Photon-Counting CT Reconstruction With a Learned Forward Operator
IEEE Transactions on Computational Imaging(2022)
摘要
Photon-Counting CT is an emerging imaging technology that promises higher spatial resolution and the possibility for material decomposition in the reconstruction. A major difficulty in Photon-Counting CT is to efficiently model cross-talk between detectors. In this work, we accelerate image reconstruction tasks for Photon-Counting CT by modelling the cross-talk with an appropriately trained deep convolutional neural network. The main result relates to proving convergence when using such a learned cross-talk model in the context of second-order optimisation methods for spectral CT. Another is to evaluate the method through numerical experiments on small-scale CT acquisitions generated using a realistic physics model. Using the reconstruction with a full cross-talk model as ground truth, the learned cross-talk model results in a 20 dB increase in peak-signal-to noise ratio compared to ignoring cross-talk altogether. At the same time, it effectively cuts the computation time of the full cross-talk model in half. Furthermore, the learned cross-talk model generalises well to both unseen data and unseen detector settings. Our results indicate that such a partially learned forward operator is a suitable way of modelling data generation in Photon-Counting CT with a computational benefit that becomes more noticeable for realistic problem sizes.
更多查看译文
关键词
Photon-counting,computed tomography,spectral CT,regularisation,deep learning,detector cross-talk
AI 理解论文
溯源树
样例
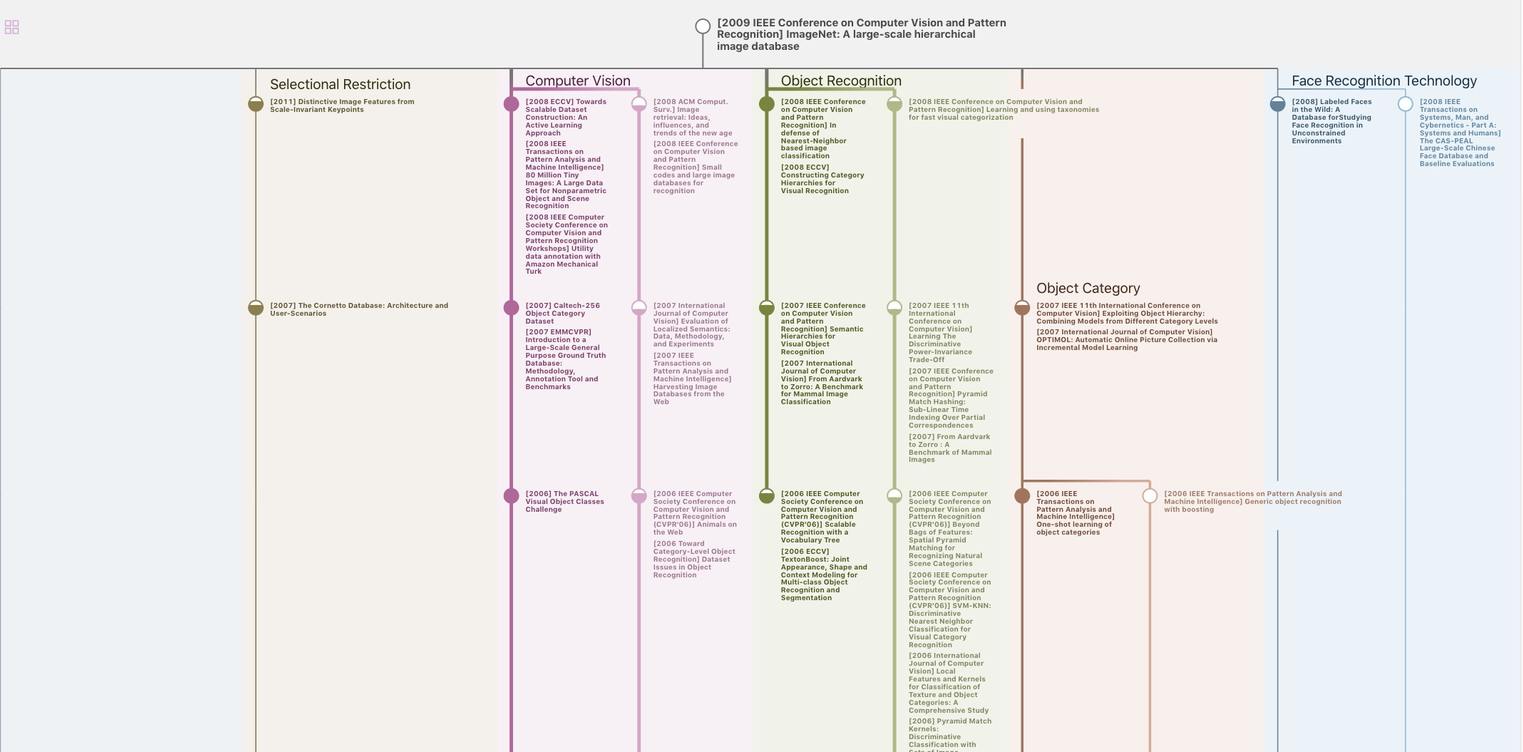
生成溯源树,研究论文发展脉络
Chat Paper
正在生成论文摘要