Double-Branch Local Context Feature Extraction Network for Hyperspectral Image Classification
IEEE GEOSCIENCE AND REMOTE SENSING LETTERS(2022)
摘要
In recent years, deep learning methods have made great progress in hyperspectral image (HSI) classification. However, the current models obtain deep-seated global feature by deepening the number of network layers and ignore the local neighborhood environment. To solve this problem, we propose a double-branch network model, which extracts spatial and spectral local context features, respectively, fuses different levels of feature adaptively, and has strong ability to aggregate context information. Specifically, this model uses self-calibrated convolution to extract spatial information and multiscale dense convolution to extract spectral information. We propose that local context feature extraction module (LCFM) makes full use of the background information and mutual information between positions of each pixel to obtain local context feature information. In addition, focal loss is used to solve the problem of different classification difficulty of each sample. Simulation results showed that the proposed module not only achieves high classification accuracy but also greatly reduces the amount of calculation and parameters.
更多查看译文
关键词
Feature extraction, Convolution, Data mining, Training, Correlation, Kernel, IP networks, Double-branch, hyperspectral image (HSI) classification, local context feature extraction, multiscale DenseNet, self-calibrated convolution
AI 理解论文
溯源树
样例
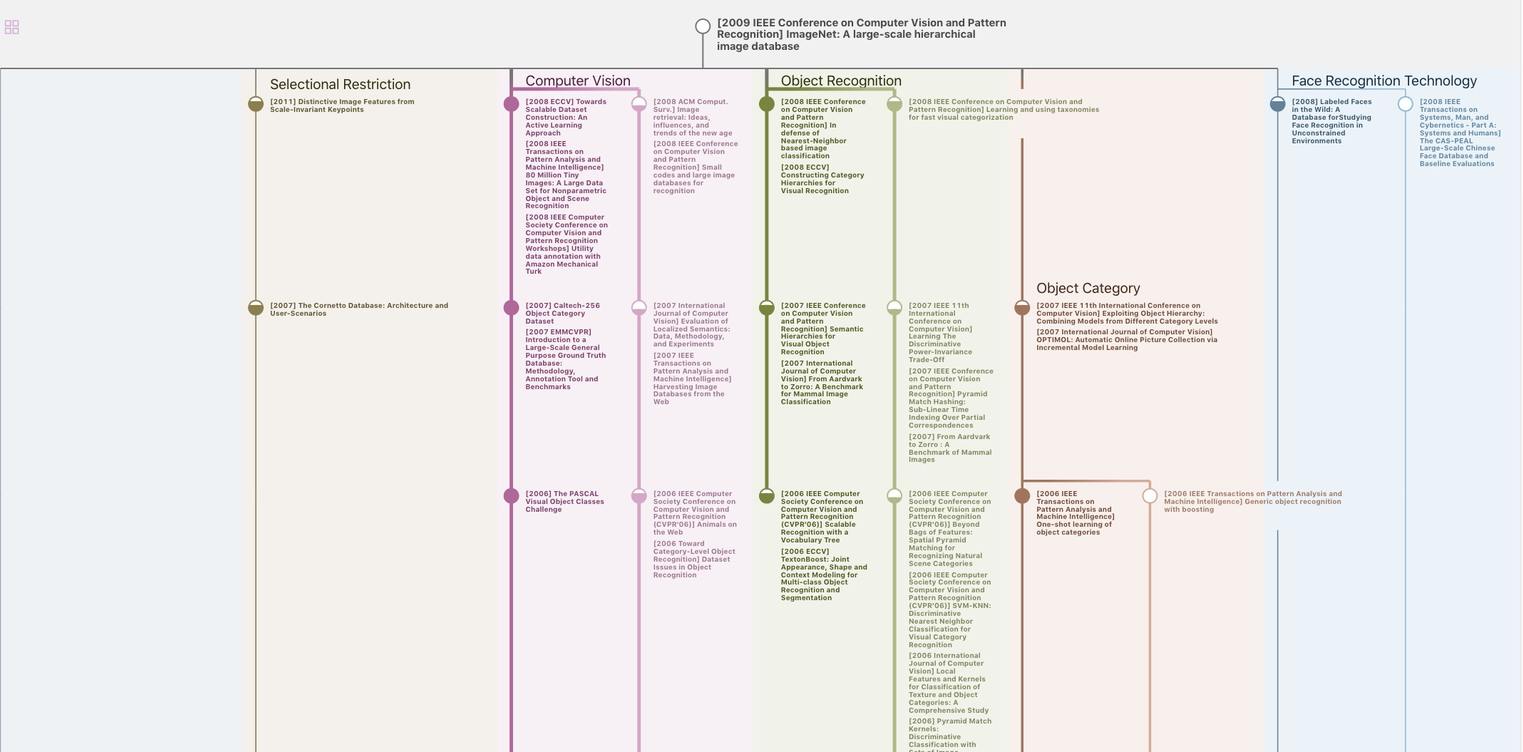
生成溯源树,研究论文发展脉络
Chat Paper
正在生成论文摘要