer, Active Learning, Computer Vision, Quantitative Architectural Features
AI 理解论文
溯源树
样例
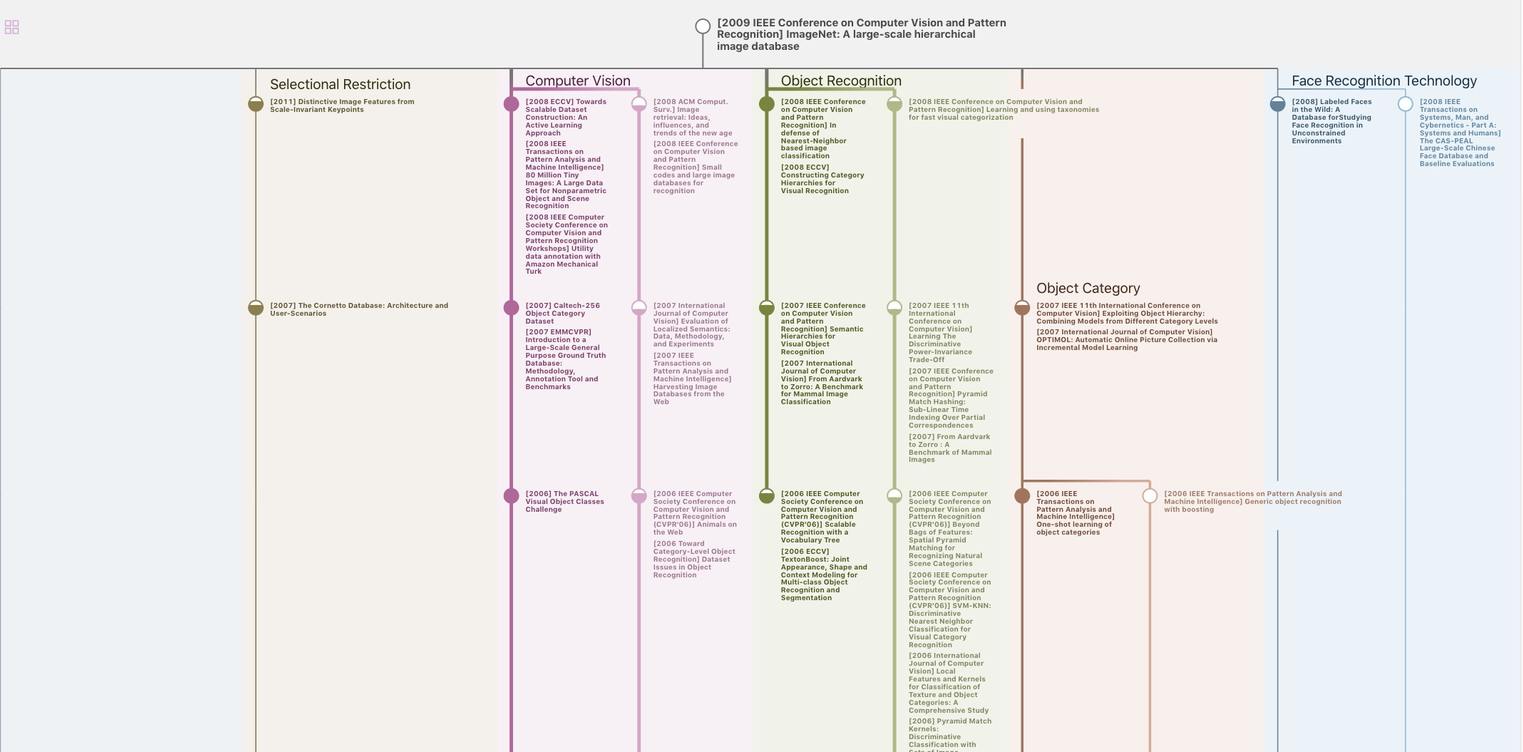
生成溯源树,研究论文发展脉络
Chat Paper
正在生成论文摘要