Learning Image Formation and Regularization in Unrolling AMP for Lensless Image Reconstruction
IEEE Transactions on Computational Imaging(2022)
摘要
This paper proposes an unrolling learnable approximate message passing recurrent neural network (called ULAMP-Net) for lensless image reconstruction. By unrolling the optimization iterations, key modules and parameters are made learnable to achieve high reconstruction quality. Specifically, observation matrices are rectified on the fly through network learning to suppress systematic errors in the measurement of the point spread function. We devise a domain transformation structure to achieve a more powerful representation and propose a learnable multistage threshold function to accommodate a much richer family of priors with only a small amount of parameters. Finally, we introduce a multi-layer perceptron (MLP) module to enhance the input and an attention mechanism as an output module to refine the final results. Experimental results on display captured dataset and real scene data demonstrate that, compared with the state-of-the-art methods, our method achieves the best reconstruction quality with low computational complexity and the tiny model size on the testing set. Our code will be released in
https://github.com/Xiangjun-TJU/ULAMP-NET
.
更多查看译文
关键词
Lensless imaging,approximate message passing algorithm,deep unfolding method,spatial-channel attention
AI 理解论文
溯源树
样例
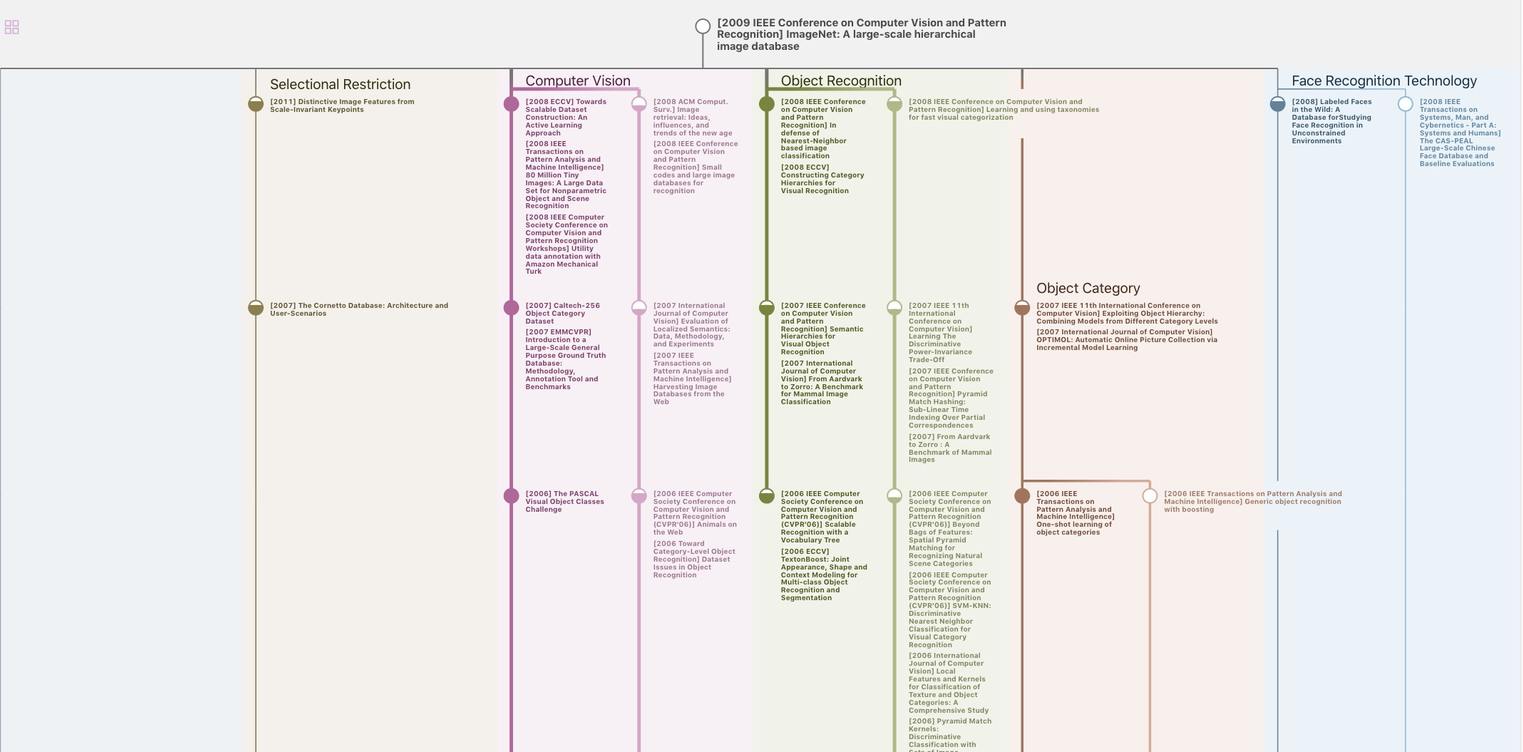
生成溯源树,研究论文发展脉络
Chat Paper
正在生成论文摘要